Recommendation Systems in Online Retail: A Comprehensive Survey of AI Techniques
Yash Patil1 , Samidha Ashtikar2 , Sakshi Shirodkar3 , Krishna Dudhate4 , Shraddha V. Pandit5
- Dept. of Artificial Intelligence and Data Science, PES’s Modern College of Engineering, India.
- Dept. of Artificial Intelligence and Data Science, PES’s Modern College of Engineering, India.
- Dept. of Artificial Intelligence and Data Science, PES’s Modern College of Engineering, India.
- Dept. of Artificial Intelligence and Data Science, PES’s Modern College of Engineering, India.
- Dept. of Artificial Intelligence and Data Science, PES’s Modern College of Engineering, India.
Section:Review Paper, Product Type: Journal Paper
Volume-12 ,
Issue-2 , Page no. 30-36, Feb-2024
CrossRef-DOI: https://doi.org/10.26438/ijcse/v12i2.3036
Online published on Feb 28, 2024
Copyright © Yash Patil, Samidha Ashtikar, Sakshi Shirodkar, Krishna Dudhate, Shraddha V. Pandit . This is an open access article distributed under the Creative Commons Attribution License, which permits unrestricted use, distribution, and reproduction in any medium, provided the original work is properly cited.
View this paper at Google Scholar | DPI Digital Library
How to Cite this Paper
- IEEE Citation
- MLA Citation
- APA Citation
- BibTex Citation
- RIS Citation
IEEE Style Citation: Yash Patil, Samidha Ashtikar, Sakshi Shirodkar, Krishna Dudhate, Shraddha V. Pandit, “Recommendation Systems in Online Retail: A Comprehensive Survey of AI Techniques,” International Journal of Computer Sciences and Engineering, Vol.12, Issue.2, pp.30-36, 2024.
MLA Style Citation: Yash Patil, Samidha Ashtikar, Sakshi Shirodkar, Krishna Dudhate, Shraddha V. Pandit "Recommendation Systems in Online Retail: A Comprehensive Survey of AI Techniques." International Journal of Computer Sciences and Engineering 12.2 (2024): 30-36.
APA Style Citation: Yash Patil, Samidha Ashtikar, Sakshi Shirodkar, Krishna Dudhate, Shraddha V. Pandit, (2024). Recommendation Systems in Online Retail: A Comprehensive Survey of AI Techniques. International Journal of Computer Sciences and Engineering, 12(2), 30-36.
BibTex Style Citation:
@article{Patil_2024,
author = {Yash Patil, Samidha Ashtikar, Sakshi Shirodkar, Krishna Dudhate, Shraddha V. Pandit},
title = {Recommendation Systems in Online Retail: A Comprehensive Survey of AI Techniques},
journal = {International Journal of Computer Sciences and Engineering},
issue_date = {2 2024},
volume = {12},
Issue = {2},
month = {2},
year = {2024},
issn = {2347-2693},
pages = {30-36},
url = {https://www.ijcseonline.org/full_paper_view.php?paper_id=5664},
doi = {https://doi.org/10.26438/ijcse/v12i2.3036}
publisher = {IJCSE, Indore, INDIA},
}
RIS Style Citation:
TY - JOUR
DO = {https://doi.org/10.26438/ijcse/v12i2.3036}
UR - https://www.ijcseonline.org/full_paper_view.php?paper_id=5664
TI - Recommendation Systems in Online Retail: A Comprehensive Survey of AI Techniques
T2 - International Journal of Computer Sciences and Engineering
AU - Yash Patil, Samidha Ashtikar, Sakshi Shirodkar, Krishna Dudhate, Shraddha V. Pandit
PY - 2024
DA - 2024/02/28
PB - IJCSE, Indore, INDIA
SP - 30-36
IS - 2
VL - 12
SN - 2347-2693
ER -
![]() |
![]() |
![]() |
108 | 110 downloads | 39 downloads |
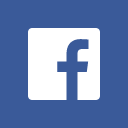
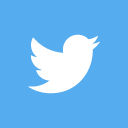
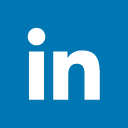
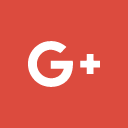
Abstract
Recommender systems play a vital role in providing pertinent content across diverse domains, such as entertainment, social networks, healthcare, education, travel, cuisine, and tourism. This review offers a thorough examination of cutting-edge recommender systems, as well as hybrid recommender systems. Hybrid models, combining different recommendation approaches, have gained prominence in enhancing system performance. The study classifies several models of hybridization and arranges the literature depending on the hybrid model and the applied machine learning methods in each study. Additionally, a systematic literature review examines the landscape of recommender systems over the last few years, emphasizing the quantitative aspects of research in this field. The review explores challenges, data mining techniques, recommendation strategies. It identifies common issues, such as addressing cold-start, accuracy, scalability and data sparsity, and highlights emerging challenges, including adapting to evolving user contexts and tastes.. Given the ongoing significance of hybrid recommenders, the review proposes exploring fresh possibilities such as utilizing parallel hybrid algorithms, and handling more extensive datasets, to address the evolving requirements of users.
Key-Words / Index Term
Artificial Intelligence, Collaborative Filtering, Recommendation System, Hybrid Recommendation System, Data Mining.
References
[1] R. Sharma, S. Rani and S. Tanwar, "Machine Learning Algorithms for building Recommender Systems," 2019 International Conference on Intelligent Computing and Control Systems (ICCS), Madurai, India, pp.785-790, 2019.
[2] G. Eason, B. Noble, and I.N. Sneddon, “On certain integrals of [1] Çano, E. and Morisio, M., 2017. Hybrid recommender systems: A systematic literature review. Intelligent Data Analysis, Vol.21, Issue.6, pp.1487-1524, 2017.
[3] Ricci, F., Rokach, L. and Shapira, B., 2011. Introduction to recommender systems handbook. In Recommender systems handbook. Springer, Boston, MA. pp.1-35, 2011.
[4] Jannach, D., Zanker, M., Felfernig, A. and Friedrich, G., 2010. Recommender systems: an introduction. Cambridge University Press. 2010.
[5] Hu, Y., Koren, Y., & Volinsky, C. (2008). Collaborative filtering for implicit feedback datasets. 2008 Eighth IEEE International Conference on Data Mining, pp.263-272, 2008.
[6] Herlocker, J.L., Konstan, J.A., Terveen, L.G. and Riedl, J.T., 2004. Evaluating collaborative filtering recommender systems. ACM Transactions on Information Systems, 22, pp.5-53, 2004.
[7] He, X., Liao, L., Zhang, H., Nie, L., Hu, X., & Chua, T. S. (2017). Neural collaborative filtering. Proceedings of the 26th international conference on World Wide Web, pp.173-182, 2017.
[8] Shani, G., Gunawardana, A. (2011). Evaluating Recommendation Systems. In: Ricci, F., Rokach, L., Shapira, B., Kantor, P. (eds) Recommender Systems Handbook. Springer, Boston, MA. 2011.
[9] Thorat, P.B., Goudar, R.M. and Barve, S., 2015. Survey on collaborative filtering, content-based filtering and hybrid recommendation system. International Journal of Computer Applications, 110(4), pp.31-36, 2015.
[10] Pazzani, M.J., Billsus, D. (2007). Content-Based Recommendation Systems. In: Brusilovsky, P., Kobsa, A., Nejdl, W. (eds) The Adaptive Web. Lecture Notes in Computer Science, vol 4321. Springer, Berlin, Heidelberg. 2007.
[11] Burke, R. (2007). Hybrid Web Recommender Systems. In: Brusilovsky, P., Kobsa, A., Nejdl, W. (eds) The Adaptive Web. Lecture Notes in Computer Science, vol 4321. Springer, Berlin, Heidelberg. 2007.
[12] Balabanovic, Marko & Shoham, Yoav. (1997). Fab: Content-Based, Collaborative Recommendation. Communications of the ACM. 40. 66-72. 10.1145/245108.245124.
[13] Adomavicius, Gediminas & Mobasher, Bamshad & Ricci, Francesco & Tuzhilin, Alexander. (2011). Context-Aware Recommender Systems. AI Magazine. 32. 67-80. 10.1609/aimag.v32i3.2364.
[14] Burke, R. (2002). Hybrid recommender systems: Survey and experiments. User Modeling and User-Adapted Interaction, Vol.12, Issue.4, pp.331-370, 2002.
[15] G. Adomavicius and A. Tuzhilin, "Toward the next generation of recommender systems: a survey of the state-of-the-art and possible extensions," in IEEE Transactions on Knowledge and Data Engineering, June, Vol.17, No.6, pp.734-749, 2005, doi: 10.1109/TKDE.2005.99.
[16] Linas Baltrunas, Bernd Ludwig, and Francesco Ricci. 2011. Matrix factorization techniques for context aware recommendation. In Proceedings of the fifth ACM conference on Recommender systems (RecSys `11). Association for Computing Machinery, New York, NY, USA, pp.301–304, 2011.
[17] Adomavicius, Gediminas & Kwon, YoungOk. (2007). New Recommendation Techniques for Multicriteria Rating Systems. Intelligent Systems, IEEE. 22. 48-55. 10.1109/MIS.2007.58.
[18] Robert M. Bell and Yehuda Koren. 2007. Lessons from the Netflix prize challenge. SIGKDD Explor. Newsl. 9, 2 (December 2007), pp.75-79, 2007.
[19] Shuai Zhang, Lina Yao, Aixin Sun, and Yi Tay. 2019. Deep Learning Based Recommender System: A Survey and New Perspectives. ACM Comput. Surv. 52, 1, Article 5 (January 2020), 38 pages.
[20] Mansur, Farhin & Patel, Vibha & Patel, Mihir. (2017). A review on recommender systems. pp.1-6, 2017. 10.1109/ICIIECS.2017.8276182.
[21] Zhang, Y., Zhao, M., & Wang, J. (2023). Exploiting temporal dynamics in recommender systems: A comprehensive survey. Knowledge and Information Systems, 1-28.
[22] Zhu, J., Zhang, P., Chen, X., Guo, M., & He, Q. (2023). A survey of graph neural networks for recommender systems. arXiv preprint arXiv:2301.07535.
[23] Liu, Y., Chen, L., Wu, X., Hu, B., & He, Q. (2023). Counterfactual recommendation with causal inference: A survey. arXiv preprint arXiv:2301.06765.
[24] Liu, Y., Li, M., & Zhu, X. (2023). Attention-based explainable ranking for fairness-aware recommendation. In Proceedings of the 46th International ACM SIGIR Conference on Research and Development in Information Retrieval, pp.331-340, 2023.
[25] Yu, J., Zhu, J., Shao, J., Jiang, J., & Wu, W. (2023). A Survey on Context-Aware Recommendation. IEEE Transactions on Knowledge and Data Engineering, Vol.35, Issue.7, pp.2209-2226, 2023.