Hydrological Drought Forecasting, using Artificial Neural Network (ANN) and Predict Values of Hydrological Drought Condition Derived using River Water Level Data
Rajesh Kumar Sharma1 , Mayank Rajput2 , Rahul Sharma3
- A-51 Exotica Villas Airport Road Bhopal (M.P), India.
- Director. Alpha College, NH 46 Bairagarh Khuman, Dist Sehore (M.P), India.
- A-51 Exotica Villas Airport Road Bhopal (M.P), India.
Section:Research Paper, Product Type: Journal Paper
Volume-11 ,
Issue-10 , Page no. 71-74, Oct-2023
CrossRef-DOI: https://doi.org/10.26438/ijcse/v11i10.7174
Online published on Oct 31, 2023
Copyright © Rajesh Kumar Sharma, Mayank Rajput, Rahul Sharma . This is an open access article distributed under the Creative Commons Attribution License, which permits unrestricted use, distribution, and reproduction in any medium, provided the original work is properly cited.
View this paper at Google Scholar | DPI Digital Library
How to Cite this Paper
- IEEE Citation
- MLA Citation
- APA Citation
- BibTex Citation
- RIS Citation
IEEE Style Citation: Rajesh Kumar Sharma, Mayank Rajput, Rahul Sharma, “Hydrological Drought Forecasting, using Artificial Neural Network (ANN) and Predict Values of Hydrological Drought Condition Derived using River Water Level Data,” International Journal of Computer Sciences and Engineering, Vol.11, Issue.10, pp.71-74, 2023.
MLA Style Citation: Rajesh Kumar Sharma, Mayank Rajput, Rahul Sharma "Hydrological Drought Forecasting, using Artificial Neural Network (ANN) and Predict Values of Hydrological Drought Condition Derived using River Water Level Data." International Journal of Computer Sciences and Engineering 11.10 (2023): 71-74.
APA Style Citation: Rajesh Kumar Sharma, Mayank Rajput, Rahul Sharma, (2023). Hydrological Drought Forecasting, using Artificial Neural Network (ANN) and Predict Values of Hydrological Drought Condition Derived using River Water Level Data. International Journal of Computer Sciences and Engineering, 11(10), 71-74.
BibTex Style Citation:
@article{Sharma_2023,
author = {Rajesh Kumar Sharma, Mayank Rajput, Rahul Sharma},
title = {Hydrological Drought Forecasting, using Artificial Neural Network (ANN) and Predict Values of Hydrological Drought Condition Derived using River Water Level Data},
journal = {International Journal of Computer Sciences and Engineering},
issue_date = {10 2023},
volume = {11},
Issue = {10},
month = {10},
year = {2023},
issn = {2347-2693},
pages = {71-74},
url = {https://www.ijcseonline.org/full_paper_view.php?paper_id=5654},
doi = {https://doi.org/10.26438/ijcse/v11i10.7174}
publisher = {IJCSE, Indore, INDIA},
}
RIS Style Citation:
TY - JOUR
DO = {https://doi.org/10.26438/ijcse/v11i10.7174}
UR - https://www.ijcseonline.org/full_paper_view.php?paper_id=5654
TI - Hydrological Drought Forecasting, using Artificial Neural Network (ANN) and Predict Values of Hydrological Drought Condition Derived using River Water Level Data
T2 - International Journal of Computer Sciences and Engineering
AU - Rajesh Kumar Sharma, Mayank Rajput, Rahul Sharma
PY - 2023
DA - 2023/10/31
PB - IJCSE, Indore, INDIA
SP - 71-74
IS - 10
VL - 11
SN - 2347-2693
ER -
![]() |
![]() |
![]() |
84 | 78 downloads | 43 downloads |
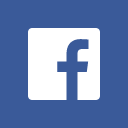
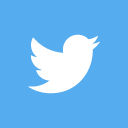
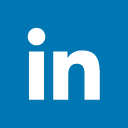
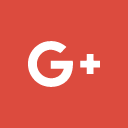
Abstract
This paper focuses on hydrological drought forecasting, using Artificial Neural Network (ANN) and predicts the values of hydrological drought condition derived using Narmada River Water level data of Hoshangabad (M.P). We have used the water level data as input data of ANN model for hydrological drought forecasting, and determine Standardized Water Level Index (SWLI). Artificial Neural networks operate on the principle of learning from a training set. There is a large variety of neural network models and learning procedures. Two classes of neural networks that are usually used for prediction applications are feed-forward networks and recurrent networks. They often train both of these networks using back-propagation algorithm.
Key-Words / Index Term
Artificial Neural Network, Hydrological Drought, RWL.
References
[1] Agnew, C. T.: Using the SPI to identify drought. Drought Network News, Vol.12, Issue.1, pp.6–11, 1999.
[2] Bankert, R. L.: Cloud classification of AVHRR Imagery in maritime regions using a probabilistic neural network, J. Appl. Meteorol., Vol.33, pp.909–918, 1994.
[3] Marzban, C. and Stumpf, G. J.: A neural network for tornado prediction based on Doppler radar-derived attributes. J. Appl. Meteor., Vol.35, pp.617–626, 1996.
[4] Mu¨ller, B., and Reinhardt, J.: Neural Networks: An Introduction, the Physics of Neural Networks Series, Springer-Verlag, Vol.2, pp 266, 1991.