Intrusion Detection and Violation of Compliance by Monitoring the Network
R.S. Priya1 , V. Anusha2 , N. Kumar3
Section:Research Paper, Product Type: Journal Paper
Volume-2 ,
Issue-3 , Page no. 84-91, Mar-2014
Online published on Mar 30, 2014
Copyright © R.S. Priya, V. Anusha, N. Kumar . This is an open access article distributed under the Creative Commons Attribution License, which permits unrestricted use, distribution, and reproduction in any medium, provided the original work is properly cited.
View this paper at Google Scholar | DPI Digital Library
How to Cite this Paper
- IEEE Citation
- MLA Citation
- APA Citation
- BibTex Citation
- RIS Citation
IEEE Style Citation: R.S. Priya, V. Anusha, N. Kumar, “Intrusion Detection and Violation of Compliance by Monitoring the Network,” International Journal of Computer Sciences and Engineering, Vol.2, Issue.3, pp.84-91, 2014.
MLA Style Citation: R.S. Priya, V. Anusha, N. Kumar "Intrusion Detection and Violation of Compliance by Monitoring the Network." International Journal of Computer Sciences and Engineering 2.3 (2014): 84-91.
APA Style Citation: R.S. Priya, V. Anusha, N. Kumar, (2014). Intrusion Detection and Violation of Compliance by Monitoring the Network. International Journal of Computer Sciences and Engineering, 2(3), 84-91.
BibTex Style Citation:
@article{Priya_2014,
author = {R.S. Priya, V. Anusha, N. Kumar},
title = {Intrusion Detection and Violation of Compliance by Monitoring the Network},
journal = {International Journal of Computer Sciences and Engineering},
issue_date = {3 2014},
volume = {2},
Issue = {3},
month = {3},
year = {2014},
issn = {2347-2693},
pages = {84-91},
url = {https://www.ijcseonline.org/full_paper_view.php?paper_id=75},
publisher = {IJCSE, Indore, INDIA},
}
RIS Style Citation:
TY - JOUR
UR - https://www.ijcseonline.org/full_paper_view.php?paper_id=75
TI - Intrusion Detection and Violation of Compliance by Monitoring the Network
T2 - International Journal of Computer Sciences and Engineering
AU - R.S. Priya, V. Anusha, N. Kumar
PY - 2014
DA - 2014/03/30
PB - IJCSE, Indore, INDIA
SP - 84-91
IS - 3
VL - 2
SN - 2347-2693
ER -
![]() |
![]() |
![]() |
3514 | 3451 downloads | 3627 downloads |
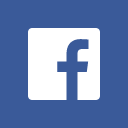
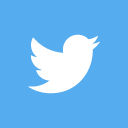
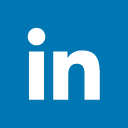
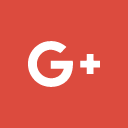
Abstract
Network and security of system has vital role in data communication environment. Web services and networks can be crashed on attempting many possible ways on forwarding by hackers or intruders. It causes malicious rapt in which it needs a technique called Intrusion Detection System through Spam Filtering. Thus gives the protection to networks. It can be done by using Open Source Network Intrusion Detection System called Snort. The process of arranging the e-mail with framed criteria called Spam Filtering. Proposed System, a Machine Learning Algorithm called Simple Probabilistic Navie Bayes Classifier used to detect the intrusion. Based on its content Probability of Spam messages can be calculated in Navie Bayes Classifier by learning it from spam and Good mail which results a robust, efficient anti-spam approach and adaption. Sniffing the packet and Fed it as input to Navie Bayes Classifier will give Test Dataset. Depends on spam and intrusion probability, the email is been classified as good or spam.
Key-Words / Index Term
Intrusion Detection, Navie Bayes Algorithm, Spam Filtering, Dynamic Tuning Mechanism
References
Androutsopoulos, J. Koutsias, V. Chandrinos, and D. Dpyropoulos, �An experimental comparison of Naive Naive Bayes and keyword-based anti-spam filtering with personal e-mail messages� In 23rd Annual Int. ACM SIGIR Conference on Research and Development in Information Retrieval, ISBN:1-58113-226-3, page no.160-167 , 2000.
[2]. Blum, T. Mitchell, �Combining labeled and unlabeled data with co-training�, in Proc. Workshop on Computational Learning Theory,ISBN:1-58113-057-0, page no.92-100, 1998.
[3]. Daniel Grossman, Pedro Domingos University of Washington, Seattle, WA �Learning Naive Bayes network classifiers by maximizing conditional likelihood� 21st international conference on Machine learning table of contents Banff, Alberta, Canada, Learning (IDEAL04), UK
ISBN: 1-58113-838-5, page no.361-368, 2004.
[4]. H. Drucker, D. Wu, and V.N. Vapnik, �Support vector machines for spam categorization �IEEE Transactions on Neural Networks, vol. 10, no. 5, page no. 1048-1054 , 1999.
[5]. Jonathan Palmer, �Naive Bayes Classification for Intrusion Detection using Live Packet Capture�, data mining in bioinformatics, 2011.
[6]. J. Provost �Naive-Bayes vs. rule-learning in classification of e-mail� The University of Texas at Austin, Department of Computer Sciences Rep, AI-TR .99-284, 1999.
[7]. M Rogati, Y Yang, �High-Performing Feature Selection for Text Classification`, CSD, Carnegie Mellon University, CIKM�02, ISBN: 1-58113-492-4, page no.659-661, 2002.
[8]. G. Sakkis, I. Androutsopoulos, G. Paliouras, �A memory-based approach to anti-spam filtering,� Information Retrieval, vol. 6, no.1, page no. 49-73, 2003.
[9]. F. Sebastiani, �Machine learning in automated text categorization� ACM Computing Surveys I vol. 34, no.1, page no.1-47, 2002.
[10]. X.-L. Wang, I. Cloete, �Learning to classify e-mail: A survey� In Proc. of the 4th Int. Conference. On Machine Learning and Cybernetics, Guangzhou.vol. 9, ISBN: 0-7803-9091-1, page no.5716-5719, 2005.