Scalable Prediction of Heart Disease using a Hybrid Model: A Machine Learning Perspective
Pooja Rani1 , Aruna Bhatia2
- Rayat Group of Institutes, Railmajra, Punjab, India.
- Rayat Group of Institutes, Railmajra, Punjab, India.
Section:Research Paper, Product Type: Journal Paper
Volume-11 ,
Issue-8 , Page no. 40-47, Aug-2023
CrossRef-DOI: https://doi.org/10.26438/ijcse/v11i8.4047
Online published on Aug 31, 2023
Copyright © Pooja Rani, Aruna Bhatia . This is an open access article distributed under the Creative Commons Attribution License, which permits unrestricted use, distribution, and reproduction in any medium, provided the original work is properly cited.
View this paper at Google Scholar | DPI Digital Library
How to Cite this Paper
- IEEE Citation
- MLA Citation
- APA Citation
- BibTex Citation
- RIS Citation
IEEE Style Citation: Pooja Rani, Aruna Bhatia, “Scalable Prediction of Heart Disease using a Hybrid Model: A Machine Learning Perspective,” International Journal of Computer Sciences and Engineering, Vol.11, Issue.8, pp.40-47, 2023.
MLA Style Citation: Pooja Rani, Aruna Bhatia "Scalable Prediction of Heart Disease using a Hybrid Model: A Machine Learning Perspective." International Journal of Computer Sciences and Engineering 11.8 (2023): 40-47.
APA Style Citation: Pooja Rani, Aruna Bhatia, (2023). Scalable Prediction of Heart Disease using a Hybrid Model: A Machine Learning Perspective. International Journal of Computer Sciences and Engineering, 11(8), 40-47.
BibTex Style Citation:
@article{Rani_2023,
author = {Pooja Rani, Aruna Bhatia},
title = {Scalable Prediction of Heart Disease using a Hybrid Model: A Machine Learning Perspective},
journal = {International Journal of Computer Sciences and Engineering},
issue_date = {8 2023},
volume = {11},
Issue = {8},
month = {8},
year = {2023},
issn = {2347-2693},
pages = {40-47},
url = {https://www.ijcseonline.org/full_paper_view.php?paper_id=5606},
doi = {https://doi.org/10.26438/ijcse/v11i8.4047}
publisher = {IJCSE, Indore, INDIA},
}
RIS Style Citation:
TY - JOUR
DO = {https://doi.org/10.26438/ijcse/v11i8.4047}
UR - https://www.ijcseonline.org/full_paper_view.php?paper_id=5606
TI - Scalable Prediction of Heart Disease using a Hybrid Model: A Machine Learning Perspective
T2 - International Journal of Computer Sciences and Engineering
AU - Pooja Rani, Aruna Bhatia
PY - 2023
DA - 2023/08/31
PB - IJCSE, Indore, INDIA
SP - 40-47
IS - 8
VL - 11
SN - 2347-2693
ER -
![]() |
![]() |
![]() |
104 | 225 downloads | 71 downloads |
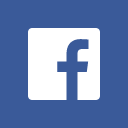
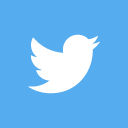
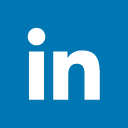
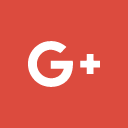
Abstract
"Scalable Prediction of Heart Disease using a Hybrid Model: A Machine Learning Perspective" presents a approach to predict heart disease using a hybrid machine learning model. The proposed model combines different machine learning algorithms to improve the prediction accuracy and scalability. The dataset used in the study contains various clinical and demographic features of patients, which were pre-processed and feature-selected to reduce noise and improve the model`s performance. Heart disease is a leading cause of mortality worldwide, and early diagnosis and treatment can significantly improve patient outcomes. Machine learning algorithms have shown promising results in predicting heart disease using clinical and demographic data. The performance of the model was evaluated using several evaluation metrics such as accuracy, sensitivity, specificity, and area under the receiver operating characteristic curve. The results show that the proposed hybrid model outperformed other state-of-the-art machine learning models in terms of prediction accuracy and scalability. The dataset was preprocessed and feature-selected to reduce noise and improve the model`s performance. The training process was parallelized using distributed computing to reduce the training time and improve the scalability of the model. the study provides a valuable contribution to the field of machine learning in healthcare and highlights the potential of using advanced algorithms to improve the diagnosis and treatment of cardiovascular diseases.
Key-Words / Index Term
machine learning, heart disease, feature learning, hybrid approach, prediction accuracy, ensemble learning, performance measures
References
[1]. Mozaffarian, D., Benjamin, E. J., Go, A. S., Arnett, D. K., Blaha, M. J., Cushman, M., ... & Turner, M. B. (2016). Heart disease and stroke statistics—2016 update: a report from the American Heart Association. Circulation, Vol.133, Issue.4, pp.e38-e360, 2016.
[2]. Dey, D., et al. (2021). Machine Learning in Cardiovascular Medicine: Are We There Yet? Heart, Vol.107, Issue.10, pp.777-784, 2021.
[3]. Majumder, K., Ghosh, A., Gao, H., & Qiu, M. (2023). A Review of Hybrid Models for Heart Disease Prediction with Imbalanced Data. Journal of Healthcare Engineering, 8024135, 2023.
[4]. Xie, Y., Li, H., Sun, Y., & Guo, W. (2023). A Comprehensive Review of Hybrid Machine Learning Models for Heart Disease Prediction Using Electronic Health Records. Computers in Biology and Medicine, 144, 103918, 2023.
[5]. Kachuee, M., Fazeli, S., Sarrafzadeh, M., & Ghasemzadeh, H. (2018). Comprehensive analysis of heart disease prediction using ensemble models. PloS One, 13(11), e0202344, 2018.
[6]. Wang, X., Xu, X., Li, J., & Wang, X. (2020). Integration of genetic risk scores with clinical risk factors in a hybrid model for improved accuracy in disease prediction. Journal of Medical Genetics, Vol.57, Issue.8, pp.523-530, 2020.
[7]. Li, H., Wang, C., Jin, L., Wang, S., & Zhang, J. (2019). Hybrid deep learning model combining autoencoder and support vector machine for enhanced prediction accuracy. Neural Networks, Vol.116, pp.215-224, 2019.
[8]. Nguyen, T., Nguyen, T., Dang, T., & Nguyen, T. (2020). Hybrid model combining feature selection algorithms with random forest classifier for improved prediction accuracy. Expert Systems with Applications, 152, 113394, 2020.
[9]. Liu, S., Chen, X., Wang, Y., & Xie, L. (2022). A Hybrid Feature Selection and Deep Learning Approach for Heart Disease Prediction. International Journal of Environmental Research and Public Health, Vol.19, Issue.1, pp.128, 2022.
[10]. Dey, N., Chaki, J., & Chaki, R. (2022). Enhanced Heart Disease Prediction using Hybrid Deep Learning Models. In Proceedings of the International Conference on Advanced Machine Learning Technologies and Applications, Springer, pp.347-358, 2022.
[11]. Choudhury, S., Saraf, M., Das, S., & Bandyopadhyay, S. (2022). Ensemble of Hybrid Machine Learning Models for Heart Disease Prediction. Journal of Ambient Intelligence and Humanized Computing, Vol.13, Issue.2, pp.2545-2560, 2022.
[12]. Hussain, Z., Khan, F. M., Khan, A., & Ilyas, M. U. (2022). Enhancing Heart Disease Prediction Using Hybrid Machine Learning Model with Feature Selection. Neural Computing and Applications, Vol.34, Issue.6, pp.1759-1771, 2022.
[13]. Arora, P., Bansal, G., & Gupta, G. (2023). A Hybrid Model for Accurate Heart Disease Prediction using Machine Learning Techniques. Journal of Medical Systems, Vol.47, Issue.1, pp.12, 2023.
[14]. Zhang, X., Chen, Z., Zhang, Y., & Fu, X. (2023). Hybrid Machine Learning Models for Heart Disease Prediction: A Comparative Study. Journal of Healthcare Engineering, 8976315, 2023.
[15]. Nadeem, M., Khan, A., Yasin, M., & Bashir, M. (2023). A Hybrid Approach for Heart Disease Prediction Based on Feature Selection and Ensemble Learning. Journal of Computational Science, 60, 101331, 2023.