News Based Trading Framework Using Genetic Programming
B.Sowmiya 1 , V.Geetha 2
Section:Review Paper, Product Type: Journal Paper
Volume-3 ,
Issue-8 , Page no. 148-152, Aug-2015
Online published on Aug 31, 2015
Copyright © B.Sowmiya , V.Geetha . This is an open access article distributed under the Creative Commons Attribution License, which permits unrestricted use, distribution, and reproduction in any medium, provided the original work is properly cited.
View this paper at Google Scholar | DPI Digital Library
How to Cite this Paper
- IEEE Citation
- MLA Citation
- APA Citation
- BibTex Citation
- RIS Citation
IEEE Style Citation: B.Sowmiya , V.Geetha, “News Based Trading Framework Using Genetic Programming,” International Journal of Computer Sciences and Engineering, Vol.3, Issue.8, pp.148-152, 2015.
MLA Style Citation: B.Sowmiya , V.Geetha "News Based Trading Framework Using Genetic Programming." International Journal of Computer Sciences and Engineering 3.8 (2015): 148-152.
APA Style Citation: B.Sowmiya , V.Geetha, (2015). News Based Trading Framework Using Genetic Programming. International Journal of Computer Sciences and Engineering, 3(8), 148-152.
BibTex Style Citation:
@article{_2015,
author = {B.Sowmiya , V.Geetha},
title = {News Based Trading Framework Using Genetic Programming},
journal = {International Journal of Computer Sciences and Engineering},
issue_date = {8 2015},
volume = {3},
Issue = {8},
month = {8},
year = {2015},
issn = {2347-2693},
pages = {148-152},
url = {https://www.ijcseonline.org/full_paper_view.php?paper_id=626},
publisher = {IJCSE, Indore, INDIA},
}
RIS Style Citation:
TY - JOUR
UR - https://www.ijcseonline.org/full_paper_view.php?paper_id=626
TI - News Based Trading Framework Using Genetic Programming
T2 - International Journal of Computer Sciences and Engineering
AU - B.Sowmiya , V.Geetha
PY - 2015
DA - 2015/08/31
PB - IJCSE, Indore, INDIA
SP - 148-152
IS - 8
VL - 3
SN - 2347-2693
ER -
![]() |
![]() |
![]() |
2383 | 2289 downloads | 2368 downloads |
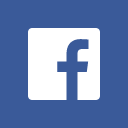
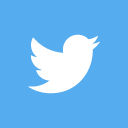
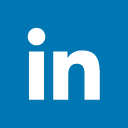
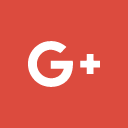
Abstract
The robotized PC programs utilizing data mining and prescient technologies do a fare sum of exchanges in the markets. Information mining is well founded on the hypothesis that the memorable data holds the key memory at that point again foreseeing the future direction. This innovation is composed to help speculators find covered up designs from the memorable data that have probable prescient capacity in their venture decisions. The forecast of stock markets is regarded as a testing assignment of monetary time arrangement prediction. Information investigation is one way of foreseeing in the occasion that future stocks costs will increment at that point again decrease. Five procedures of breaking down stocks were joined to anticipate in the occasion that the day’s shutting cost would increment at that point again decrease. These procedures were Regular Cost (TP), Bollinger Bands, Relative Quality List (RSI), CMI and Moving Normal (MA). This paper discussed different procedures which are able to anticipate with future shutting stock cost will increment at that point again diminish better than level of significance. Also, it investigated different worldwide events and their issues foreseeing on stock markets. It supports numerically and graphically.
Key-Words / Index Term
Information mining, Time arrangement Analysis, Binomial test, Regular Price, Bollinger Bands, Relative Quality List and Moving Average
References
[1] J. Borsje, F. Hogenboom, and F. Frasincar, “Semi-automatic financial events discovery based on lexico-semantic patterns,” International Journal of Web Engineering and Technology, vol. 6, no. 2, pp. 115–140, 2010.
[2] W. IJntema, J. Sangers, F. Hogenboom, and F. Frasincar, “A lexico-semantic pattern language for learning ontology instances from text,” Journal of Web Semantics: Science, Services and Agents on the World Wide Web, vol. 15, no. 1, pp. 37–50, 2012.
[3] P. C. Tetlock, “Giving content to investor sentiment: The role of media in the stock market,” Journal of Finance, vol. 62, no. 3, pp. 1139–1168, 2007.
[4] K. Mehta and S. Bhattacharyya, “Adequacy of training data for evolutionary mining of trading rules,” Decision Support Systems, vol. 37, no. 4, pp. 461–474, 2004.
[5] M. L. Mitchell and J. H. Mulherin, “The impact of public information on the stock market,” Journal of Finance, vol. 49, no. 3, pp. 923–950, 1994.
[6] W. S. Chan, “Stock price reaction to news and no-news: drift and reversal after headlines,” Journal of Financial Economics, vol. 70, no. 2, pp. 223–260, 2003.
[7] S. T. Kim, J. C. Lin, and M. B. Slovin, “Market structure, informed trading, and analyst’ recommendations,” Journal of Financial and Quantitative Analysis, vol. 32, no. 4, pp. 507–524, 1997.
[8] J. B. Warner, R. L. Watts, and K. H. Wruck, “Stock prices and top management changes,” Journal of Financial Economics, vol. 20, no. 1, pp. 461–492, 1988.
[9] Bonnier and R. F. Bruner, “An analysis of stock price reaction to management change in distressed firms,” Journal of Accounting and Economics, vol. 11, no. 1, pp. 95–106, 1989.
[10] D. L. Ikenberry and S. Ramnath, “Under reaction to self-selected news events: the case of stock splits,” Review of Financial Studies, vol. 15, no. 2, pp. 489–526, 2002.