Mechanisation of Nuclei detection and segmentation:a leap in Medical Research
Besiahgari Dinesh1 , B.Kavya 2 , Besiahgari Sree Avinash3 , Sarvamangala D R4
Section:Research Paper, Product Type: Journal Paper
Volume-07 ,
Issue-14 , Page no. 116-120, May-2019
CrossRef-DOI: https://doi.org/10.26438/ijcse/v7si14.116120
Online published on May 15, 2019
Copyright © Besiahgari Dinesh, B.Kavya, Besiahgari Sree Avinash, Sarvamangala D R . This is an open access article distributed under the Creative Commons Attribution License, which permits unrestricted use, distribution, and reproduction in any medium, provided the original work is properly cited.
View this paper at Google Scholar | DPI Digital Library
How to Cite this Paper
- IEEE Citation
- MLA Citation
- APA Citation
- BibTex Citation
- RIS Citation
IEEE Style Citation: Besiahgari Dinesh, B.Kavya, Besiahgari Sree Avinash, Sarvamangala D R, “Mechanisation of Nuclei detection and segmentation:a leap in Medical Research,” International Journal of Computer Sciences and Engineering, Vol.07, Issue.14, pp.116-120, 2019.
MLA Style Citation: Besiahgari Dinesh, B.Kavya, Besiahgari Sree Avinash, Sarvamangala D R "Mechanisation of Nuclei detection and segmentation:a leap in Medical Research." International Journal of Computer Sciences and Engineering 07.14 (2019): 116-120.
APA Style Citation: Besiahgari Dinesh, B.Kavya, Besiahgari Sree Avinash, Sarvamangala D R, (2019). Mechanisation of Nuclei detection and segmentation:a leap in Medical Research. International Journal of Computer Sciences and Engineering, 07(14), 116-120.
BibTex Style Citation:
@article{Dinesh_2019,
author = {Besiahgari Dinesh, B.Kavya, Besiahgari Sree Avinash, Sarvamangala D R},
title = {Mechanisation of Nuclei detection and segmentation:a leap in Medical Research},
journal = {International Journal of Computer Sciences and Engineering},
issue_date = {5 2019},
volume = {07},
Issue = {14},
month = {5},
year = {2019},
issn = {2347-2693},
pages = {116-120},
url = {https://www.ijcseonline.org/full_spl_paper_view.php?paper_id=1103},
doi = {https://doi.org/10.26438/ijcse/v7i14.116120}
publisher = {IJCSE, Indore, INDIA},
}
RIS Style Citation:
TY - JOUR
DO = {https://doi.org/10.26438/ijcse/v7i14.116120}
UR - https://www.ijcseonline.org/full_spl_paper_view.php?paper_id=1103
TI - Mechanisation of Nuclei detection and segmentation:a leap in Medical Research
T2 - International Journal of Computer Sciences and Engineering
AU - Besiahgari Dinesh, B.Kavya, Besiahgari Sree Avinash, Sarvamangala D R
PY - 2019
DA - 2019/05/15
PB - IJCSE, Indore, INDIA
SP - 116-120
IS - 14
VL - 07
SN - 2347-2693
ER -
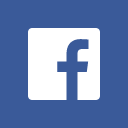
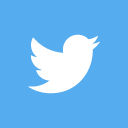
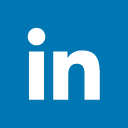
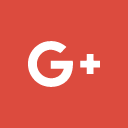
Abstract
The process of identifying and segmenting nuclei in the cell is a prerequisite for the analysis of various genetic disorders. The main carrier of genetic information in most of the living organisms is Deoxyribonucleic acid(DNA) which is present in the nucleus of the cell. Detection and segmentation of nuclei is laborious and time demanding. This paper intends to explore an untouched approach towards solving the issue by automating the process which drastically reduces the development time and required man power. Many classic methods like Otsu, watershed were proposed but they failed to accurately segment and few caused over segmentation. In the recent timespan, the executions of Convolutional Neural Networks (CNN) have made it evident that they demonstrate impressive performance on biomedical image classification. CNN methods also face issues with stipulation for hefty delineated tutoring data sets but in this context, a CNN architecture U-Net which is proficient of grasping knowledge from smaller pre-processed augmented data-set is proposed. The proposed encoder-decoder U-Net model indicates better execution in identifying genuine fragments contrasted with the cutting edge system for rapid CNN shows better performance in detecting true segments compared to the state-of-the-art technique Faster Recurrent-CNN (R-CNN).
Key-Words / Index Term
CNN, R-CNN, U-Net Model
References
[1] O.Ronneberger, P.Fischer,T. Brox, “U-Net: Convolutional Networks for Biomedical Image Segmentation”, arXiv:1505.04597v1 [cs.CV] 18 May 2015
[2] H.Irshad, A.Veillard, L.Roux, and Daniel Racoceanu, “Methods for Nuclei Detection, Segmentation, and Classification in Digital Histopathology: A Review—Current Status and Future Potential ” IEEE REVIEWS IN BIOMEDICAL ENGINEERING, VOL. 7, 2014
[3] ZENG,W.XIE,Y.ZHANG,YAO LU “RIC-Unet: An Improved Neural Network Based on Unet for Nuclei Segmentation in Histology Images” 10.1109/ACCESS.2019.2896920, IEEE
[4] J. Kong, L. Cooper, T. Kurc, D. Brat, and J. Saltz, “Towards building computerized image analysis framework for nucleus discrimination in microscopy images of diffuse glioma,” in Proc. IEEE 33rd Annu. Int. Conf. Eng. Med. Biol. Soc., Boston, MA, USA, Aug. 30–Sep. 3, 2011, pp. 6605–6608.
[5] V.-T. Ta, O. Lezoray, A. Elmoataz, and S. Sch ´ upp, “Graph-based tools ¨ for microscopic cellular image segmentation,” Pattern Recog., vol. 42, no. 6, pp. 1113–1125, 2009.
[6] Md Alom, Chris Yakopcic, M. Taha , V.K.Asari “Nuclei Segmentation with Recurrent Residual Convolutional Neural Networks based U-Net (R2U-Net)” IEEE 2018
[7] A. Hafiane, F. Bunyak, and K. Palaniappan, “Clustering initiated multiphase active contours and robust separation of nuclei groups for tissue segmentation,” in Proc. 19th Int. Conf. Pattern Recog., Tampa, FL, USA, Dec. 2008
[8] C. Wahlby, I. M. Sintorn, F. Erlandsson, G. Borgefors, and E. Bengtsson, “Combining intensity, edge and shape information for 2D and 3D segmentation of cell nuclei in tissue sections,” J. Microsc., vol. 215, no. 1, pp. 67–76, 2004
[9] J. Vink, M. V. Leeuwen, C. V. Deurzen, and G. Haan, “Efficient nucleus detector in histopathology images,” J. Microsc., vol. 249, no. 2, pp. 124– 135, 2013
[10] Yu He,Xi Yu,Chang Liu,Jian Zhang,Ke Hu,H C Zhu “A 3D Dual Path U-Net of Cancer Segmentation Based on MRI” 3rd IEEE International Conference on Image, Vision and Computing 2018.
[11] S. Wienert, D. Heim, K. Saeger, A. Stenzinger, M. Beil, P. Hufnagl, M. Dietel, C. Denkert, and F. Klauschen, “Detection and segmentation of cell nuclei in virtual microscopy images: A minimum-model approach,” Sci. Rep., vol. 2, 7 pp., 2012.
[12] H. Chang, L. A. Loss, and B. Parvin, “Nuclear segmentation in H&E sections via multi-reference graph cut (MRGC),” in Proc. 9th IEEE Int. Symp. Biomed. Imag.: Nano Macro, Barcelona, Spain, pp. 614– 617, 2012
[13] S. M.K Hasan and Cristian A. Linte,Chester F. Carlson “A Modified U-Net Convolutional Network Featuring a Nearest-neighbor Re-sampling-based Elastic-Transformation for Brain Tissue Characterization and Segmentation” IEEE 2018
[14] C. Jung and C. Kim, “Segmenting clustered nuclei using h-minima transform-based marker extraction and contour parameterization,” IEEE Trans. Biomed. Eng., vol. 57, no. 10, pp. 2600–2604, Oct. 2010.
[15] C. Jung, C. Kim, S. W. Chae, and S. Oh, “Unsupervised segmentation of overlapped nuclei using Bayesian classification,” IEEE Trans. Biomed. Eng., vol. 57, no. 12, pp. 2825–2832, Dec. 2010.
[16] Martin Kolaˇr´ık, Radim Burget, Vaclav Uher, Malay Kishore Dutta “3D Dense-U-Net for MRI Brain Tissue Segmentation”IEEE 2018
[17] S. Ali and A. Madabhushi, “An integrated region-, boundary-, shapebased active contour for multiple object overlap resolution in histological imagery,” IEEE Trans. Med. Imag., vol. 31, no. 7, pp. 1448–1460, Jul. 2012
[18] Ling Luo,Dali Chen,Dingyu Xue1 “Retinal Blood Vessels Semantic Segmentation Method Based on Modified U-Net” IEEE 2018
[19] M. Dundar, S. Badve, G. Bilgin, V. C. Raykar, R. K. Jain, O. Sertel, and M. N. Gurcan, “Computerized classification of intraductal breast lesions using histopathological images,” IEEE Trans. Biomed. Eng., vol. 58, no. 7, pp. 1977–1984, Jul. 2011.
[20] L. Yang, O. Tuzel, P. Meer, and D. Foran, “Automatic image analysis of histopathology specimens using concave vertex graph,” in Proc. Med. Image Comput. Comput.-Assist. Interv., New York, NY, USA, 2008
[21] Reza Moradi Rad, Parvaneh Saeedi, Jason Au, and Jon Havelock “MULTI-RESOLUTIONAL ENSEMBLE OF STACKED DILATED U-NET FOR INNER CELL MASS SEGMENTATION IN HUMAN EMBRYONIC IMAGES” IEEE 2018
[22] X. Qi, F. Xing, D. J. Foran, and L. Yang, “Robust segmentation of overlapping cells in histopathology specimens using parallel seed detection and repulsive level set,” IEEE Trans. Biomed. Eng., vol. 59, no. 3, pp. 754–765, Mar. 2012.
[23] Humera Shaziya, K. Shyamala and Raniah Zaheer “Automatic Lung Segmentation on Thoracic CT Scans using U-Net Convolutional Network” International Conference on Communication and Signal Processing, IEEE 2018
[24] P. W. Huang and Y. H. Lai, “Effective segmentation and classification for HCC biopsy images,” Pattern Recog., vol. 43, no. 4, pp. 1550–1563, 2010.
[25] Dong Yang,Qiaoying Huang, Leon Axel, Dimitris Metaxas1 “MULTI-COMPONENT DEFORMABLE MODELS COUPLED WITH 2D-3D U-NET FOR AUTOMATED PROBABILISTIC SEGMENTATION OF CARDIAC WALLS AND BLOOD” IEEE 15th International Symposium on Biomedical Imaging 2018
[26] Lu Zhang,Li Xu “An Automatic Liver Segmentation Algorithm for CT Images U-net with separated paths of feature extraction” 3rd IEEE International Conference on Image, Vision and Computing 2018