AI-Driven Data Analytics Unveiling Sales Insights from Demographics and Beyond
Krishnamurty Raju Mudunuru1 , Rajesh Remala2 , Sevinthi Kali Sankar Nagarajan3
- Independent Researcher, San Antonio, Texas, USA.
- Independent Researcher, San Antonio, Texas, USA.
- Independent Researcher, San Antonio, Texas, USA.
Section:Research Paper, Product Type: Journal Paper
Volume-12 ,
Issue-5 , Page no. 11-18, May-2024
CrossRef-DOI: https://doi.org/10.26438/ijcse/v12i5.1118
Online published on May 31, 2024
Copyright © Krishnamurty Raju Mudunuru, Rajesh Remala, Sevinthi Kali Sankar Nagarajan . This is an open access article distributed under the Creative Commons Attribution License, which permits unrestricted use, distribution, and reproduction in any medium, provided the original work is properly cited.
View this paper at Google Scholar | DPI Digital Library
How to Cite this Paper
- IEEE Citation
- MLA Citation
- APA Citation
- BibTex Citation
- RIS Citation
IEEE Style Citation: Krishnamurty Raju Mudunuru, Rajesh Remala, Sevinthi Kali Sankar Nagarajan, “AI-Driven Data Analytics Unveiling Sales Insights from Demographics and Beyond,” International Journal of Computer Sciences and Engineering, Vol.12, Issue.5, pp.11-18, 2024.
MLA Style Citation: Krishnamurty Raju Mudunuru, Rajesh Remala, Sevinthi Kali Sankar Nagarajan "AI-Driven Data Analytics Unveiling Sales Insights from Demographics and Beyond." International Journal of Computer Sciences and Engineering 12.5 (2024): 11-18.
APA Style Citation: Krishnamurty Raju Mudunuru, Rajesh Remala, Sevinthi Kali Sankar Nagarajan, (2024). AI-Driven Data Analytics Unveiling Sales Insights from Demographics and Beyond. International Journal of Computer Sciences and Engineering, 12(5), 11-18.
BibTex Style Citation:
@article{Mudunuru_2024,
author = {Krishnamurty Raju Mudunuru, Rajesh Remala, Sevinthi Kali Sankar Nagarajan},
title = {AI-Driven Data Analytics Unveiling Sales Insights from Demographics and Beyond},
journal = {International Journal of Computer Sciences and Engineering},
issue_date = {5 2024},
volume = {12},
Issue = {5},
month = {5},
year = {2024},
issn = {2347-2693},
pages = {11-18},
url = {https://www.ijcseonline.org/full_paper_view.php?paper_id=5686},
doi = {https://doi.org/10.26438/ijcse/v12i5.1118}
publisher = {IJCSE, Indore, INDIA},
}
RIS Style Citation:
TY - JOUR
DO = {https://doi.org/10.26438/ijcse/v12i5.1118}
UR - https://www.ijcseonline.org/full_paper_view.php?paper_id=5686
TI - AI-Driven Data Analytics Unveiling Sales Insights from Demographics and Beyond
T2 - International Journal of Computer Sciences and Engineering
AU - Krishnamurty Raju Mudunuru, Rajesh Remala, Sevinthi Kali Sankar Nagarajan
PY - 2024
DA - 2024/05/31
PB - IJCSE, Indore, INDIA
SP - 11-18
IS - 5
VL - 12
SN - 2347-2693
ER -
![]() |
![]() |
![]() |
134 | 194 downloads | 60 downloads |
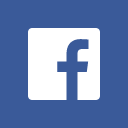
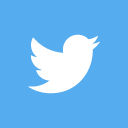
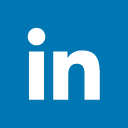
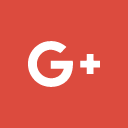
Abstract
Leveraging a synthesis of literature review and case studies, it illuminates how AI empowers organizations to discern intricate patterns and correlations within vast datasets. Through sophisticated algorithms and machine learning techniques, AI facilitates the nuanced understanding of the interplay between consumer demographics and purchasing behaviors, enabling targeted marketing strategies. Moreover, the study extends beyond demographics, encompassing psychographic, geographic, and behavioral factors through the amalgamation of diverse data sources. By employing predictive modeling, AI enables businesses to forecast market trends, optimize product positioning, and deliver personalized customer capabilities. Ethics around artificial intelligence-driven data analytics, incorporating consumer discretion and algorithmic fairness, are also addressed. Transparent methodologies and regulatory compliance are emphasized as crucial elements in fostering trust and mitigating risks. This paper explores the utilization of AI-driven data analytics in uncovering profound sales insights derived not only from demographics but also from diverse sources beyond traditional parameters. Machine learning deeper into consumer behavior patterns, market trends, and socio-economic indicators to gain a comprehensive understanding of their target audience. The paper discusses various methodologies employed in AI-driven data analytics, including predictive modeling, clustering techniques, and sentiment analysis, to extract valuable sales insights. Furthermore, it shows how crucial it is to incorporate data from various sources, including social media, geospatial information, and transactional records, to enrich the analytical process and enhance the accuracy of predictive models. Through real-world case studies and examples, this paper demonstrates how AI-driven data analytics can empower businesses to optimize their sales strategies, personalize marketing campaigns, and identify untapped market opportunities. By leveraging the capabilities of AI, organizations can move beyond traditional demographic segmentation and uncover nuanced insights that drive competitive advantage and foster sustainable growth.
Key-Words / Index Term
AI-driven data analytics, sales insights, demographics, machine learning, predictive modeling, consumer behavior, market trends, socio-economic indicators, personalized marketing
References
[1] Acquisti, L. Brandimarte, and G. Loewenstein, "Privacy and human behavior in the age of information," Science, Vol.347, No.6221, pp.509-514, 2019.
[2] S. LaValle, E. Lesser, R. Shockley, M. S. Hopkins, and N. Kruschwitz, "Big Data, Analytics and the Path from Insights to Value," MIT Sloan Management Review, 52, 2, 2011.
[3] T. H. Davenport and R. Ronanki, "Artificial intelligence for the real world," Harvard Business Review, Vol.96, No.1, pp.108-116, 2018.
[4] L. A. Siiman, et al., "Opportunities and Challenges for AI-Assisted Qualitative Data Analysis: An Example from Collaborative Problem-Solving Discourse Data," in International Conference on Innovative Technologies and Learning, Cham: Springer Nature Switzerland, 2023.
[5] Z. Wang, A. Maalla and M. Liang, "Research on E-Commerce Personalized Recommendation System based on Big Data Technology," 2021 IEEE 2nd International Conference on Information Technology, Big Data and Artificial Intelligence (ICIBA), Chongqing, China, 2021, pp.909-913.
[6] A. K. Sharma, N. Goel, J. Rajput and M. Bilal, "An Intelligent Model For Predicting the Sales of a Product," 2020 10th International Conference on Cloud Computing, Data Science & Engineering (Confluence), Noida, India, pp.341-345, 2020.
[7] V. Geetha, M. C. Manikandan, R. Rejith, R. S. Rishi, and K. Umapathy, "A Survey on E-Commerce Recommendation Systems Using Artificial Intelligence and Current Trends for Personalization to Improve Customer Experience," International Journal of Engineering Research & Technology (IJERT), March, Vol.13, No.3, 2024.
[8] D. Marupaka and S. Rangineni, "Machine Learning-Driven Predictive Data Quality Assessment in ETL Frameworks," International Journal of Computer Trends and Technology, Vol.72, No.3, pp.53-60, 2024.
[9] S. Rangineni and A. K. Bhardwaj, “Analysis Of DevOps Infrastructure Methodology and Functionality of Build Pipelines”, EAI Endorsed Scal Inf Syst, Jan., Vol.11, No.4, 2024.
[10] N. S. A. Polireddi, M. Suryadevara, S. Venkata, S. Rangineni, S. K. R. Koduru and S. Agal, "A Novel Study on Data Science for Data Security and Data Integrity with Enhanced Heuristic Scheduling in Cloud," 2023 2nd International Conference on Automation, Computing and Renewable Systems (ICACRS), Pudukkottai, India, pp.1862-1868, 2023.
[11] T. Esther, A. Akinsola, T. Ahmed, E. Makinde, K. Akinwande, and M. Mayowa, "A Review of the Ethics of Artificial Intelligence and Its Applications in the United States," International Journal on Cybernetics & Informatics (IJCI), Vol.12, No.6, 2023.
[12] Y. Qin and H. Li, "Sales forecast based on BP neural network," 2011 IEEE 3rd International Conference on Communication Software and Networks, Xi`an, China, pp.186-189, 2011.
[13] B. ?ater and J. Serafimova, "The Influence of Socio-Demographic Characteristics on Environmental Concern and Ecologically Conscious Consumer Behaviour among Macedonian Consumers," Economic and Business Review, Vol.21, No.2, 2019.
[14] N. A. Kumar, "Impact and Role of Artificial Intelligence in Sales and Marketing," i-manager`s Journal on Management, Vol.15, No.1, pp.1-6, 2020.
[15] V. Antonio, "How AI is changing sales,"Harvard Business Review, Vol.30, 2018.
[16] W. M. Lim, S. Kumar, N. Pandey, D. Verma, and D. Kumar, "Evolution and trends in consumer behavior: Insights from Journal of Consumer Behavior," Journal of Consumer Behavior, Vol.22, No.1, pp.217-232, 2023.
[17] Y. Gao and H. Liu, "Artificial intelligence-enabled personalization in interactive marketing: a customer journey perspective," Journal of Research in Interactive Marketing, Vol.17, No.5, pp.663-680, 2023.
[18] G. S. Gautham and S. Rao, "The Impact of Artificial Intelligence on Personalized Marketing," International Journal for Research in Applied Science & Engineering Technology (IJRASET), Vol.12, No.4, 2024.
[19] A. R. Gonçalves, D. C. Pinto, P. Rita, and T. Pires, "Artificial Intelligence and Its Ethical Implications for Marketing," Emerging Science Journal, Vol.7, No.2, 2023.
[20] P. Sinha, A. Shastri, and S. Lorimer, "How generative AI will change sales,"Harvard Business Review, 2023.
[21] C. Homburg, M. Droll, and D. Totzek, "Customer Prioritization: Does it Pay off, and How Should it be Implemented?" Journal of Marketing, Vol.72, No.5, pp.110-130, 2008.