Pattern Based Frequent Term Retrieval Search Using Text Clustering
R.Krithika 1 , G.Sathish Kumar2
Section:Research Paper, Product Type: Journal Paper
Volume-4 ,
Issue-4 , Page no. 292-297, Apr-2016
Online published on Apr 27, 2016
Copyright © R.Krithika, G.Sathish Kumar . This is an open access article distributed under the Creative Commons Attribution License, which permits unrestricted use, distribution, and reproduction in any medium, provided the original work is properly cited.
View this paper at Google Scholar | DPI Digital Library
How to Cite this Paper
- IEEE Citation
- MLA Citation
- APA Citation
- BibTex Citation
- RIS Citation
IEEE Citation
IEEE Style Citation: R.Krithika, G.Sathish Kumar, “Pattern Based Frequent Term Retrieval Search Using Text Clustering,” International Journal of Computer Sciences and Engineering, Vol.4, Issue.4, pp.292-297, 2016.
MLA Citation
MLA Style Citation: R.Krithika, G.Sathish Kumar "Pattern Based Frequent Term Retrieval Search Using Text Clustering." International Journal of Computer Sciences and Engineering 4.4 (2016): 292-297.
APA Citation
APA Style Citation: R.Krithika, G.Sathish Kumar, (2016). Pattern Based Frequent Term Retrieval Search Using Text Clustering. International Journal of Computer Sciences and Engineering, 4(4), 292-297.
BibTex Citation
BibTex Style Citation:
@article{Kumar_2016,
author = {R.Krithika, G.Sathish Kumar},
title = {Pattern Based Frequent Term Retrieval Search Using Text Clustering},
journal = {International Journal of Computer Sciences and Engineering},
issue_date = {4 2016},
volume = {4},
Issue = {4},
month = {4},
year = {2016},
issn = {2347-2693},
pages = {292-297},
url = {https://www.ijcseonline.org/full_paper_view.php?paper_id=935},
publisher = {IJCSE, Indore, INDIA},
}
RIS Citation
RIS Style Citation:
TY - JOUR
UR - https://www.ijcseonline.org/full_paper_view.php?paper_id=935
TI - Pattern Based Frequent Term Retrieval Search Using Text Clustering
T2 - International Journal of Computer Sciences and Engineering
AU - R.Krithika, G.Sathish Kumar
PY - 2016
DA - 2016/04/27
PB - IJCSE, Indore, INDIA
SP - 292-297
IS - 4
VL - 4
SN - 2347-2693
ER -
![]() |
![]() |
![]() |
1456 | 1404 downloads | 1478 downloads |
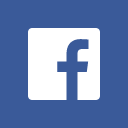
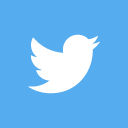
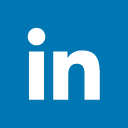
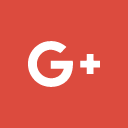
Abstract
Clients are known to experience troubles in dealing with information retrieval look outputs, particularly if those yields are above a certain size. It has been contended by several analysts that look yield Clustering can help clients in their collaboration with IR frameworks in some retrieval situations, providing them with an review of their results by abusing the topicality information that resides in the yield but has not been used at the retrieval stage. This review might enable them to find applicable records more effortlessly by focused on the most promising clusters, or to use the Groups as a starting-point for question refinement or expansion. In this paper, the results of tests carried out to assess the viability of Clustering as a look yield presentation technique are reported and discussed.
Key-Words / Index Term
Content Clustering, Pattern Mining, Content Retrieval, Clustering Algorithm
References
[1] Bastide Y., Taouil R., Pasquier N., Stumme G., and Lakhal L. (2000) “Mining frequent patterns with counting inference” ACM SIGKDD Explorations Newslett., vol. 2, no. 2, pp. 66–75.
[2] Bayardo Jr R. J. (1998) “Efficiently mining long patterns from databases” in Proc. ACM Sigmod Record, vol. 27, no. 2, pp. 85–93.
[3] Beil F., Ester M., and Xu X. (2002) “Frequent term-based text clustering” Proc. 8th ACM SIGKDD Int. Conf. Knowledge Discov. Data Minining, pp. 436–442.
[4] Cheng H., Yan X., Han J., and Hsu C.-W. (2007) “Discriminative frequent pattern analysis for effective classification” Proc. IEEE 23rd Int. Conf. Data Eng., pp. 716–725.
[5] Gao Y., Xu Y., Li Y., and Liu B. (2013) “A two-stage approach for generating topic models” in Advances in Knowledge Discovery and Data Mining, PADKDD’13. New York, NY, USA: Springer, pp. 221–232.
[6] Gao Y., Xu Y., and Li Y. (2013) “Pattern-based topic models for information filtering” in Proc. Int. Conf. Data Min. Workshop SENTIRE, pp. 921–928.
[7] Han J., Cheng H., Xin D., and Yan X. (2007) “Frequent pattern mining: Current status and future directions” Data Min. Knowl. Discov., vol. 15, no. 1, pp. 55–86.
[8] Robertson S., Zaragoza H., and Taylor M. (2004) ‘Simple BM25 extension to multiple weighted fields’ in Proc. 13th ACM Int. Conf. Inform. Knowl. Manag., pp. 42–49.
[9] Anuradha Awachar, Rajashree Bairagi, Vijayalaxmi Hegade and Mahadev Khandagale (2014), "An Overview of Ontology Based Text Document Clustering Algorithms", International Journal of Computer Sciences and Engineering, Volume-02, Issue-02, Page No (60-64
[10] Wang C. and Blei D. M. (2011) “Collaborative topic modeling for recommending scientific articles” in Proc. 17th ACM SIGKDD Int. Conf. Knowl. Discov. Data Min., pp. 448–456.
[11] Xu Y., Li Y., and Shaw G. (2011), “Reliable representations for association rules” Data Knowl. Eng., vol. 70, no. 6, pp. 555–575.
[12] Zaki M. J. and Hsiao C.-J. (2002) “CHARM: An efficient algorithm for closed item set mining.” in Proc. SDM, vol. 2, pp. 457–473.