YOLO Based Object Detection Using Drone
Shiva Kumar R Naik1 , Kushal A2 , Lakshmi Narayan S3 , Sreeraam V Chatrapathi4 , Sagar T5
Section:Research Paper, Product Type: Journal Paper
Volume-07 ,
Issue-14 , Page no. 181-184, May-2019
CrossRef-DOI: https://doi.org/10.26438/ijcse/v7si14.181184
Online published on May 15, 2019
Copyright © Shiva Kumar R Naik, Kushal A, Lakshmi Narayan S, Sreeraam V Chatrapathi, Sagar T . This is an open access article distributed under the Creative Commons Attribution License, which permits unrestricted use, distribution, and reproduction in any medium, provided the original work is properly cited.
View this paper at Google Scholar | DPI Digital Library
How to Cite this Paper
- IEEE Citation
- MLA Citation
- APA Citation
- BibTex Citation
- RIS Citation
IEEE Style Citation: Shiva Kumar R Naik, Kushal A, Lakshmi Narayan S, Sreeraam V Chatrapathi, Sagar T, “YOLO Based Object Detection Using Drone,” International Journal of Computer Sciences and Engineering, Vol.07, Issue.14, pp.181-184, 2019.
MLA Style Citation: Shiva Kumar R Naik, Kushal A, Lakshmi Narayan S, Sreeraam V Chatrapathi, Sagar T "YOLO Based Object Detection Using Drone." International Journal of Computer Sciences and Engineering 07.14 (2019): 181-184.
APA Style Citation: Shiva Kumar R Naik, Kushal A, Lakshmi Narayan S, Sreeraam V Chatrapathi, Sagar T, (2019). YOLO Based Object Detection Using Drone. International Journal of Computer Sciences and Engineering, 07(14), 181-184.
BibTex Style Citation:
@article{Naik_2019,
author = {Shiva Kumar R Naik, Kushal A, Lakshmi Narayan S, Sreeraam V Chatrapathi, Sagar T},
title = {YOLO Based Object Detection Using Drone},
journal = {International Journal of Computer Sciences and Engineering},
issue_date = {5 2019},
volume = {07},
Issue = {14},
month = {5},
year = {2019},
issn = {2347-2693},
pages = {181-184},
url = {https://www.ijcseonline.org/full_spl_paper_view.php?paper_id=1116},
doi = {https://doi.org/10.26438/ijcse/v7i14.181184}
publisher = {IJCSE, Indore, INDIA},
}
RIS Style Citation:
TY - JOUR
DO = {https://doi.org/10.26438/ijcse/v7i14.181184}
UR - https://www.ijcseonline.org/full_spl_paper_view.php?paper_id=1116
TI - YOLO Based Object Detection Using Drone
T2 - International Journal of Computer Sciences and Engineering
AU - Shiva Kumar R Naik, Kushal A, Lakshmi Narayan S, Sreeraam V Chatrapathi, Sagar T
PY - 2019
DA - 2019/05/15
PB - IJCSE, Indore, INDIA
SP - 181-184
IS - 14
VL - 07
SN - 2347-2693
ER -
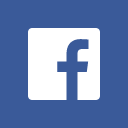
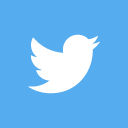
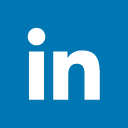
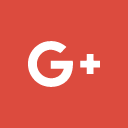
Abstract
The headway of convolutional neural systems (CNNs) and Deep learning (DL) in the previous decade brought about significant upgrades in computer vision. One of the recipients of these advances is the task of object detection, where the goal is to distinguish and locate real-world objects inside pictures or videos. Real-time object tracking on a drone under a dynamic situation has been a difficult issue for a long time, with existing methodologies utilizing off-line calculation or powerful computation units on board. This paper displays lightweight real-time on board object tracking methodology, which varies, from basic image classification in that the AI demonstrate needs to distinguish numerous objects in a single frame, and figure out where these objects are found. The advances in procedures, joined with the improved PC equipment, put real-time object detection well inside the capacities of present day processors. Real-time object recognition is essential for some utilization of Unmanned Aerial Vehicles (UAVs), for example, observation and reconnaissance, search-and-rescue, and foundation assessment. In the previous couple of years, Convolutional Neural Networks (CNNs) have ascended as an unbelievable class of models for recognizing picture content, and are seen as the standard strategy for generally issues.
Key-Words / Index Term
Detection, Drone, Pattern Matching, Privacy Preserving, Security Vulnerabilities, Sensitive Items, Yolo
References
[1]“N.H.Motlagh, T.Taleb, and O.Arouk,”Low-altitude unmanned aerial vehicle-based internet of things services: Comprehensivesurvey and futurePerspectives,”IEEE internet of Things Journal, Vol.3, no.6, Dec.2016”
[2]“Arvind and Rishiyur S. Nikhil. Executing a program on the MIT tagged-token dataflow architecture. IEEE Trans. Comput., 39(3):300–318, 1990.”
[3]“Jack Clark. Google turning its lucrative web search over to AI machines, 2015.”
[4]“Sherrah, J. Fully Convolutional Networks for Dense Semantic Labelling of High-Resolution Aerial Imagery. Available online: https://arxiv.org/pdf/1606.02585.pdf (accessed on 8 June 2017).”
[5]“Sermanet, P.; Eigen, D.; Zhang, X.; Mathieu, M.; Fergus, R.; LeCun, Y. Overfeat: Integrated Recognition, Localization and Detection Using Convolutional Networks. Available online: https://arxiv.org/pdf/1312.6229.pdf (accessed on 14 June 2017).”
[6] “T. P. Breckon, S. E. Barnes, M. L. Eichner, and K. Wahren, “Autonomous real-time vehicle detection from a medium-level UAV,” in Proc. 24th International Conference on Unmanned Air Vehicle Systems, pp. 29.1-29.9, 2009”
[7] “M. Bhaskaranand, and J. D. Gibson, “Low-complexity video encoding for UAV reconnaissance and surveillance,” in Proc. IEEE Military Communications Conference (MILCOM), pp. 1633-1638, 2011.”