Artificial Intelligence in Credit Risk: Identifying and Preventing Credit Washing
Vijay Arpudaraj Antonyraj1
- Independent Researcher, Alpharetta, Georgia, USA.
Section:Research Paper, Product Type: Journal Paper
Volume-12 ,
Issue-12 , Page no. 33-39, Dec-2024
CrossRef-DOI: https://doi.org/10.26438/ijcse/v12i12.3339
Online published on Dec 31, 2024
Copyright © Vijay Arpudaraj Antonyraj . This is an open access article distributed under the Creative Commons Attribution License, which permits unrestricted use, distribution, and reproduction in any medium, provided the original work is properly cited.
View this paper at Google Scholar | DPI Digital Library
How to Cite this Paper
- IEEE Citation
- MLA Citation
- APA Citation
- BibTex Citation
- RIS Citation
IEEE Style Citation: Vijay Arpudaraj Antonyraj, “Artificial Intelligence in Credit Risk: Identifying and Preventing Credit Washing,” International Journal of Computer Sciences and Engineering, Vol.12, Issue.12, pp.33-39, 2024.
MLA Style Citation: Vijay Arpudaraj Antonyraj "Artificial Intelligence in Credit Risk: Identifying and Preventing Credit Washing." International Journal of Computer Sciences and Engineering 12.12 (2024): 33-39.
APA Style Citation: Vijay Arpudaraj Antonyraj, (2024). Artificial Intelligence in Credit Risk: Identifying and Preventing Credit Washing. International Journal of Computer Sciences and Engineering, 12(12), 33-39.
BibTex Style Citation:
@article{Antonyraj_2024,
author = {Vijay Arpudaraj Antonyraj},
title = {Artificial Intelligence in Credit Risk: Identifying and Preventing Credit Washing},
journal = {International Journal of Computer Sciences and Engineering},
issue_date = {12 2024},
volume = {12},
Issue = {12},
month = {12},
year = {2024},
issn = {2347-2693},
pages = {33-39},
url = {https://www.ijcseonline.org/full_paper_view.php?paper_id=5748},
doi = {https://doi.org/10.26438/ijcse/v12i12.3339}
publisher = {IJCSE, Indore, INDIA},
}
RIS Style Citation:
TY - JOUR
DO = {https://doi.org/10.26438/ijcse/v12i12.3339}
UR - https://www.ijcseonline.org/full_paper_view.php?paper_id=5748
TI - Artificial Intelligence in Credit Risk: Identifying and Preventing Credit Washing
T2 - International Journal of Computer Sciences and Engineering
AU - Vijay Arpudaraj Antonyraj
PY - 2024
DA - 2024/12/31
PB - IJCSE, Indore, INDIA
SP - 33-39
IS - 12
VL - 12
SN - 2347-2693
ER -
![]() |
![]() |
![]() |
32 | 55 downloads | 24 downloads |
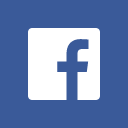
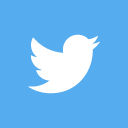
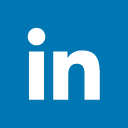
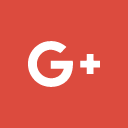
Abstract
Credit repair is the process of fixing a credit history that has one or more problems, such as errors, identity theft, or actual delinquencies and similar issues. Credit report inaccuracies can be disputed easily with the credit bureaus and at the same time whenever a consumer is affected by identity theft would require an extensive amount of investigation and steps to fix the same. As per Federal Trade Commission (FTC) guidelines, consumers are protected and have rules in place to dispute any fraudulent activity in their credit report. This loophole is being exploited by bad actors and credit repair companies to falsely raise a dispute on the recent activities of new tradlines, new mortgage, or fraudulent activities with the only aim to remove such activities from their credit file and boost their credit score which in turn they will use it to get more loans or open new tradelines. This process of intentionally raising false disputes to mislead the lenders and financial institutions is called Credit Washing.In other words, Credit Washing is the act of working with the credit bureaus to dispute legitimates charges with the intention of improving a previously reported low credit score, either by falsely disputing incorrect items (yourself or with the help of a company) or by falsely correcting certain financial behaviors. This journal discusses the basic understanding of Credit washing,its impacts on financial markets,risks associated,current measures to monitor and control credit washing, proposed enhanced methods of advanced predictive machine learning and AI capabilities to improve detection of credit washing in order to protect the financial interests of millions of people who are genuinely impacted by false reporting and also to safeguard consumer rights.
Key-Words / Index Term
Credit Washing, Machine Learning, AI, Automated Fraud Detection, Identify Theft, Predictive Modelling, Credit Score Manipulation, Behavioral Analytics
References
[1] Mohsin Ali Farhad, “Consumer data protection laws and their impact on business models in the tech industry”, Telecommunications Policy, Vol.48, Issue.9, pp.1-8, 2024.
[2] Victoria Prussen Spears, “Editor’s Note: Here’s What’s Happening in Arbitration”, Dispute Resolution Journal,Vol.78, Issue.2, pp.123-126, 2024
[3] Jeremy Burke, Julian Jamison, Dean Karlan, Kata Mihaly, Jonathan Zinman, “Credit Building or Credit Crumbling? A Credit Builder Loan’s Effects on Consumer Behavior and Market Efficiency in the United States”, The Review of Financial Studies, Vol.36, Issue.4, pp.1585-1620, 2022.
[4] Ma, Y,”Prediction of Default Probability of Credit-Card Bills”,Open Journal of Business and Management, Vol.8,Issue.1, pp.231-244, 2020.
[5] David Burnes, Marguerite DeLiema, Lynn Langton, “Risk and protective factors of identity theft victimization in the United States”, Preventive Medicine Reports, Vol.17, Issue.1, pp.1-8, 2020.
[6] Krishnamurty Raju Mudunuru, Rajesh Remala, Sevinthi Kali Sankar Nagarajan,“AI-Driven Data Analytics Unveiling Sales Insights from Demographics and Beyond,” International Journal of Computer Sciences and Engineering,Vol.12, Issue.5, pp.11-18, 2024.
[7] Rahul Singh, Deepti Gupta, "Enhancing Interpretable Anomaly Detection: Depth-based Extended Isolation Forest Feature Importance (DEIFFI)", International Journal of Computer Sciences and Engineering, Vol.12, Issue.5, pp.59-67, 2024.
[8] Abowd, J. M., Dwork, C., Karr, A. F., Nissim, K., Reiter, J., Slavkovi?, A. and Vilhuber, L, “On Privacy and Public Data: A study of data.gov.uk”, Journal of Privacy and Confidentiality, Vol.3, Issue.1, pp.51-65, 2011.
[9] Indra Setiawan , Humiras Hardi Purba, “A Systematic Literature Review of Key Performance Indicators (KPIs) Implementation”,Journal of Industrial Engineering & Management Research,Vol.1, Issue.3, pp.200-208, 2020.