Development of Automatic Fracture Detection System using Image Processing and Classification Methods for Femur Bone X-Ray Images
M P Deshmukh1 , P D Deshmukh2
Section:Research Paper, Product Type: Journal Paper
Volume-4 ,
Issue-1 , Page no. 56-60, Jan-2016
Online published on Jan 31, 2016
Copyright © M P Deshmukh, P D Deshmukh . This is an open access article distributed under the Creative Commons Attribution License, which permits unrestricted use, distribution, and reproduction in any medium, provided the original work is properly cited.
View this paper at Google Scholar | DPI Digital Library
How to Cite this Paper
- IEEE Citation
- MLA Citation
- APA Citation
- BibTex Citation
- RIS Citation
IEEE Citation
IEEE Style Citation: M P Deshmukh, P D Deshmukh, “Development of Automatic Fracture Detection System using Image Processing and Classification Methods for Femur Bone X-Ray Images,” International Journal of Computer Sciences and Engineering, Vol.4, Issue.1, pp.56-60, 2016.
MLA Citation
MLA Style Citation: M P Deshmukh, P D Deshmukh "Development of Automatic Fracture Detection System using Image Processing and Classification Methods for Femur Bone X-Ray Images." International Journal of Computer Sciences and Engineering 4.1 (2016): 56-60.
APA Citation
APA Style Citation: M P Deshmukh, P D Deshmukh, (2016). Development of Automatic Fracture Detection System using Image Processing and Classification Methods for Femur Bone X-Ray Images. International Journal of Computer Sciences and Engineering, 4(1), 56-60.
BibTex Citation
BibTex Style Citation:
@article{Deshmukh_2016,
author = {M P Deshmukh, P D Deshmukh},
title = {Development of Automatic Fracture Detection System using Image Processing and Classification Methods for Femur Bone X-Ray Images},
journal = {International Journal of Computer Sciences and Engineering},
issue_date = {1 2016},
volume = {4},
Issue = {1},
month = {1},
year = {2016},
issn = {2347-2693},
pages = {56-60},
url = {https://www.ijcseonline.org/full_paper_view.php?paper_id=780},
publisher = {IJCSE, Indore, INDIA},
}
RIS Citation
RIS Style Citation:
TY - JOUR
UR - https://www.ijcseonline.org/full_paper_view.php?paper_id=780
TI - Development of Automatic Fracture Detection System using Image Processing and Classification Methods for Femur Bone X-Ray Images
T2 - International Journal of Computer Sciences and Engineering
AU - M P Deshmukh, P D Deshmukh
PY - 2016
DA - 2016/01/31
PB - IJCSE, Indore, INDIA
SP - 56-60
IS - 1
VL - 4
SN - 2347-2693
ER -
![]() |
![]() |
![]() |
1963 | 1737 downloads | 1654 downloads |
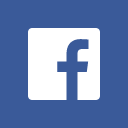
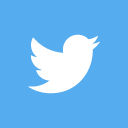
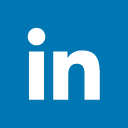
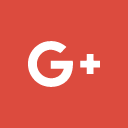
Abstract
Clinician and Practitioners suggest that detection of fractures from x-ray images is considered as an essential process in medical x- ray image analysis for diagnosis. Patients suffer in most cases seriously. So the study proposes a combined classification technique for automatic fracture detection from long bones, in particular the leg femur bones. The proposed system has following steps, preprocessing, segmentation, feature extraction and bone detection, which uses a combination of classification techniques of image processing for successful detection of fractures. The classifiers, Support Vector Machine Classifiers (SVM), feed forward Back Propagation Neural Networks (BPNN), and Naïve Bayes Classifiers (NB) are used during combination of classification. The results from various experiments showed that the proposed system is showing significant improvement in terms of detection rate of fractures.
Key-Words / Index Term
SVM, BPNN, Navie Base Calssification
References
[1] Luisier, F., Vonesch, C., Blu, T. and Unser, M., “Fast interscale wavelet denoising of Poisson-corrupted images”, Science Direct, Signal Processing, Elsevier, Volume 90, Issue 2, Pp.(415–427), Feb 2010.
[2] Kaur, A. and Singh, K., “Speckle noise reduction by using wavelets”, National Conference on Computational Instrumentation, CSIO Chandigarh, INDIA, Pp. (198-203), NCCI March 19-20, 2010.
[3] Sharma, N. and Aggarwal, L.M., “Automated medical image segmentation techniques”, Journal of Med Physics, Vol. 35 , Pp.3-14. Jan-March 2010.
[4] Sakata M, and Ogawa,K., “Noise reduction and contrast enhancement for small-dose X-Ray images in wavelet domain”, IEEE Nuclear Science Symposium Conference Record (NSS/MIC), Orlando, FL, Pp. 2924-3654, Oct, 2009.
[5] Donnelley, M., “Computer aided long-bone segmentation and fracture detection”, Computer and Information Science, Ph.D Thesis, flinders university, Jan 15, 2008.
[6] Tamisiea, D.F., “Radiologic aspects of orthopedic diseases”, Mercier LR, ed. Practical Orthopedics. 6th ed. Philadelphia, Pa: Mosby Elsevier; Chapter 16, 2008.
[7] Zhang B. Fadili, M J Starc, J L and Digel, S W, “Fast Poisson nose removal by bi orthogonal harr domain hypothesis testing, statistical methodology”, Journal of the International Indian statistical Association, Elsevier, Vol. 5, Pp. 387-396, Feb, 2008.
[8] Federico, A. and Kaufmann, G.H., “Denoising in digital speckle pattern interferometry using wave atoms”, Opt. Lett., Vol. 32, Pp.1232-1234, 2007.
[9] Rajan, J. and Kaimal, M.R., “Image Denoising using Wavelet Embedded Anisotropic Diffusion (WEAD)”, Proceedings of IET International Conference on Visual Information Engineering, Pp 589-593, Sept 26-28, 2006.
[10] Lim, V.L.F., Leow, L.W., Chen, T., Howe, T.S. and Png, M.A., “Combining classifiers for bone fracture detection in X-ray images”, IEEE International Conference on Image Processing, ICIP 2005, Vol. 1, Pp.I - 1149-1152, 2005.
[11] Marusic, S., Deng, G., Tay, D.B.H., “Image Denoising Using Over-complete Wavelet Representations”, Proceedings of the European Signal Processing Conference, EUSIPCO 2005, Pp.1-4, 2005.
[12] Chen, Y., Yap, W.H., Leow, W.K., Howe, T.S. and Png, M.A., “Detecting femur fractures by texture analysis of trabeculae”, Proc. Int. Conf. on Pattern Recognition., 2004.
[13] Lim, S.E., Xing, Y., Chen, Y., Leow, W.K., Howe, T.S. and Png, M.A.,” Detection of femur and radius fractures in x-ray images”, Proc. 2nd Int. Conf. on Advances in Medical Signal and Info. Proc., 2004.
[14] Tian, T.P., Chen, Y., Leow, W.K., Hsu, W., Howe, T.S. and Png, M.A., “Computing neck-shaft angle of femur for x-ray fracture detection”, Proc. Int. Conf on Computer Analysis of Images and Patterns (LNCS 2756), Pp. 82–89, 2003.
[15] Niijima, M., “Wavelet image analysis”, Kagaku Gijutsu Publishing (in Japanese), 2000.
[16] Tsukahara, M., Haseyama, M. and Kitajima, H., “Removal of additive white noise using an adaptive Wiener filter with edge retention”, Trans IEICE 1998, J81-DII: 623–631, 1998.
[17] Pisano, E.D., Zong, S., Hemminger, B.M., DeLuca, M., Johnston, R.E., Muller, K., Braeuning, P.M. and Pizer, S.M., “Contrast limited adaptive histogram equalization image processing to improve the detection of simulated speculations in dense mammograms”, Journal of Digital Imaging, Vol. 11, No. 4, Pp. 193-200, 1998.
[18] Najman, L. and Schmitt, M., “Geodesic saliency of watershed contours and hierarchical segmentation, Pattern Analysis and Machine Intelligence”, IEEE Transactions on Pattern Analysis and Machine Intelligence, Vol.18, No. 12, Pp.1163-1173, 1996.
[19] Duda, R. and Hart, P., “Use of the hough transformation to detect lines and curves in pictures”, Comm. ACM, Vol . 15, Pp. 11–15, 1972