BigData Analytics Predicting Risk of Readmissions of Diabetic Patients
G. Amrutha Varshini1 , Nafisa.S 2 , Priyanka 3 , S. Sri Vishnupriya4 , Ambika B.J5
Section:Research Paper, Product Type: Journal Paper
Volume-07 ,
Issue-14 , Page no. 99-102, May-2019
CrossRef-DOI: https://doi.org/10.26438/ijcse/v7si14.99102
Online published on May 15, 2019
Copyright © G. Amrutha Varshini, Nafisa.S, Priyanka, S. Sri Vishnupriya, Ambika B.J . This is an open access article distributed under the Creative Commons Attribution License, which permits unrestricted use, distribution, and reproduction in any medium, provided the original work is properly cited.
View this paper at Google Scholar | DPI Digital Library
How to Cite this Paper
- IEEE Citation
- MLA Citation
- APA Citation
- BibTex Citation
- RIS Citation
IEEE Style Citation: G. Amrutha Varshini, Nafisa.S, Priyanka, S. Sri Vishnupriya, Ambika B.J, “BigData Analytics Predicting Risk of Readmissions of Diabetic Patients,” International Journal of Computer Sciences and Engineering, Vol.07, Issue.14, pp.99-102, 2019.
MLA Style Citation: G. Amrutha Varshini, Nafisa.S, Priyanka, S. Sri Vishnupriya, Ambika B.J "BigData Analytics Predicting Risk of Readmissions of Diabetic Patients." International Journal of Computer Sciences and Engineering 07.14 (2019): 99-102.
APA Style Citation: G. Amrutha Varshini, Nafisa.S, Priyanka, S. Sri Vishnupriya, Ambika B.J, (2019). BigData Analytics Predicting Risk of Readmissions of Diabetic Patients. International Journal of Computer Sciences and Engineering, 07(14), 99-102.
BibTex Style Citation:
@article{Varshini_2019,
author = {G. Amrutha Varshini, Nafisa.S, Priyanka, S. Sri Vishnupriya, Ambika B.J},
title = {BigData Analytics Predicting Risk of Readmissions of Diabetic Patients},
journal = {International Journal of Computer Sciences and Engineering},
issue_date = {5 2019},
volume = {07},
Issue = {14},
month = {5},
year = {2019},
issn = {2347-2693},
pages = {99-102},
url = {https://www.ijcseonline.org/full_spl_paper_view.php?paper_id=1099},
doi = {https://doi.org/10.26438/ijcse/v7i14.99102}
publisher = {IJCSE, Indore, INDIA},
}
RIS Style Citation:
TY - JOUR
DO = {https://doi.org/10.26438/ijcse/v7i14.99102}
UR - https://www.ijcseonline.org/full_spl_paper_view.php?paper_id=1099
TI - BigData Analytics Predicting Risk of Readmissions of Diabetic Patients
T2 - International Journal of Computer Sciences and Engineering
AU - G. Amrutha Varshini, Nafisa.S, Priyanka, S. Sri Vishnupriya, Ambika B.J
PY - 2019
DA - 2019/05/15
PB - IJCSE, Indore, INDIA
SP - 99-102
IS - 14
VL - 07
SN - 2347-2693
ER -
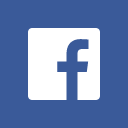
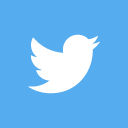
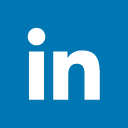
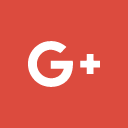
Abstract
Healthcare has huge impact on the society and also holds more importance in which analytics are applied to achieve accurate results about patients and to identify bottlenecks and to increase the business efficiency. Hospital readmissions are way too expensive and reflect the insufficiency in the healthcare system. Since readmission into hospitals has become unaffordable necessary measure needs to be taken to make them preventable [1] Readmissions rate decides the quality of treatment provided by the hospitals. Mostly readmissions are caused due to improper medication, early discharge, unmonitored discharge and poor care of hospital staff. In USA alone treatment of readmitted diabetics patients has exceeded over 250 million dollars per year. Advance identification of patient having high risk of readmission can allow the healthcare providers to perform additional investigations and also provides possibility to prevent readmissions. This method improves the quality of care and also reduces the medical expenses caused due to readmission .Number of patient visits, discharge order, type of admission were identified as the predicators of readmission. It was found that based on number of laboratory tests and discharge order both together predict whether the patient will be readmitted shortly after being discharged from the hospital (i.e. <30 days) or after a longer period of time (i.e. >30 days).These accurate results help the healthcare providers to improve care taken for diabetic patients.
Key-Words / Index Term
Machine Learning, Analysis on Medical data, Data collection, Data preprocessing, Data labeling, Predictive modeling, Model training, Prediction
References
[1] Donzé J. Aujesky D., Williams D., Schnipper J.L, MD. ―Potentially avoidable 30-day hospital readmissions in medical patients: Derivation and validation of a prediction model. JAMA Internal Medicine,‖173(8):632-638, Apr. 2013.
[2] Definition, Diagnosis and Classification of Diabetes Mellitus and its Complications,‖ Report of a WHO Consultation Part 1: Diagnosis and Classification of Diabetes Mellitus World Health Organization Department of Non communicable Disease Surveillance, Geneva, 1999.
[3] T. D. Briefing. Ahrq: The conditions that cause the most readmissions. The Daily Briefing. Web,2014..
[4] P.Yasodha and M. Kannan, "Analysis of a Population of Diabetic Patients Databases in WekaTool", International Journal of Scientific & Engineering Research.
[5] [2]A. Iyer, J. S and R. Sumbaly, "Diagnosis of Diabetes Using Classification Mining Techniques".
[6] UCI Machine Learning Repository http://www.ics.uci.edu/~mlearn/MLRepository.html
[7] T. D. Briefing. Ahrq: The conditions that cause the most readmissions. The Daily Briefing. Web, 2014.
[8] K. M. Dungan. The effect of diabetes on hospital readmissions. Journal of diabetes science and technology, 6(5):1045–1052, 2012.
[9] E. Eby, C. Hardwick, M. Yu, S. Gelwicks, K. Deschamps, J. Xie, and S. George. Predictors of 30 day hospital readmission in patients with type 2 diabetes: a retrospective, case-control, database study. Current Medical Research & Opinion, 31(1):107–114, 2014.
[10] H. Zhang. The optimality of naive bayes. AA, 1(2):3, 2004.