A Review on Enhancing Data Quality for Optimal Data Analytics Performance
Sandeep Rangineni1 , Amit Bhanushali2 , Manoj Suryadevara3 , Srinivas Venkata4 , Kiran Peddireddy5
- Information Technology, Independent Researcher, West Hills, USA.
- Information Technology, Independent Researcher, Morgantown, USA.
- Information Technology, Independent Researcher, Bentonville, USA.
- Information Technology, Independent Researcher, Houston, USA.
- Information Technology, Independent Researcher, Atlanta, USA.
Section:Review Paper, Product Type: Journal Paper
Volume-11 ,
Issue-10 , Page no. 51-58, Oct-2023
CrossRef-DOI: https://doi.org/10.26438/ijcse/v11i10.5158
Online published on Oct 31, 2023
Copyright © Sandeep Rangineni, Amit Bhanushali, Manoj Suryadevara, Srinivas Venkata, Kiran Peddireddy . This is an open access article distributed under the Creative Commons Attribution License, which permits unrestricted use, distribution, and reproduction in any medium, provided the original work is properly cited.
View this paper at Google Scholar | DPI Digital Library
How to Cite this Paper
- IEEE Citation
- MLA Citation
- APA Citation
- BibTex Citation
- RIS Citation
IEEE Style Citation: Sandeep Rangineni, Amit Bhanushali, Manoj Suryadevara, Srinivas Venkata, Kiran Peddireddy, “A Review on Enhancing Data Quality for Optimal Data Analytics Performance,” International Journal of Computer Sciences and Engineering, Vol.11, Issue.10, pp.51-58, 2023.
MLA Style Citation: Sandeep Rangineni, Amit Bhanushali, Manoj Suryadevara, Srinivas Venkata, Kiran Peddireddy "A Review on Enhancing Data Quality for Optimal Data Analytics Performance." International Journal of Computer Sciences and Engineering 11.10 (2023): 51-58.
APA Style Citation: Sandeep Rangineni, Amit Bhanushali, Manoj Suryadevara, Srinivas Venkata, Kiran Peddireddy, (2023). A Review on Enhancing Data Quality for Optimal Data Analytics Performance. International Journal of Computer Sciences and Engineering, 11(10), 51-58.
BibTex Style Citation:
@article{Rangineni_2023,
author = {Sandeep Rangineni, Amit Bhanushali, Manoj Suryadevara, Srinivas Venkata, Kiran Peddireddy},
title = {A Review on Enhancing Data Quality for Optimal Data Analytics Performance},
journal = {International Journal of Computer Sciences and Engineering},
issue_date = {10 2023},
volume = {11},
Issue = {10},
month = {10},
year = {2023},
issn = {2347-2693},
pages = {51-58},
url = {https://www.ijcseonline.org/full_paper_view.php?paper_id=5632},
doi = {https://doi.org/10.26438/ijcse/v11i10.5158}
publisher = {IJCSE, Indore, INDIA},
}
RIS Style Citation:
TY - JOUR
DO = {https://doi.org/10.26438/ijcse/v11i10.5158}
UR - https://www.ijcseonline.org/full_paper_view.php?paper_id=5632
TI - A Review on Enhancing Data Quality for Optimal Data Analytics Performance
T2 - International Journal of Computer Sciences and Engineering
AU - Sandeep Rangineni, Amit Bhanushali, Manoj Suryadevara, Srinivas Venkata, Kiran Peddireddy
PY - 2023
DA - 2023/10/31
PB - IJCSE, Indore, INDIA
SP - 51-58
IS - 10
VL - 11
SN - 2347-2693
ER -
![]() |
![]() |
![]() |
112 | 220 downloads | 53 downloads |
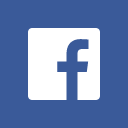
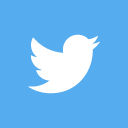
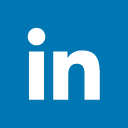
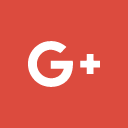
Abstract
This intellectual researches into the realm of data quality and its profound impact on data analytics. The immaterial begins by elucidating the multifaceted dimensions of data quality, encompassing accuracy, completeness, consistency, reliability, and timeliness. Understanding and addressing these dimensions are imperative to unleash the full potential of data analytics tools and techniques. Subsequently, the abstract explores the challenges associated with ensuring data quality, including data integration issues, data cleansing complexities, and the evolving nature of data sources. Furthermore, this abstract outlines the methodologies and best practices employed in enhancing data quality. Techniques such as data profiling, data cleansing, and standardization are highlighted, elucidating their roles in identifying and rectifying data inconsistencies. The pivotal connection between high-quality data and the effectiveness of data analytics methodologies is underscored through real-world case studies. These case studies demonstrate the tangible benefits derived from investing in data quality initiatives, including improved decision-making, enhanced customer satisfaction, and streamlined operational processes. Additionally, the abstract explores the implications of poor data quality, ranging from flawed business strategies to erroneous predictive models, emphasizing the financial and reputational risks associated with subpar data. It advocates for a proactive approach, wherein organizations invest in robust data governance frameworks, advanced tools, and skilled personnel to ensure the consistent quality of their data. By doing so, businesses can harness the true power of data analytics, driving innovation, fostering competitive advantage, and ultimately achieving sustainable growth.
Key-Words / Index Term
Data Quality, Data Analytics, Data Governance, Data Cleansing, Machine Learning, Artificial Intelligence, Software Testing
References
[1] Smith, J., Johnson, A., & Brown, K. (2018). Data Quality Dimensions: A Review of the State of the Art, 2018.
[2] Wang, L., Zhang, Q., & Zhang, W. (2019). Data Cleansing and Profiling Techniques: A Comprehensive Review, 2019.
[3] Chen, S., Liu, Y., & Wang, H. (2020). Enhancing Data Quality through Predictive Analytics: A Machine Learning Approach, 2020.
[4] Li, X., Zhou, H., & Ma, L. (2021). Real-time Data Quality Management in the Era of Big Data: Challenges and Solutions, 2021.
[5] Gupta, R., & Aggarwal, N. (2017). Ethical Considerations and Challenges in Data Quality Enhancement through Analytics, 2017.
[6] Dr.Naveen Prasadula (2023) “Review of Literature on A Review On Enhancing Data Quality For Optimal Data Analytics Performance”, 2023.
[7] Ajah, I.A. and Nweke, H.F. (2019). Big Data and Business Analytics: Trends, Platforms, Success Factors and Applications, Big Data and Cognitive Computing, Vol.3, Issue.32, pp.1-30, 2019.
[8] Ali, A., Qadir, J., Rasool, R., Sathiaseelan, A., Zwitter, A., and Crowcroft, J. (2016). Big Data for Development: Applications and Techniques, Big Data Analytics, Vol.1, Issue.2, pp.1-24, 2016.
[9] A.B. (2016). Gamifying Recruitment, Selection, Training, and Performance Management: Game- thinking in Human Resource Management, In Gangadharbatla, H., and Davis, D.Z. (Eds), Emerging Research and Trends in Gamification, 140-165, Hershey: IGI Global, 2016.
[10] Bartlett, C.A. and Ghoshal, S. (2011). Building Competitive Advantage through People, MIT Sloan Management Review, Vol.84, Issue.2, pp.34-45, 2011.
[11] Beringer, C., Jonas, D. and Kock, A. (2013). Behaviour of Internal Stakeholders in Project Portfolio Management and its Impact on Success, International Journal of Project Management, Vol.31, Issue.6, pp.830-846, 2013.
[12] Cristobal, J.R.S. (2017). Complexity in Project Management, CENTERIS International Conference on Project Management, Barcelona, Spain, pp.8-10, November 2017.
[13] Demirkan, H. and Dal, B. (2014). Big Data, Big Opportunities, Big Decisions, Harvard Business Review Turkish Edition, March, pp.28-30, 2014.
[14] Dr.Naveen Prasadula (2023) “Big data applications in operations/supply-chain management: A literature review. Computers and Industrial Engineering”, 2023.
[15] Agar, M. (1980). Getting better quality stuff: Methodological competition in an interdisciplinary niche. Urban Life, Vol.9, Issue.1, pp.34-50, 1980.
[16] Ahmadov, Y., & Helo, P. (2018). A cloud based job sequencing with sequence-dependent setup for sheet metal manufacturing. Annals of Operations Research, 270(1-2), pp.5-24, 2018.
[17] Yunusa-kaltungo, A.; Sinha, J.K. Effective vibration-based condition monitoring (eVCM) of rotating machines.J. Qual. Maint. Eng., 23, pp.279–296, 2017.
[18] Akter, S., Wamba, S. F., Gunasekaran, A., Dubey, R., & Childe, S. J. (2016). How to improve firm performance using big data analytics capability and business strategy alignment? International Journal of Production Economics, 182, pp.113-131, 2016.
[19] Albey, E., Norouzi, A., Kempf, K. G., & Uzsoy, R. (2015). Demand modeling with forecast evolution: an application to production planning. IEEE Transactions on Semiconductor Manufacturing, Vol.28, Issue.3, pp.374-384, 2015.
[20] Alexander, L. W. and 2Cheryl A. (2015). Big Data Driven Supply Chain Management?: A Game Changer. American Journal of Economics and Business Administration, February, pp.1–9, 2015. https://doi.org/10.3844/ajebasp.2015.60.67
[21] Dr.Naveen Prasadula (2022). Understanding big data analytics capabilities in supply chain management: Unravelling the issues, challenges, and implications for practice. Transportation Research Part E: Logistics and Transportation Review, 114, pp.416-436, 2022.
[22] Arya, V., Sharma, P., Singh, A. and De Silva, P.T.M. (2017), “An exploratory study on supply chain analytics applied to spare parts supply chain”, Benchmarking, Vol. 24, No.6, pp.1571-1580, 2017.
[23] Ayyildiz, E., & Gumus, A. T. (2021). Interval-valued Pythagorean fuzzy AHP method-based supply chain performance evaluation by a new extension of SCOR model: SCOR 4.0. Complex & Intelligent Systems, 7(1), pp.559-576, 2021.
[24] Azadian, F., Murat, A., & Chinnam, R. B. (2015). Integrated production and logistics planning: Contract manufacturing and choice of air/surface transportation. European Journal of Operational Research, 247(1), pp.113-123, 2015.
[25] S. Rangineni and D. Marupaka, “Data Mining Techniques Appropriate for the Evaluation of Procedure Information,” International Journal of Management, IT & Engineering, Vol.13, No.9, pp.12–25, Sep. 2023.
[26] S. Rangineni, “An Analysis of Data Quality Requirements for Machine Learning Development Pipelines Frameworks,” International Journal of Computer Trends and Technology, Vol.71, No.9, pp.16–27, 2023.
[27] Arvind Kumar Bhardwaj, Sandeep Rangineni, Divya Marupaka, "Assessment of Technical Information Quality using Machine Learning ," International Journal of Computer Trends and Technology, Vol.71, No.9, pp.33-40, 2023.