A Novel Deep Learning Framework for the Detection of Tuberculosis using Chest X-ray Images
Sourabh Shastri1 , Shiwalika Sambyal2 , Sachin Kumar3 , Vibhakar Mansotra4
- Dept. of Computer Science and IT, University of Jammu, Jammu and Kashmir, India.
- Dept. of Computer Science and IT, University of Jammu, Jammu and Kashmir, India.
- Dept. of Computer Science and IT, University of Jammu, Jammu and Kashmir, India.
- Dept. of Computer Science and IT, University of Jammu, Jammu and Kashmir, India.
Section:Research Paper, Product Type: Journal Paper
Volume-12 ,
Issue-6 , Page no. 13-20, Jun-2024
CrossRef-DOI: https://doi.org/10.26438/ijcse/v12i6.1320
Online published on Jun 30, 2024
Copyright © Sourabh Shastri, Shiwalika Sambyal, Sachin Kumar, Vibhakar Mansotra . This is an open access article distributed under the Creative Commons Attribution License, which permits unrestricted use, distribution, and reproduction in any medium, provided the original work is properly cited.
View this paper at Google Scholar | DPI Digital Library
How to Cite this Paper
- IEEE Citation
- MLA Citation
- APA Citation
- BibTex Citation
- RIS Citation
IEEE Style Citation: Sourabh Shastri, Shiwalika Sambyal, Sachin Kumar, Vibhakar Mansotra, “A Novel Deep Learning Framework for the Detection of Tuberculosis using Chest X-ray Images,” International Journal of Computer Sciences and Engineering, Vol.12, Issue.6, pp.13-20, 2024.
MLA Style Citation: Sourabh Shastri, Shiwalika Sambyal, Sachin Kumar, Vibhakar Mansotra "A Novel Deep Learning Framework for the Detection of Tuberculosis using Chest X-ray Images." International Journal of Computer Sciences and Engineering 12.6 (2024): 13-20.
APA Style Citation: Sourabh Shastri, Shiwalika Sambyal, Sachin Kumar, Vibhakar Mansotra, (2024). A Novel Deep Learning Framework for the Detection of Tuberculosis using Chest X-ray Images. International Journal of Computer Sciences and Engineering, 12(6), 13-20.
BibTex Style Citation:
@article{Shastri_2024,
author = {Sourabh Shastri, Shiwalika Sambyal, Sachin Kumar, Vibhakar Mansotra},
title = {A Novel Deep Learning Framework for the Detection of Tuberculosis using Chest X-ray Images},
journal = {International Journal of Computer Sciences and Engineering},
issue_date = {6 2024},
volume = {12},
Issue = {6},
month = {6},
year = {2024},
issn = {2347-2693},
pages = {13-20},
url = {https://www.ijcseonline.org/full_paper_view.php?paper_id=5698},
doi = {https://doi.org/10.26438/ijcse/v12i6.1320}
publisher = {IJCSE, Indore, INDIA},
}
RIS Style Citation:
TY - JOUR
DO = {https://doi.org/10.26438/ijcse/v12i6.1320}
UR - https://www.ijcseonline.org/full_paper_view.php?paper_id=5698
TI - A Novel Deep Learning Framework for the Detection of Tuberculosis using Chest X-ray Images
T2 - International Journal of Computer Sciences and Engineering
AU - Sourabh Shastri, Shiwalika Sambyal, Sachin Kumar, Vibhakar Mansotra
PY - 2024
DA - 2024/06/30
PB - IJCSE, Indore, INDIA
SP - 13-20
IS - 6
VL - 12
SN - 2347-2693
ER -
![]() |
![]() |
![]() |
18 | 21 downloads | 9 downloads |
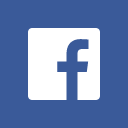
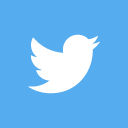
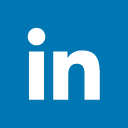
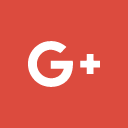
Abstract
Machine learning can play an important role in changing the dynamics of the modern healthcare system. In terms of the diagnosis field, Machine learning algorithms have offered tremendous support to Radiologists, healthcare workers, and other decision-makers. Early diagnosis of TB can stop the further spread and eventually mortality rate due to TB will fall. Currently, the standard method that is used for the diagnosis of TB takes one to four weeks while the rapid test takes 24 hours, so using Radiological images has an advantage over the existing standard method. In this paper, we have proposed a Novel Framework based on the application of Deep Learning to detect Tuberculosis (TB) using Chest X-ray images. In this work, 4200 images have been used to train the deep learning model. The model has achieved an accuracy of 99.41% in classifying Normal Chest X-rays and Tuberculosis (TB) Chest X-rays.
Key-Words / Index Term
Machine learning, Tuberculosis, Deep learning, Chest X-ray, Radiological images.
References
[1] D. Verma, C. Bose, N. Tufchi, K. Pant, V. Tripathi, and A. Thapliyal, “An efficient framework for identification of Tuberculosis and Pneumonia in Chest X-ray images using Neural Network,” Procedia Comput. Sci., Vol.171, No.2019, pp.217–224, 2020, doi: 10.1016/j.procs.2020.04.023.
[2] G. M. M. Alshmrani, Q. Ni, R. Jiang, H. Pervaiz, and N. M. Elshennawy, “A deep learning architecture for multi-class lung diseases classification using Chest X-ray (CXR) images,” Alexandria Eng. J., 2022, doi: 10.1016/j.aej.2022.10.053.
[3] S. Shastri, S. Kumar, K. Singh, and V. Mansotra, “Designing Contactless Automated Systems Using IoT, Sensors, and Artificial Intelligence to Mitigate COVID-19,” Internet of Things, pp.257–278, Mar. 2022, doi: 10.1201/9781003219620-13.
[4] L. J. Muhammad, E. A. Algehyne, S. S. Usman, A. Ahmad, C. Chakraborty, and I. A. Mohammed, “Supervised Machine Learning Models for Prediction of COVID-19 Infection using Epidemiology Dataset,” SN Comput. Sci., Vol.2, No.1, 2021, doi: 10.1007/s42979-020-00394-7.
[5] S. Khan and T. Yairi, “A review on the application of deep learning in system health management,” Mech. Syst. Signal Process., Vol.107, pp.241–265, 2018.
[6] S. Shastri, P. Kour, S. Kumar, K. Singh, and V. Mansotra, “GBoost: A novel Grading-AdaBoost ensemble approach for automatic identification of erythemato-squamous disease,” Int. J. Inf. Technol., Vol.13, No.3, pp.959–971, 2021, doi: 10.1007/s41870-020-00589-4.
[7] L. Martinez et al., “Infant BCG vaccination and risk of pulmonary and extrapulmonary tuberculosis throughout the life course: a systematic review and individual participant data meta-analysis,” Lancet Glob. Heal., Vol.10, No.9, pp.e1307–e1316, 2022, doi: 10.1016/S2214-109X(22)00283-2.
[8] T. R. Soares et al., “Evaluation of Chest X-ray with automated interpretation algorithms for mass tuberculosis screening in prisons: A cross-sectional study,” Lancet Reg. Heal. - Am., Vol.17, p. 100388, 2023, doi: 10.1016/j.lana.2022.100388.
[9] O. Hrizi et al., “Tuberculosis Disease Diagnosis Based on an Optimized Machine Learning Model,” J. Healthc. Eng., Vol.2022, 2022, doi: 10.1155/2022/8950243.
[10] S. M. Fati, E. M. Senan, and N. ElHakim, “Deep and Hybrid Learning Technique for Early Detection of Tuberculosis Based on X-ray Images Using Feature Fusion,” Appl. Sci., Vol.12, No.14, 2022, doi: 10.3390/app12147092.
[11] R. Mehrrotraa et al., “Ensembling of Efficient Deep Convolutional Networks and Machine Learning Algorithms for Resource Effective Detection of Tuberculosis Using Thoracic (Chest) Radiography,” IEEE Access, Vol.10, no. June, pp.85442–85458, 2022, doi: 10.1109/ACCESS.2022.3194152.
[12] C. Liu et al., “TX-CNN: Detecting tuberculosis in Chest X-ray images using convolutional neural network,” Proc. - Int. Conf. Image Process. ICIP, vol. 2017-Septe, pp.2314–2318, 2018, doi: 10.1109/ICIP.2017.8296695.
[13] R. Mohan, S. Kadry, V. Rajinikanth, A. Majumdar, and O. Thinnukool, “Automatic Detection of Tuberculosis Using VGG19 with Seagull-Algorithm,” Life, Vol.12, No.11, pp.1848, 2022, doi: 10.3390/life12111848.
[14] E. Showkatian, M. Salehi, H. Ghaffari, R. Reiazi, and N. Sadighi, “Deep learning-based automatic detection of tuberculosis disease in Chest X-ray images,” Polish J. Radiol., Vol.87, No.1, pp.118–124, 2022, doi: 10.5114/pjr.2022.113435.
[15] A. S. Becker et al., “Detection of tuberculosis patterns in digital photographs of Chest X-ray images using Deep Learning: Feasibility study,” Int. J. Tuberc. Lung Dis., Vol.22, No.3, pp.328–335, 2018, doi: 10.5588/ijtld.17.0520.
[16] M. Ayaz, F. Shaukat, and G. Raja, “Ensemble learning based automatic detection of tuberculosis in Chest X-ray images using hybrid feature descriptors,” Phys. Eng. Sci. Med., Vol.44, no.1, pp.183–194, 2021, doi: 10.1007/s13246-020-00966-0.
[17] L. Wang et al., “Distinguishing nontuberculous mycobacteria from Mycobacterium tuberculosis lung disease from CT images using a deep learning framework,” Eur. J. Nucl. Med. Mol. Imaging, Vol.48, No.13, pp.4293–4306, 2021, doi: 10.1007/s00259-021-05432-x.
[18] F. Weng et al., “Differentiation of intestinal tuberculosis and Crohn’s disease through an explainable machine learning method,” Sci. Rep., Vol.12, No.1, pp.1–12, 2022, doi: 10.1038/s41598-022-05571-7.
[19] N. Sasikaladevi, “Deep learning framework for the robust prognosis of Tuberculosis from radiography images based on fused linear triangular interpolation,” 2022.
[20] L. An et al., “Article E?TBNet: Light Deep Neural Network for Automatic Detection of Tuberculosis with X?ray DR Imaging,” Sensors, Vol.22, No.3, 2022, doi: 10.3390/s22030821.
[21] R. H. Abiyev and M. K. S. Ma’aitah, “Deep Convolutional Neural Networks for Chest Diseases Detection,” J. Healthc. Eng., Vol.2018, pp.4168538, 2018, doi: 10.1155/2018/4168538.
[22] Z. Z. Qin et al., “Using artificial intelligence to read Chest radiographs for tuberculosis detection: A multi-site evaluation of the diagnostic accuracy of three deep learning systems,” Sci. Rep., Vol.9, No.1, pp.1–10, 2019, doi: 10.1038/s41598-019-51503-3.