Review of Content Based Image Retrieval Using Low Level Features
Shraddha S.Katariya1 , Ulhas B.Shinde2
Section:Review Paper, Product Type: Journal Paper
Volume-4 ,
Issue-3 , Page no. 91-97, Mar-2016
Online published on Mar 30, 2016
Copyright © Shraddha S.Katariya , Ulhas B.Shinde . This is an open access article distributed under the Creative Commons Attribution License, which permits unrestricted use, distribution, and reproduction in any medium, provided the original work is properly cited.
View this paper at Google Scholar | DPI Digital Library
How to Cite this Paper
- IEEE Citation
- MLA Citation
- APA Citation
- BibTex Citation
- RIS Citation
IEEE Citation
IEEE Style Citation: Shraddha S.Katariya , Ulhas B.Shinde, “Review of Content Based Image Retrieval Using Low Level Features,” International Journal of Computer Sciences and Engineering, Vol.4, Issue.3, pp.91-97, 2016.
MLA Citation
MLA Style Citation: Shraddha S.Katariya , Ulhas B.Shinde "Review of Content Based Image Retrieval Using Low Level Features." International Journal of Computer Sciences and Engineering 4.3 (2016): 91-97.
APA Citation
APA Style Citation: Shraddha S.Katariya , Ulhas B.Shinde, (2016). Review of Content Based Image Retrieval Using Low Level Features. International Journal of Computer Sciences and Engineering, 4(3), 91-97.
BibTex Citation
BibTex Style Citation:
@article{S.Katariya_2016,
author = {Shraddha S.Katariya , Ulhas B.Shinde},
title = {Review of Content Based Image Retrieval Using Low Level Features},
journal = {International Journal of Computer Sciences and Engineering},
issue_date = {3 2016},
volume = {4},
Issue = {3},
month = {3},
year = {2016},
issn = {2347-2693},
pages = {91-97},
url = {https://www.ijcseonline.org/full_paper_view.php?paper_id=834},
publisher = {IJCSE, Indore, INDIA},
}
RIS Citation
RIS Style Citation:
TY - JOUR
UR - https://www.ijcseonline.org/full_paper_view.php?paper_id=834
TI - Review of Content Based Image Retrieval Using Low Level Features
T2 - International Journal of Computer Sciences and Engineering
AU - Shraddha S.Katariya , Ulhas B.Shinde
PY - 2016
DA - 2016/03/30
PB - IJCSE, Indore, INDIA
SP - 91-97
IS - 3
VL - 4
SN - 2347-2693
ER -
![]() |
![]() |
![]() |
1584 | 1467 downloads | 1581 downloads |
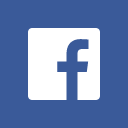
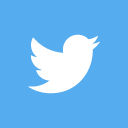
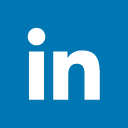
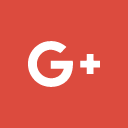
Abstract
Content based image retrieval is a important research area in the field of image processing used for searching and retrieving images from large database. It uses virtual content of images comprises of low level feature extraction such as color, texture, shape & spatial locations to represent images in the database. The system retrieves similar images images when an example image or sketch is presented as input to the system. This paper provides review of the approaches used for extracting low level features, various distance measures for retrieval, various datasets used in CBIR & performance measures. Creation of a content-based image retrieval system implies solving a number of difficult problems, including analysis of low-level image features and construction of feature vectors, multidimensional indexing, design of user interface, and data visualization. Quality of a retrieval system depends, first of all, on the feature vectors used, which describe image content. The paper presents a survey of common feature extraction and representation techniques and metrics of the corresponding feature spaces. Color, texture, and shape features are considered.
Key-Words / Index Term
Content based image retrieval (CBIR), Image retrieval, and feature extraction
References
[1] T. Gevers, Color in image Database, Intelligent Sensory Information Systems, University of Amsterdam, the Netherlands. 1998.
[2] X. Wan and C. C. Kuo, “Color distribution analysis and quantization for image retrieval”, In SPIE Storage and Retrieval for Image and Video Databases IV, Vol. SPIE 2670, pp. 9–16. 1996.
[3] M. W. Ying and Z. HongJiang, “Benchmarking of image feature for content-based retrieval”, IEEE. pp.253-257, 1998.
[4] Z. Zhenhua, L. Wenhui and L. Bo, “An Improving Technique of Color Histogram in Segmentation based Image Retrieval”, 2009 Fifth International Conference on Information Assurance and Security, IEEE, pp. 381-384, 2009.
[5] L. Haldurai and V. Vinodhini, "Parallel Indexing on Color and Texture Feature Extraction using R-Tree for Content Based Image Retrieval", International Journal of Computer Sciences and Engineering, Volume-03, Issue-11, Page No (11-15), Nov -2015
[6] S. Manimala and K. Hemachandran, “Performance analysis of Color Spaces in Image Retrieval”, Assam University Journal of science & Technology, Vol. 7 Number II pp. 94-104, 2011.
[7] J.R. Smith and S. Chang, “Transform Features for Texture Classification and Discrimination in Large Image Databases. Proceeding”, IEEE International Conference on Image Processing, Vol. 3, pp.407-411, 1994.
[8] B. Manjunath, P. Wu, S. Newsam and H. Shin, “A texture descriptor for browsing and similarity retrieval”, Journal of Signal Processing: Image Communication, vol. 16, pp. 33-43, 2000.
[9] H. Tamura, S. Mori and T. Yamawaki, “Textural features corresponding to visual perception”, IEEE Transactions. On Systems, Man and Cybern., Vol. 8, pp- 460-472, 1978.
[10] M. Ioka, “A Method of defining the similarity of images on the basis of color information”, Technical Report IBM Research, Tokyo Research Laboratory, 1989.
[11] H. James. H, S. Harpreet, W. Equits, M. Flickner and W. Niblack, “Efficient Color Histogram Indexing for Quadratic Form Distance Functions”, IEEE Transactions on Pattern Analysis and Machine Intelligence, Vol. 17, No. 7, 1995.
[12] J.R. Smith and S.F. Chang, “Automated Image Retrieval using Color and Texture”, Technical Report, Columbia University, 1995.
[13] V. V. Kumar, N. G. Rao, A. L. N. Rao and V. V. Krishna, “IHBM: Integrated Histogram Bin Matching For Similarity Measures of Color Image Retrieval”, International Journal of Signal Processing, Image Processing and Pattern Recognition Vol. 2, No.3, 2009.
[14] M. Swain, D. Ballard, “Color indexing”, International Journal of Computer Vision, Vol 7, pp-11–32, 1991.