Machine Learning Approach for Cancer Detection
Anooja Ali1 , Pooja G2 , Prajeela MP3 , Riddhi Rakesh4 , Tabassum Taj5
Section:Research Paper, Product Type: Journal Paper
Volume-07 ,
Issue-14 , Page no. 224-228, May-2019
CrossRef-DOI: https://doi.org/10.26438/ijcse/v7si14.224228
Online published on May 15, 2019
Copyright © Anooja Ali, Pooja G, Prajeela MP, Riddhi Rakesh, Tabassum Taj . This is an open access article distributed under the Creative Commons Attribution License, which permits unrestricted use, distribution, and reproduction in any medium, provided the original work is properly cited.
View this paper at Google Scholar | DPI Digital Library
How to Cite this Paper
- IEEE Citation
- MLA Citation
- APA Citation
- BibTex Citation
- RIS Citation
IEEE Style Citation: Anooja Ali, Pooja G, Prajeela MP, Riddhi Rakesh, Tabassum Taj, “Machine Learning Approach for Cancer Detection,” International Journal of Computer Sciences and Engineering, Vol.07, Issue.14, pp.224-228, 2019.
MLA Style Citation: Anooja Ali, Pooja G, Prajeela MP, Riddhi Rakesh, Tabassum Taj "Machine Learning Approach for Cancer Detection." International Journal of Computer Sciences and Engineering 07.14 (2019): 224-228.
APA Style Citation: Anooja Ali, Pooja G, Prajeela MP, Riddhi Rakesh, Tabassum Taj, (2019). Machine Learning Approach for Cancer Detection. International Journal of Computer Sciences and Engineering, 07(14), 224-228.
BibTex Style Citation:
@article{Ali_2019,
author = {Anooja Ali, Pooja G, Prajeela MP, Riddhi Rakesh, Tabassum Taj},
title = {Machine Learning Approach for Cancer Detection},
journal = {International Journal of Computer Sciences and Engineering},
issue_date = {5 2019},
volume = {07},
Issue = {14},
month = {5},
year = {2019},
issn = {2347-2693},
pages = {224-228},
url = {https://www.ijcseonline.org/full_spl_paper_view.php?paper_id=1126},
doi = {https://doi.org/10.26438/ijcse/v7i14.224228}
publisher = {IJCSE, Indore, INDIA},
}
RIS Style Citation:
TY - JOUR
DO = {https://doi.org/10.26438/ijcse/v7i14.224228}
UR - https://www.ijcseonline.org/full_spl_paper_view.php?paper_id=1126
TI - Machine Learning Approach for Cancer Detection
T2 - International Journal of Computer Sciences and Engineering
AU - Anooja Ali, Pooja G, Prajeela MP, Riddhi Rakesh, Tabassum Taj
PY - 2019
DA - 2019/05/15
PB - IJCSE, Indore, INDIA
SP - 224-228
IS - 14
VL - 07
SN - 2347-2693
ER -
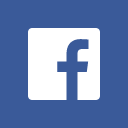
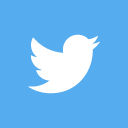
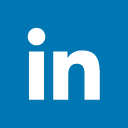
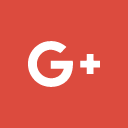
Abstract
Machine Learning has several applications in Healthcare Domain. It provides more efficient, faster, smarter ways to detect and cure various diseases. Machine learning approaches are widely used for cancer diagnosis. In our approach we classify cancerous and noncancerous Oral Cancer images. We focused on image pre-processing, segmentation using image segmentation app in Matlab to improve the image quality and thereby improving the accuracy of classification and cancer detection. This approach using Support Vector Machines (SVM) obtained an accuracy of 89.2%. This method can be easily adopted for early cancer detection.
Key-Words / Index Term
Machine Learning,Oral Cancer, Image Segmentation, Support Vector Machines
References
[1] Van Dyck, &, W Stremersch S, “Marketing of the LifeSciences: A New Framework and Research Agenda for a Nascent Field”. Journal of Marketing, 73(4), 430, 2009
[2] Rowlands, S., Coverdale, S., & Callen, J, “Documentation of clinical care in hospital patients’ medical records: A qualitative study of medical students’ perspectives on clinical documentation education”. Health Information Management Journal, 45(3), 99–106, 2016
[3] Lenzer, Jeanne, “Claim that smallpox vaccine protects against HIV is premature, say critics.” BMJ (Clinical research ed.) vol. 327,7417: 699. doi:10.1136 /bmj.327.7417.699, 2003
[4] F., Abdolali, R. A., Zoroofi, Y., Otake, &Y., Sato, “Automated classification of maxillofacial cysts in cone beam CT images using contour less transformation and Spherical Harmonics,” Computer Methods and Programs in Biomedicine, 139, 197-207, 2017.
[5] Early stage oral cavity cancer detection: Anisotropic pre-processing and fuzzy C-means segmentation 2018 IEEE 8th Annual Computing and Communication Workshop and Conference, CCWC 2018, Volumes 2018-January, 2018
.[6] T, Karthikeyan, “Unified RF-SVM model based digital radiography classification for Inferior Alveolar Nerve Injury (IANA) identification,” Biomedical Research, 27(4), 2016.
[7] Amin, Javeria, et al. "A distinctive approach in brain tumor detection and classification using MRI." Pattern Recognition Letters ,2017.
[8] S.-W.,Chang, S., Abdul-Kareem, A.F.,Merican, & R. B. , Zain, “ Oral cancer prognosis based on clinicopathologic and genomic markers using a hybrid of feature selection and machine learning methods,” BMC Bioinformatics, 14(1), 2013.
[9] S., Galib, F., Islam, M., Abir& H.-K. , Lee, “Computer aided detection of oral lesions on CT images,” Journal of Instrumentation, 10(12), 2015.
[10] M. M. R., Krishnan, P., Shah, C. Chakraborty, & A. K., Ray, “Statistical analysis of textual features for improved classification of oral histopathological images,” Journal of medical systems, 36(2) , 2012.
[11] I., Nurtanio, E. R., Astuti, I. K. E., Purnama, M., Hariadi, & M. H. , Purnomo, “ Classifying cyst and tumor lesion using support vector machine based on dental panoramic images texture features,” IAENG International Journal of Computer Science, 40(1), 29-37, 2013.
[12] K., Nguyen, A. K., Jain, & R. L., Allen,” Automated Gland Segmentation and Classification for Gleason Grading of Prostate Tissue Images,” 20th International Conference on Pattern Recognition, 23-26, 2010.
[13] N. P., Malek, S., Schmidt, P., Huber, M. P., Manns,T.F.Greten, “The diagnosis and treatment of hepatocellular carcinoma,” Alcohol, 20, 2014.