Logistic Regression for Detection of Bankruptcy
Sagar Kumar1 , Shubhajit Mukherjee2 , Shubham Agrawal3 , Ila Chandrakar4
Section:Research Paper, Product Type: Journal Paper
Volume-07 ,
Issue-14 , Page no. 131-133, May-2019
CrossRef-DOI: https://doi.org/10.26438/ijcse/v7si14.131133
Online published on May 15, 2019
Copyright © Sagar Kumar, Shubhajit Mukherjee, Shubham Agrawal, Ila Chandrakar . This is an open access article distributed under the Creative Commons Attribution License, which permits unrestricted use, distribution, and reproduction in any medium, provided the original work is properly cited.
View this paper at Google Scholar | DPI Digital Library
How to Cite this Paper
- IEEE Citation
- MLA Citation
- APA Citation
- BibTex Citation
- RIS Citation
IEEE Style Citation: Sagar Kumar, Shubhajit Mukherjee, Shubham Agrawal, Ila Chandrakar, “Logistic Regression for Detection of Bankruptcy,” International Journal of Computer Sciences and Engineering, Vol.07, Issue.14, pp.131-133, 2019.
MLA Style Citation: Sagar Kumar, Shubhajit Mukherjee, Shubham Agrawal, Ila Chandrakar "Logistic Regression for Detection of Bankruptcy." International Journal of Computer Sciences and Engineering 07.14 (2019): 131-133.
APA Style Citation: Sagar Kumar, Shubhajit Mukherjee, Shubham Agrawal, Ila Chandrakar, (2019). Logistic Regression for Detection of Bankruptcy. International Journal of Computer Sciences and Engineering, 07(14), 131-133.
BibTex Style Citation:
@article{Kumar_2019,
author = {Sagar Kumar, Shubhajit Mukherjee, Shubham Agrawal, Ila Chandrakar},
title = {Logistic Regression for Detection of Bankruptcy},
journal = {International Journal of Computer Sciences and Engineering},
issue_date = {5 2019},
volume = {07},
Issue = {14},
month = {5},
year = {2019},
issn = {2347-2693},
pages = {131-133},
url = {https://www.ijcseonline.org/full_spl_paper_view.php?paper_id=1106},
doi = {https://doi.org/10.26438/ijcse/v7i14.131133}
publisher = {IJCSE, Indore, INDIA},
}
RIS Style Citation:
TY - JOUR
DO = {https://doi.org/10.26438/ijcse/v7i14.131133}
UR - https://www.ijcseonline.org/full_spl_paper_view.php?paper_id=1106
TI - Logistic Regression for Detection of Bankruptcy
T2 - International Journal of Computer Sciences and Engineering
AU - Sagar Kumar, Shubhajit Mukherjee, Shubham Agrawal, Ila Chandrakar
PY - 2019
DA - 2019/05/15
PB - IJCSE, Indore, INDIA
SP - 131-133
IS - 14
VL - 07
SN - 2347-2693
ER -
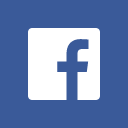
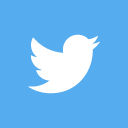
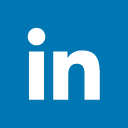
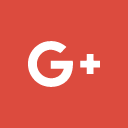
Abstract
Bankruptcy is a legal procedure that claims a person or organization as a debtor. It is essential to ascertain the risk of bankruptcy at initial stages to prevent financial losses. In this perspective, different soft computing techniques can be employed to ascertain bankruptcy. This study proposes a bankruptcy prediction system to categorize the companies based on extent of risk. The prediction system acts as a decision support tool for detection of bankruptcy
Key-Words / Index Term
Bankruptcy, soft computing, decision support tool
References
[1] Hauser, R.P. and Booth, D., “Predicting Bankruptcy with Robust Logistic Regression”, Journal of Data Science , Vol 9, pp. 565-584, 2011.
[2] Kim, M.-J. and Han, I. , ”The Discovery of Experts’ Decision Ruels from Qualitative Bankruptcy Data Using Genetic Algorithms”, Expert Systems with Application , Vol 25, pp. 637-646, 2003.
[3] Pedregosa, et al., “Scikit-Learn: Machine Learning in Python”, Journal of M achine Learning Research , Vol 12, pp. 2825-2830, 2011.
[4] Sirvastava, N., et al., “Dropout: A Simple Way to Prevent Neural Networks from Overfitting, “Journal of Machine Learning Research, Vol 15, 1929-1958, 2011.
[5] Dev, D., “Deep Learning with Hadoop”, Packet Publishing, Birmingham, 52, 2017.
[6] Nielsen, F., “Neural Networks—Algorithms and Applications”, https://www.mendeley.com/research-papers/neural-networks-algorithms-applicatio ns-5/
[7] Robinson, N., “The Disadvantages of Logistic Regression. http://classroom.synonym.com/disadvantages-logistic-regression-8574447.html “
[8] Sima, J. (1998) Introduction to Neural Networks. Technical Report No. 755.
[9] Baldi, P. (2012) Autoencoders, Unsupervised Learning, and Deep Architectures. Journal of Machine Learning Research , 27, 37-50.
[10] Martin, A., Uthayakumar, J. and Nadarajan, M. (2014) Qualitative Bankruptcy Data Set, UCI.
[11] Ramosacaj, Miftar & Hasani, Vjollca & Dumi, Alba. (2015). Application of Logistic Regression in the Study of Students’ Performance Level (Case Study of Vlora University). Journal of Educational and Social Research.
[12] Abedin, Tasnima & Chowdhury, Mohammad & Afzal, Arfan & F, Yeasmin & Turin, Tanvir. (2016). Application of Binary Logistic Regression in Clinical Research. Journal of National Heart Foundation of Bangladesh.