Improving Accuracy of Obsolescence Detection Using Machine Learning
Sarangi Choudhari1 , Nisha Balani2 , Parul Jha3
Section:Research Paper, Product Type: Journal Paper
Volume-07 ,
Issue-12 , Page no. 111-113, May-2019
Online published on May 12, 2019
Copyright © Sarangi Choudhari, Nisha Balani, Parul Jha . This is an open access article distributed under the Creative Commons Attribution License, which permits unrestricted use, distribution, and reproduction in any medium, provided the original work is properly cited.
View this paper at Google Scholar | DPI Digital Library
How to Cite this Paper
- IEEE Citation
- MLA Citation
- APA Citation
- BibTex Citation
- RIS Citation
IEEE Style Citation: Sarangi Choudhari, Nisha Balani, Parul Jha, “Improving Accuracy of Obsolescence Detection Using Machine Learning,” International Journal of Computer Sciences and Engineering, Vol.07, Issue.12, pp.111-113, 2019.
MLA Style Citation: Sarangi Choudhari, Nisha Balani, Parul Jha "Improving Accuracy of Obsolescence Detection Using Machine Learning." International Journal of Computer Sciences and Engineering 07.12 (2019): 111-113.
APA Style Citation: Sarangi Choudhari, Nisha Balani, Parul Jha, (2019). Improving Accuracy of Obsolescence Detection Using Machine Learning. International Journal of Computer Sciences and Engineering, 07(12), 111-113.
BibTex Style Citation:
@article{Choudhari_2019,
author = {Sarangi Choudhari, Nisha Balani, Parul Jha},
title = {Improving Accuracy of Obsolescence Detection Using Machine Learning},
journal = {International Journal of Computer Sciences and Engineering},
issue_date = {5 2019},
volume = {07},
Issue = {12},
month = {5},
year = {2019},
issn = {2347-2693},
pages = {111-113},
url = {https://www.ijcseonline.org/full_spl_paper_view.php?paper_id=1056},
publisher = {IJCSE, Indore, INDIA},
}
RIS Style Citation:
TY - JOUR
UR - https://www.ijcseonline.org/full_spl_paper_view.php?paper_id=1056
TI - Improving Accuracy of Obsolescence Detection Using Machine Learning
T2 - International Journal of Computer Sciences and Engineering
AU - Sarangi Choudhari, Nisha Balani, Parul Jha
PY - 2019
DA - 2019/05/12
PB - IJCSE, Indore, INDIA
SP - 111-113
IS - 12
VL - 07
SN - 2347-2693
ER -
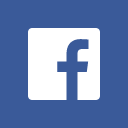
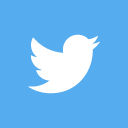
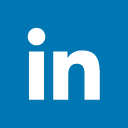
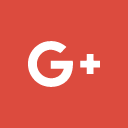
Abstract
Driven by the frequent technological changes and innovation, obsolescence has become a major challenge that cannot be ignored in which the life cycle of the components is often shorter than that of their systems. Basically, obsolescence problems are often sudden and not planned which causes delays and extra costs. On the other side forecasting appears to be one of the most efficient solutions to solve this problem. This paper aims to provide new light and help industries to generate different solutions to the problems of obsolescence. Specifically it presents a framework for forecasting the obsolescence based on random forest (RF) algorithm which has proven as the best predictor for forecasting obsolescence risk based on a previous comparative study with a high degree of accuracy.
Key-Words / Index Term
components, Data minig, Obsolescence
References
[1] Forecasting Obsolescence Risk and Product Life Cycle With Machine Learning- Connor Jennings, Student Member, IEEE, Dazhong Wu, and Janis Terpenny(SEPTEMBER 2016)
[2]Forecasting technology and part obsolescence-Peter Sandborn(July 2015).
[3]Forecasting Obsolescence Risk using Machine Learning- Connor Jennings, Dazhong Wu, Janis Terpenny-Center for e-Design Industrial and Manufacturing Systems Engineering Pennsylvania State University State College, Pennsylvania, 16801, USA
[4]Electronic Part Obsolescence Forecasting Based on Time Series Modeling-Jungmok Ma1 and Namhun Kim2,#
[5] Electronic Part Life Cycle Concepts and Obsolescence Forecasting- Rajeev Solomon, Peter A. Sandborn, Member, IEEE, and Michael G. Pecht, Fellow, IEEE
[6]Obsolescence and Life Cycle Management for Avionics(November 2015)
[7]A Random Forest Method for Obsolescence Forecasting-Grichi1Y. Beauregard1, T. M. Dao1
[8]Estimating obsolescence risk from demand data - a case studyWillem van Jaarsveld* and Rommert Dekker-( January 18, 2010).
[9]Using Learning-based Filters to Detect Rule-based Filtering Obsolescence by Francis Wolinski 1,2,Frantz Vichot 1,3 & Mathieu Stricker 1,4
[10] Generic Tools and Methods for Obsolescence Control G6rard Gaillat Thomson-CSF Technologies et MNthodes B.P. 56 91401 Orsay, France
[11] Obsolescence and Life Cycle Management for Avionics By Federal Aviation Administration William J. Hughes Technical Center Aviation Research Division Atlantic City International Airport New Jersey 08405
[12] Obsolescence by D. KAYE GAPEN SIGRID P. MILNER