Human-in-the-Loop Techniques: The Game-Changer in Facial Recognition
Tharun Anand Reddy Sure1
- Department of Software Engineering, ServiceNow, Santa Clara, California, USA.
Section:Research Paper, Product Type: Journal Paper
Volume-11 ,
Issue-11 , Page no. 1-4, Nov-2023
CrossRef-DOI: https://doi.org/10.26438/ijcse/v11i11.14
Online published on Nov 30, 2023
Copyright © Tharun Anand Reddy Sure . This is an open access article distributed under the Creative Commons Attribution License, which permits unrestricted use, distribution, and reproduction in any medium, provided the original work is properly cited.
View this paper at Google Scholar | DPI Digital Library
How to Cite this Paper
- IEEE Citation
- MLA Citation
- APA Citation
- BibTex Citation
- RIS Citation
IEEE Style Citation: Tharun Anand Reddy Sure, “Human-in-the-Loop Techniques: The Game-Changer in Facial Recognition,” International Journal of Computer Sciences and Engineering, Vol.11, Issue.11, pp.1-4, 2023.
MLA Style Citation: Tharun Anand Reddy Sure "Human-in-the-Loop Techniques: The Game-Changer in Facial Recognition." International Journal of Computer Sciences and Engineering 11.11 (2023): 1-4.
APA Style Citation: Tharun Anand Reddy Sure, (2023). Human-in-the-Loop Techniques: The Game-Changer in Facial Recognition. International Journal of Computer Sciences and Engineering, 11(11), 1-4.
BibTex Style Citation:
@article{Sure_2023,
author = {Tharun Anand Reddy Sure},
title = {Human-in-the-Loop Techniques: The Game-Changer in Facial Recognition},
journal = {International Journal of Computer Sciences and Engineering},
issue_date = {11 2023},
volume = {11},
Issue = {11},
month = {11},
year = {2023},
issn = {2347-2693},
pages = {1-4},
url = {https://www.ijcseonline.org/full_paper_view.php?paper_id=5635},
doi = {https://doi.org/10.26438/ijcse/v11i11.14}
publisher = {IJCSE, Indore, INDIA},
}
RIS Style Citation:
TY - JOUR
DO = {https://doi.org/10.26438/ijcse/v11i11.14}
UR - https://www.ijcseonline.org/full_paper_view.php?paper_id=5635
TI - Human-in-the-Loop Techniques: The Game-Changer in Facial Recognition
T2 - International Journal of Computer Sciences and Engineering
AU - Tharun Anand Reddy Sure
PY - 2023
DA - 2023/11/30
PB - IJCSE, Indore, INDIA
SP - 1-4
IS - 11
VL - 11
SN - 2347-2693
ER -
![]() |
![]() |
![]() |
151 | 157 downloads | 59 downloads |
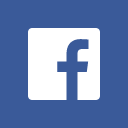
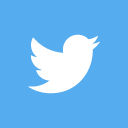
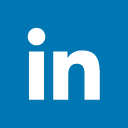
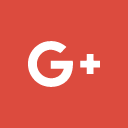
Abstract
Facial recognition technology has become increasingly ubiquitous, being used for everything from unlocking smartphones to identifying individuals. This technology has made our lives easier and more efficient in many ways, but it still has limitations. One of the most significant challenges facial recognition systems face is their accuracy, particularly for underrepresented demographic groups. This issue is further complicated by biases in the datasets used to train these systems. Facial recognition that produces inaccurate results can lead to severe consequences, including wrongful arrests and false accusations. Such technology must be thoroughly tested and regulated to avoid harming innocent individuals. Researchers have explored various methods for improving facial recognition algorithms to address these challenges. One promising approach is to leverage human expertise to supplement the machine-learning process. This article reviews some of the most effective human-in-the-loop approaches to enhance facial recognition systems` fairness, interpretability, and performance. These methods involve incorporating human feedback at different stages of the machine-learning pipeline, such as active learning, clean labeling, and human-AI collaboration in model development and evaluation. The results of these studies have shown that incorporating human judgment and domain knowledge can significantly improve facial recognition systems` accuracy and fairness. For example, active learning methods can help mitigate dataset biases by prioritizing the most informative samples for human labeling. Clean labeling can help ensure the training data is accurate and unbiased, while human-AI collaboration can improve model interpretability and generalization. The significance of these findings is that thoughtfully integrating human expertise into the facial recognition process can lead to more ethical and robust systems. By involving human feedback, we can mitigate the biases and limitations of machine learning algorithms and ensure that these technologies work for everyone, regardless of race, gender, or other demographic factors. Ultimately, this will help us build a more just and equitable society.
Key-Words / Index Term
facial recognition, human-in-the-loop, active learning, clean labeling, model interpretation, fairness
References
[1] Al-Rahayfeh, A., & Faezipour, M., “Eye Tracking and Head Movement Detection: A State-of-Art Survey”. IEEE Journal of Translational Engineering in Health and Medicine, Vol.1, 2013.
[2] Buolamwini, J., & Gebru, T., “Gender Shades: Intersectional Accuracy Disparities in Commercial Gender Classification”. Proceedings of Machine Learning Research, Vol.81, pp.1-15, 2018.
[3] Krishnapriya, K.S., Vangara, K., King, M.C. et al, “Characterizing demographic bias in actionable attributes”. Nature Machine Intelligence, Vol.4, pp.577–589, 2022.
[4] Tan, X. and Triggs, B., “Enhanced local texture feature sets for face recognition under difficult lighting conditions”. IEEE transactions on image processing, vol.19, Issue.6, pp.1635-1650, 2010.
[5] D. Mahapatra et al., “Efficient crowd sourcing based annotation of video data”. Pattern Recognition Letters, Vol.132, pp.83-90, 2020.
[6] A. Mishra et al., “Assessing image abstraction and person recognition skills using games with non-expert contributors”. Frontiers in ICT, Vol.4, p.27, 2017.
[7] A. Srivastava et al., “Structured annotation for facial recognition in real-world conditions”. In 2019 International Conference on Computer Vision Workshop (ICCVW) IEEE, pp. 2702-2710, 2019.
[8] J. Whitehill et al., “Whose vote should count more: Optimal integration of labels from labelers of unknown expertise”. Advances in neural information processing systems, Vol.22, 2009.
[9] E. Agustsson et al., “Recursive stochastic processes for face aging”. In Proceedings of the IEEE International Conference on Computer Vision, pp.231-240, 2017.
[10] Krishnapriya, K.S., Vangara, K., King, M.C. et al., “Characterizing demographic bias in actionable attributes”. Nature Machine Intelligence, Vol.4, pp.577–589, 2022.
[11] S. Lumini, L. Nanni, “Overview of the combination of biometric matchers”. Information Fusion, Vol.40, pp.1-10, 2017.
[12] Q. Cao et al., “Interpretable convolutional neural networks via feedforward design”. Journal of Visual Communication and Image Representation, Vol.56, pp.346-359, 2018.
[13] A. Krizhevsky et al., “ImageNet classification with deep convolutional neural networks”. Communications of the ACM, Vol.60, Issue.6, pp.84-90, 2017.
[14] Y. Taigman et al, “Deepface: Closing the gap to human-level performance in face verification”. In Proceedings of the IEEE conference on computer vision and pattern recognition, pp.1701-1708, 2014.
[15] Chouldechova, A., “Fair prediction with disparate impact: A study of bias in recidivism prediction instruments”. Big data, Vol.5, Issue.2, pp.153-163, 2017.
[16] J.T. Barron, “A general and adaptive robust loss function”. In Proceedings of the IEEE/CVF Conference on Computer Vision and Pattern Recognition, pp.4331-4339, 2019.
[17] D. Güera, E.J. Delp, “Deepfake video detection using recurrent neural networks”. In 2018 15th IEEE International Conference on Advanced Video and Signal Based Surveillance (AVSS), pp.1-6, 2018.
[18] T. Karras et al., “Training generative adversarial networks with limited data”. Advances in Neural Information Processing Systems, Vol.33, pp.12104-12114, 2020.
[19] A. Bothe et al., “Enabling facial retargeting in visual dubbing”. In Computer Graphics Forum, Vol.38, Issue.2, pp.379-390, 2019.
[20] J. Yan et al., “Human-in-the-Loop Sketch-Based Image Synthesis”. In Proceedings of the IEEE/CVF International Conference on Computer Vision, pp.7172-7182, 2021.
[21] H. Qin et al., “Hierarchical face clustering on deep features for movie character recognition”. IEEE Transactions on Image Processing, Vol.30, pp.347-362, 2020.
[22] X. Liu et al., “Crowdsourcing annotations for visual recognition”. 2021 IEEE/CVF Conference on Computer Vision and Pattern Recognition (CVPR), IEEE, pp.15576-15585, 2021.
[23] Das, A., Agrawal, H., Zitnick, C.L., Parikh, D. and Batra, D., “Human attention in visual question answering: Do humans and deep networks look at the same regions?”. Computer Vision and Image Understanding, Vol.163, pp.90-100, 2017.
[24] Lu, C., Tang, X., “Surpassing Human-Level Face Verification Performance on LFW with GaussianFace”. Proceedings of the AAAI Conference on Artificial Intelligence, Vol.28, Issue.1, 2014.
[25] K. Liang et al., “Gt-net: Interactive machine teaching with knowledge graph for improving facial action unit recognition”. In Proceedings of the IEEE/CVF International Conference on Computer Vision, pp.1402-1411, 2021.