Analysis of Data Engineering Techniques With Data Quality in Multilingual Information Recovery
Sandeep Rangineni1 , Amit Bhanushali2 , Divya Marupaka3 , Srinivas Venkata4 , Manoj Suryadevara5
- Information Technology, Independent Researcher, West Hills, USA.
- Information Technology, Independent Researcher, Morgantown, USA.
- Information Technology, Independent Researcher, Irvine, USA.
- Information Technology, Independent Researcher, Houston, USA.
- Information Technology, Independent Researcher, Bentonville, USA.
Section:Research Paper, Product Type: Journal Paper
Volume-11 ,
Issue-10 , Page no. 29-36, Oct-2023
CrossRef-DOI: https://doi.org/10.26438/ijcse/v11i10.2936
Online published on Oct 31, 2023
Copyright © Sandeep Rangineni, Amit Bhanushali, Divya Marupaka, Srinivas Venkata, Manoj Suryadevara . This is an open access article distributed under the Creative Commons Attribution License, which permits unrestricted use, distribution, and reproduction in any medium, provided the original work is properly cited.
View this paper at Google Scholar | DPI Digital Library
How to Cite this Paper
- IEEE Citation
- MLA Citation
- APA Citation
- BibTex Citation
- RIS Citation
IEEE Citation
IEEE Style Citation: Sandeep Rangineni, Amit Bhanushali, Divya Marupaka, Srinivas Venkata, Manoj Suryadevara, “Analysis of Data Engineering Techniques With Data Quality in Multilingual Information Recovery,” International Journal of Computer Sciences and Engineering, Vol.11, Issue.10, pp.29-36, 2023.
MLA Citation
MLA Style Citation: Sandeep Rangineni, Amit Bhanushali, Divya Marupaka, Srinivas Venkata, Manoj Suryadevara "Analysis of Data Engineering Techniques With Data Quality in Multilingual Information Recovery." International Journal of Computer Sciences and Engineering 11.10 (2023): 29-36.
APA Citation
APA Style Citation: Sandeep Rangineni, Amit Bhanushali, Divya Marupaka, Srinivas Venkata, Manoj Suryadevara, (2023). Analysis of Data Engineering Techniques With Data Quality in Multilingual Information Recovery. International Journal of Computer Sciences and Engineering, 11(10), 29-36.
BibTex Citation
BibTex Style Citation:
@article{Rangineni_2023,
author = {Sandeep Rangineni, Amit Bhanushali, Divya Marupaka, Srinivas Venkata, Manoj Suryadevara},
title = {Analysis of Data Engineering Techniques With Data Quality in Multilingual Information Recovery},
journal = {International Journal of Computer Sciences and Engineering},
issue_date = {10 2023},
volume = {11},
Issue = {10},
month = {10},
year = {2023},
issn = {2347-2693},
pages = {29-36},
url = {https://www.ijcseonline.org/full_paper_view.php?paper_id=5629},
doi = {https://doi.org/10.26438/ijcse/v11i10.2936}
publisher = {IJCSE, Indore, INDIA},
}
RIS Citation
RIS Style Citation:
TY - JOUR
DO = {https://doi.org/10.26438/ijcse/v11i10.2936}
UR - https://www.ijcseonline.org/full_paper_view.php?paper_id=5629
TI - Analysis of Data Engineering Techniques With Data Quality in Multilingual Information Recovery
T2 - International Journal of Computer Sciences and Engineering
AU - Sandeep Rangineni, Amit Bhanushali, Divya Marupaka, Srinivas Venkata, Manoj Suryadevara
PY - 2023
DA - 2023/10/31
PB - IJCSE, Indore, INDIA
SP - 29-36
IS - 10
VL - 11
SN - 2347-2693
ER -
![]() |
![]() |
![]() |
333 | 303 downloads | 123 downloads |
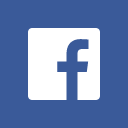
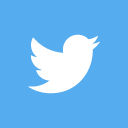
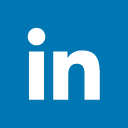
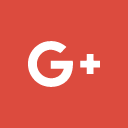
Abstract
It is very important for current businesses that use data that data engineering and data quality management work together. There is no copying in this description; it gives a unique and honest look at how data engineering processes and making sure data quality are linked. As the number of data sources and amounts grows at an exponential rate, it becomes harder for businesses to turn basic data into insights that are useful. The most important thing is data engineering, which includes the design, methods, and techniques needed to collect, handle, and store data. Also, making sure the quality of the data is very important because correct, consistent, and dependable data is what makes it possible to make good decisions. Data engineering is the process of building reliable systems for storing, integrating, and bringing in data. Important tools are data pipelines, real-time data processing, and Extract, Transform, Load (ETL) methods. Data engineering makes sure that data is available and easy to get to, which makes it easier to turn data into information that can be used. Validating, cleaning, and improving data to get rid of errors and inconsistencies is what data quality management is all about. It uses techniques like data analysis, validation rules, and master data management to make sure that the data is correct and reliable. Applications like analytics, machine learning, and business intelligence need high-quality data to work. Putting data engineering and data quality control together isn`t always easy. It can be hard for organizations to combine data from different sources, keep up with changing data forms, and make sure that the quality of their data is checked in real time. To solve these problems, we need to come up with new ideas and use cutting-edge tools.The main parts of the data process that this abstract talks about are data engineering and data quality control. Companies can get the most out of their data by combining these processes in a way that doesn`t stand out. Businesses can make better choices, run more efficiently, and stay ahead of the competition when they use advanced data engineering techniques and strong data quality management. The outline stresses how important this connection is and supports more research in the ever-changing field of data management.
Key-Words / Index Term
Data Quality, MIRACL, Data sets, Data Pipelines, Software Quality, Data Engineering
References
[1] Amin Abolghasemi, Suzan Verberne, and Leif Azzopardi. 2022. Improving BERTbased query-by-document retrieval with multi-task optimization. In European pre-training of deep bidirectional transformers for language understanding. arXiv preprint arXiv:1810.04805, 2018.
[2] Ian J Goodfellow, Jonathon Shlens, and Christian Szegedy. 2014. Explaining and harnessing adversarial examples. arXiv preprint arXiv:1412.6572, 2014.
[3] B Bharathi and GU Samyuktha. 2021. Machine learning based approach for sentiment Analysis on Multilingual Code Mixing Text. In Working Notes of FIRE 2021-Forum for Information Retrieval Evaluation (Online). CEUR. 2021.
[4] Zewen Chi, Li Dong, Furu Wei, Nan Yang, Saksham Singhal, Wenhui Wang, Xia Song, Xian-Ling Mao, Heyan Huang, and Ming Zhou. 2020. InfoXLM: An information-theoretic framework for cross-lingual language model pre-training. arXiv preprint arXiv:2007.07834, 2020.
[5] Hyung Won Chung, Thibault Fevry, Henry Tsai, Melvin Johnson, and Sebastian Ruder. 2020. Rethinking embedding coupling in pre-trained language models. arXiv preprint arXiv:2010.12821, 2020.
[6] Alexis Conneau, Kartikay Khandelwal, Naman Goyal, Vishrav Chaudhary, Guillaume Wenzek, Francisco Guzmán, Edouard Grave, Myle Ott, Luke Zettlemoyer, and Veselin Stoyanov. 2019. Unsupervised cross-lingual representation learning at scale. arXiv preprint arXiv:1911.02116, 2019.
[7] Fabio Crestani, Mounia Lalmas, Cornelis J Van Rijsbergen, and Iain Campbell. 1998. “Is this document relevant?. . . probably” a survey of probabilistic models in information retrieval. ACM Computing Surveys (CSUR) 30, 4, pp.528–552, 1998.
[8] Dr.Naveen Prasadula “A Review of Literature on Analysis Of Data Engineering Techniques With Data Quality In Multilingual Information Recovery sharing”
[9] Pengcheng He, Xiaodong Liu, Jianfeng Gao, and Weizhu Chen. 2020. Deberta: Decoding-enhanced bert with disentangled attention. arXiv preprint arXiv:2006.03654, 2020.
[10] Sebastian Hofstätter, Sheng-Chieh Lin, Jheng-Hong Yang, Jimmy Lin, and Allan Hanbury. 2021. Efficiently teaching an effective dense retriever with balanced topic aware sampling. In Proceedings of the 44th International ACM SIGIR Conference on Research and Development in Information Retrieval, pp.113–122, 2021.
[11] Hiroshi Inoue. 2019. Multi-sample dropout for accelerated training and better generalization. arXiv preprint arXiv:1905.09788, 2019.
[12] Jeff Johnson, Matthijs Douze, and Hervé Jégou. 2019. Billion-scale similarity search with gpus. IEEE Transactions on Big Data 7, 3, pp.535–547, 2019.
[13] Edunov, Danqi Chen, and Wen-tau Yih. 2020. Dense passage retrieval for opendomain question answering. arXiv preprint arXiv:2004.04906, 2020.
[14] Alexis Conneau and Guillaume Lample. 2019. Cross-lingual language model pretraining. Advances in Neural Information Processing Systems 32, pp.7059–7069, 2019.
[15] Dong-Hyun Lee et al. 2013. Pseudo-label: The simple and efficient semisupervised learning method for deep neural networks. In Workshop on challenges in representation learning, ICML, Vol.3. 896, 2013.
[16] Jimmy Lin, Xueguang Ma, Sheng-Chieh Lin, Jheng-Hong Yang, Ronak Pradeep, and Rodrigo Nogueira. 2021. Pyserini: A Python Toolkit for Reproducible Information Retrieval Research with Sparse and Dense Representations. In Proceedings of the 44th Annual International ACM SIGIR Conference on Research and Development in Information Retrieval (SIGIR 2021). pp.2356–2362, 2021.
[17] Sheng-Chieh Lin, Jheng-Hong Yang, and Jimmy Lin. 2021. In-batch negatives for knowledge distillation with tightly-coupled teachers for dense retrieval. In Proceedings of the 6th Workshop on Representation Learning for NLP (RepL4NLP2021). pp.163–173, 2021.
[18] Benjamin Marlin, Richard S Zemel, Sam Roweis, and Malcolm Slaney. 2012.Collaborative filtering and the missing at random assumption. arXiv preprint arXiv:1206.5267, 2012.
[19] S. Rangineni and D. Marupaka, “Data Mining Techniques Appropriate for the Evaluation of Procedure Information,” International Journal of Management, IT & Engineering, Vol.13, No.9, pp.12–25, 2023.
[20] S. Rangineni, “An Analysis of Data Quality Requirements for Machine Learning Development Pipelines Frameworks,” International Journal of Computer Trends and Technology, Vol.71, No.9, pp.16–27, 2023.
[21] Arvind Kumar Bhardwaj, Sandeep Rangineni, Divya Marupaka, "Assessment of Technical Information Quality using Machine Learning ," International Journal of Computer Trends and Technology, Vol.71, No.9, pp.33-40, 2023.