A YOLO-Powered Deep Learning Approach to Psoriasis Classification
Anushree Goswami1 , Nidhi Sharma2
- School of Biotechnology and Bioinformatics, D Y Patil Deemed to be University, Navi Mumbai, India.
- School of Biotechnology and Bioinformatics, D Y Patil Deemed to be University, Navi Mumbai, India.
Section:Research Paper, Product Type: Journal Paper
Volume-12 ,
Issue-1 , Page no. 1-7, Jan-2024
CrossRef-DOI: https://doi.org/10.26438/ijcse/v12i1.17
Online published on Jan 31, 2024
Copyright © Anushree Goswami, Nidhi Sharma . This is an open access article distributed under the Creative Commons Attribution License, which permits unrestricted use, distribution, and reproduction in any medium, provided the original work is properly cited.
View this paper at Google Scholar | DPI Digital Library
How to Cite this Paper
- IEEE Citation
- MLA Citation
- APA Citation
- BibTex Citation
- RIS Citation
IEEE Style Citation: Anushree Goswami, Nidhi Sharma, “A YOLO-Powered Deep Learning Approach to Psoriasis Classification,” International Journal of Computer Sciences and Engineering, Vol.12, Issue.1, pp.1-7, 2024.
MLA Style Citation: Anushree Goswami, Nidhi Sharma "A YOLO-Powered Deep Learning Approach to Psoriasis Classification." International Journal of Computer Sciences and Engineering 12.1 (2024): 1-7.
APA Style Citation: Anushree Goswami, Nidhi Sharma, (2024). A YOLO-Powered Deep Learning Approach to Psoriasis Classification. International Journal of Computer Sciences and Engineering, 12(1), 1-7.
BibTex Style Citation:
@article{Goswami_2024,
author = {Anushree Goswami, Nidhi Sharma},
title = {A YOLO-Powered Deep Learning Approach to Psoriasis Classification},
journal = {International Journal of Computer Sciences and Engineering},
issue_date = {1 2024},
volume = {12},
Issue = {1},
month = {1},
year = {2024},
issn = {2347-2693},
pages = {1-7},
url = {https://www.ijcseonline.org/full_paper_view.php?paper_id=5656},
doi = {https://doi.org/10.26438/ijcse/v12i1.17}
publisher = {IJCSE, Indore, INDIA},
}
RIS Style Citation:
TY - JOUR
DO = {https://doi.org/10.26438/ijcse/v12i1.17}
UR - https://www.ijcseonline.org/full_paper_view.php?paper_id=5656
TI - A YOLO-Powered Deep Learning Approach to Psoriasis Classification
T2 - International Journal of Computer Sciences and Engineering
AU - Anushree Goswami, Nidhi Sharma
PY - 2024
DA - 2024/01/31
PB - IJCSE, Indore, INDIA
SP - 1-7
IS - 1
VL - 12
SN - 2347-2693
ER -
![]() |
![]() |
![]() |
128 | 166 downloads | 67 downloads |
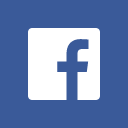
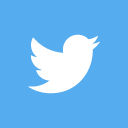
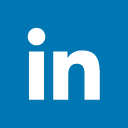
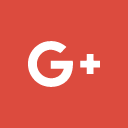
Abstract
With the rise of technological advancements, various clinical practices have undergone significant transformations. The field of dermatology, in particular, has experienced rapid progress. Skin ailments encompass a spectrum of conditions impacting the human body`s largest organ. These conditions range in severity from mild instances like acne or eczema to more serious cases such as skin cancer or Psoriasis, which is a persistent inflammatory skin disorder affecting a considerable global population. The precise categorization and assessment of the severity of Psoriasis play a pivotal role in its effective treatment and management. Conventional classification methodologies often prove subjective, time-intensive, and susceptible to variations in interpretation amongst different observers. In contrast, machine learning and deep learning, subsets of artificial intelligence, are revolutionizing various domains by addressing diverse challenges autonomously, without the need for human intervention. AI technologies have opened up fresh avenues for the objective and automated classification of Psoriasis. However, these technologies are yet to attain their maximum potential in terms of accuracy. Here, we try to implement a relatively new method i.e., YOLO (You Only Look Once) which is basically an object detection technique, to try to classify psoriasis. A comparison of all the different models of YOLOv8 have been studied here. The study also deploys the Google Colab platform for computational needs and ease.
Key-Words / Index Term
Psoriasis, Classification, YOLOv8, Artificial Intelligence, Deep Learning, Dermatology, Google Colab
References
[1] A. B. Kuchekar, R. R. Pujari, S. B. Kuchekar, S. N. Dhole, and P. M. Mule, “Psoriasis: A comprehensive review,” International Journal of pharmacy & life sciences, Vol.2, No.6, 2011.
[2] A. W. Armstrong, M. D. Mehta, C. W. Schupp, G. C. Gondo, S. J. Bell, and C. E. M. Griffiths, “Psoriasis Prevalence in Adults in the United States,” JAMA Dermatol, Vol.157, No.8, pp.940–946, Aug. 2021, doi: 10.1001/jamadermatol.2021.2007.
[3] R. Parisi, D. P. M. Symmons, and C. E. Griffiths, “i Ashcroft, DM. Global epidemiology of psoriasis: a systematic review of incidence and prevalence,” Journal of Investigative Dermatology, Vol.133, No.2, pp.377–385, 2013.
[4] E. M. Farber and L. Nall, “Pustular psoriasis.,” Cutis, Vol.51, No.1, pp.29–32, 1993.
[5] E. M. Farber and L. Nall, “Nail psoriasis.,” Cutis, Vol.50, No.3, pp.174–178, 1992.
[6] R. K. Singh et al., “Erythrodermic psoriasis: pathophysiology and current treatment perspectives,” Psoriasis: Targets and Therapy, Vol.6, pp.93–104, 2016.
[7] Z. U. Syed and A. Khachemoune, “Inverse psoriasis: case presentation and review,” Am J Clin Dermatol, Vol.12, pp.143–146, 2011.
[8] M. Kumar, “AYURVEDIC PERSPECTIVE OF PSORIASIS: A CASE STUDY,” World Journal of Pharmaceutical Research, Vol.10, Issue 3, pp.2250-2255, 2021.
[9] R. J. G. Chalmers, T. O’sullivan, C. M. Owen, and C. E. M. Griffiths, “A systematic review of treatments for guttate psoriasis,” British Journal of Dermatology, Vol.145, No.6, pp. 891–894, 2001.
[10] H.-J. Jang and K.-O. Cho, “Applications of deep learning for the analysis of medical data,” Arch Pharm Res, Vol.42, pp.492–504, 2019.
[11] L. Wang and K. Zuo, “Medical Data Classification Assisted by Machine Learning Strategy.,” Computational and Mathematical Methods in Medicine, Vol.2022, 2022.
[12] T. Davenport and R. Kalakota, “The potential for artificial intelligence in healthcare,” Future Health J, Vol.6, No.2, pp.94, 2019.
[13] T. Diwan, G. Anirudh, and J. V Tembhurne, “Object detection using YOLO: Challenges, architectural successors, datasets and applications,” Multimed Tools Appl, Vol.82, No.6, pp.9243–9275, 2023.
[14] L. Liu et al., “Deep learning for generic object detection: A survey,” Int J Comput Vis, Vol.128, pp.261–318, 2020.
[15] S. M. Anwar, M. Majid, A. Qayyum, M. Awais, M. Alnowami, and M. K. Khan, “Medical image analysis using convolutional neural networks: a review,” J Med Syst, Vol.42, pp.1–13, 2018.
[16] N. Tajbakhsh et al., “Convolutional neural networks for medical image analysis: Full training or fine tuning?,” IEEE Trans Med Imaging, Vol.35, No.5, pp.1299–1312, 2016.
[17] D. Po?ap, A. Winnicka, K. Serwata, K. K?sik, and M. Wo?niak, “An intelligent system for monitoring skin diseases,” Sensors, Vol. 18, No. 8, pp.2552, 2018.
[18] N. Amin and M. S. Farooq, “Automated Psoriasis Detection using Deep Learning,” Vol.9, No.3, pp.93–101, Sep. 2021.
[19] S. F. Aijaz, S. J. Khan, F. Azim, C. S. Shakeel, and U. Hassan, “Deep learning application for effective classification of different types of psoriasis,” J Health Eng, Vol.2022, 2022.
[20] M. Dash, N. D. Londhe, S. Ghosh, A. Semwal, and R. S. Sonawane, “PsLSNet: Automated psoriasis skin lesion segmentation using modified U-Net-based fully convolutional network,” Biomed Signal Process Control, Vol.52, pp.226–237, 2019.
[21] V. K. Shrivastava, N. D. Londhe, R. S. Sonawane, and J. S. Suri, “Reliable and accurate psoriasis disease classification in dermatology images using comprehensive feature space in machine learning paradigm,” Expert Syst Appl, Vol.42, No.15–16, pp.6184–6195, 2015.
[22] L.-H. Juang and M.-N. Wu, “Psoriasis image identification using k-means clustering with morphological processing,” Measurement, Vol.44, No.5, pp.895–905, 2011, doi: https://doi.org/10.1016/j.measurement.2011.02.006.
[23] Dr. Chelsea Jones and Dr. Monisha Gupta, “Psoriasis,” DermNet.
[24] N. Ruhil, D. Sahini, Anushka, A. Wadhwa and A. Sharma, “Pothole Detection and Reporting System Implementation Using Yolov8 and TensorFlow.js,” Int J Comp Sciences and Engineering, Vol.11, Issue.12, pp.26-31, 2023.