Evaluating VGG-16 Performance in Brain Tumor Detection: A Comprehensive Review
Sajid Faysal Fahim1 , Nayem Mollah2 , Nusrat Sultana3 , Md. Mohshiu Islam Khan4
- Dept. of Computer Science and Engineering, East West University, Dhaka, Bangladesh.
- Dept. of Computer Science and Engineering, East West University, Dhaka, Bangladesh.
- Dept. of Computer Science and Engineering, East West University, Dhaka, Bangladesh.
Section:Research Paper, Product Type: Journal Paper
Volume-11 ,
Issue-10 , Page no. 59-63, Oct-2023
CrossRef-DOI: https://doi.org/10.26438/ijcse/v11i10.5963
Online published on Oct 31, 2023
Copyright © Sajid Faysal Fahim, Nayem Mollah, Nusrat Sultana, Md. Mohshiu Islam Khan . This is an open access article distributed under the Creative Commons Attribution License, which permits unrestricted use, distribution, and reproduction in any medium, provided the original work is properly cited.
View this paper at Google Scholar | DPI Digital Library
How to Cite this Paper
- IEEE Citation
- MLA Citation
- APA Citation
- BibTex Citation
- RIS Citation
IEEE Style Citation: Sajid Faysal Fahim, Nayem Mollah, Nusrat Sultana, Md. Mohshiu Islam Khan, “Evaluating VGG-16 Performance in Brain Tumor Detection: A Comprehensive Review,” International Journal of Computer Sciences and Engineering, Vol.11, Issue.10, pp.59-63, 2023.
MLA Style Citation: Sajid Faysal Fahim, Nayem Mollah, Nusrat Sultana, Md. Mohshiu Islam Khan "Evaluating VGG-16 Performance in Brain Tumor Detection: A Comprehensive Review." International Journal of Computer Sciences and Engineering 11.10 (2023): 59-63.
APA Style Citation: Sajid Faysal Fahim, Nayem Mollah, Nusrat Sultana, Md. Mohshiu Islam Khan, (2023). Evaluating VGG-16 Performance in Brain Tumor Detection: A Comprehensive Review. International Journal of Computer Sciences and Engineering, 11(10), 59-63.
BibTex Style Citation:
@article{Fahim_2023,
author = {Sajid Faysal Fahim, Nayem Mollah, Nusrat Sultana, Md. Mohshiu Islam Khan},
title = {Evaluating VGG-16 Performance in Brain Tumor Detection: A Comprehensive Review},
journal = {International Journal of Computer Sciences and Engineering},
issue_date = {10 2023},
volume = {11},
Issue = {10},
month = {10},
year = {2023},
issn = {2347-2693},
pages = {59-63},
url = {https://www.ijcseonline.org/full_paper_view.php?paper_id=5633},
doi = {https://doi.org/10.26438/ijcse/v11i10.5963}
publisher = {IJCSE, Indore, INDIA},
}
RIS Style Citation:
TY - JOUR
DO = {https://doi.org/10.26438/ijcse/v11i10.5963}
UR - https://www.ijcseonline.org/full_paper_view.php?paper_id=5633
TI - Evaluating VGG-16 Performance in Brain Tumor Detection: A Comprehensive Review
T2 - International Journal of Computer Sciences and Engineering
AU - Sajid Faysal Fahim, Nayem Mollah, Nusrat Sultana, Md. Mohshiu Islam Khan
PY - 2023
DA - 2023/10/31
PB - IJCSE, Indore, INDIA
SP - 59-63
IS - 10
VL - 11
SN - 2347-2693
ER -
![]() |
![]() |
![]() |
107 | 204 downloads | 70 downloads |
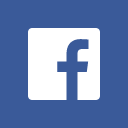
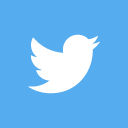
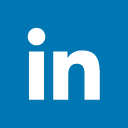
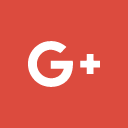
Abstract
This comprehensive examination deeply explores the evaluation of the VGG-16 architecture in the critical and significant domain of brain tumor detection, which holds utmost importance in the field of medical image analysis. The study meticulously and thoroughly evaluates the strengths and weaknesses of the VGG-16 model, taking into account its pivotal role as a deep learning model specifically crafted for this crucial medical application A comprehensive and meticulous evaluation is conducted to offer a thorough and all-encompassing assessment of the effectiveness of VGG-16 in precisely identifying brain tumors. This entails a meticulous and detailed exploration of diverse datasets, methodologies, and benchmarking metrics. The significant findings obtained from this extensive analysis shed crucial light on the immense potential of the VGG-16 model in the field of brain tumor detection, while also highlighting its inherent limitations and areas that could be enhanced. These invaluable observations have been demonstrated to be extremely advantageous for both individuals conducting research and professionals working in the field of medical image analysis. It is of utmost significance to acknowledge that this analysis ultimately underscores the crucial significance of continuous research initiatives directed towards enhancing the efficacy of VGG-16 specifically in the domain of brain tumor detection. The ultimate objective of these endeavors is to formulate healthcare solutions that are more precise and efficient, thereby greatly benefiting patients requiring such interventions.
Key-Words / Index Term
Brain diseases, Proposed Method, Artificial Neural Networks, Tumor, Necrosis, Anisotropic Diffusion
References
[1] N Van . Porz, "Multi-modalodal glioblastoma segmentation: Man versus machine", PLOS ONE, Vol.9, pp.e96873, 2014.
[2]J.L. Marroquin, B.C. Vemuri, S. Botello and F. Calderon, ?An accurate and efficient Bayesian method for automatic segmentation of brain MRI,? Proceedings of the 7th European Conference on Computer Vision, London, UK, August 2002.
[3] M.G DiBono and M. Zorzi, ?Decoding cognitive states from fMRI data using support vector regression,? Psychology Journal, 2008.
[4] S. Bauer, R. Wiest, L.-P. Nolte and M. Reyes, "A survey of MRI based medical image analysis for brain tumor studies", Phys. Med. Biol., Vol.58, No.13, pp.R97-R129, 2013.
[5] Z. Shi, L. He, T.N.K Suzuki, and H. Itoh, ?Survey on Neural Networks used for Medical Image Processing,? International Journal of Computational Science, 2009.
[6] V.B Padole and D.S. Chaudhari, ?Detection of Brain Tumor in MRI Images Using Mean Shift Algorithm and Normalized Cut Method,? International Journal of Engineering and Advanced Technology, June 2012.
[7] L. Weizman, "Automatic segmentation, internal classification, and follow-up of optic pathway gliomas in MRI", Med. Image Anal., Vol.16, no.1, pp.177-188, 2012.
[8].Meenakshi, R & Anandhakumar, P 2012, ?Brain Tumor identification in MRI with BPN Classifier and Orthonormal Operators‘, European Journal of Scientific Research, Vol.85, no.4, pp.559-569, 2012.
[9] Manoj K Kowear and Sourabh Yadev, “Brain tumor detection and segmentation using histogram thresholding”, International Journal of engineering and Advanced Technology, April 2012.
[10] Rajesh C. patil, A.S. Bhalchandra, “Brain tumor extraction from MRI images Using MAT Lab”, IJECSCSE, ISSN: 2277-9477, Vol.2, Issue.1, pp.1-4, 2012.
[11] Vinay Parmeshwarappa, Nandish S, “A segmented morphological approach to detect tumor in brain images”, IJARCSSE, ISSN: 2277 128X , Vol.4, Issue.1, January 2014.