Classification of land cover using Data Analytics for Hyperspectral Imaging
Sakshi Suman1 , Sanjana Suman2 , Santhosh J3 , Sham Vignesh4 , K Anitha5
Section:Research Paper, Product Type: Journal Paper
Volume-07 ,
Issue-14 , Page no. 345-348, May-2019
CrossRef-DOI: https://doi.org/10.26438/ijcse/v7si14.345348
Online published on May 15, 2019
Copyright © Sakshi Suman, Sanjana Suman, Santhosh J, Sham Vignesh, K Anitha . This is an open access article distributed under the Creative Commons Attribution License, which permits unrestricted use, distribution, and reproduction in any medium, provided the original work is properly cited.
View this paper at Google Scholar | DPI Digital Library
How to Cite this Paper
- IEEE Citation
- MLA Citation
- APA Citation
- BibTex Citation
- RIS Citation
IEEE Style Citation: Sakshi Suman, Sanjana Suman, Santhosh J, Sham Vignesh, K Anitha, “Classification of land cover using Data Analytics for Hyperspectral Imaging,” International Journal of Computer Sciences and Engineering, Vol.07, Issue.14, pp.345-348, 2019.
MLA Style Citation: Sakshi Suman, Sanjana Suman, Santhosh J, Sham Vignesh, K Anitha "Classification of land cover using Data Analytics for Hyperspectral Imaging." International Journal of Computer Sciences and Engineering 07.14 (2019): 345-348.
APA Style Citation: Sakshi Suman, Sanjana Suman, Santhosh J, Sham Vignesh, K Anitha, (2019). Classification of land cover using Data Analytics for Hyperspectral Imaging. International Journal of Computer Sciences and Engineering, 07(14), 345-348.
BibTex Style Citation:
@article{Suman_2019,
author = {Sakshi Suman, Sanjana Suman, Santhosh J, Sham Vignesh, K Anitha},
title = {Classification of land cover using Data Analytics for Hyperspectral Imaging},
journal = {International Journal of Computer Sciences and Engineering},
issue_date = {5 2019},
volume = {07},
Issue = {14},
month = {5},
year = {2019},
issn = {2347-2693},
pages = {345-348},
url = {https://www.ijcseonline.org/full_spl_paper_view.php?paper_id=1150},
doi = {https://doi.org/10.26438/ijcse/v7i14.345348}
publisher = {IJCSE, Indore, INDIA},
}
RIS Style Citation:
TY - JOUR
DO = {https://doi.org/10.26438/ijcse/v7i14.345348}
UR - https://www.ijcseonline.org/full_spl_paper_view.php?paper_id=1150
TI - Classification of land cover using Data Analytics for Hyperspectral Imaging
T2 - International Journal of Computer Sciences and Engineering
AU - Sakshi Suman, Sanjana Suman, Santhosh J, Sham Vignesh, K Anitha
PY - 2019
DA - 2019/05/15
PB - IJCSE, Indore, INDIA
SP - 345-348
IS - 14
VL - 07
SN - 2347-2693
ER -
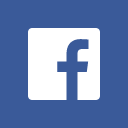
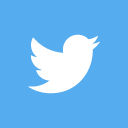
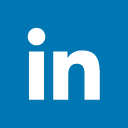
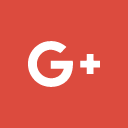
Abstract
Recent advances in remote sensing technology have made hyperspectral data with hundreds of narrow contiguous bands more widely available. The hyperspectral data can, therefore, reveal narrow differences in the spectral signatures of land cover classes that appear to be similar when viewed by multispectral sensors. If successfully used, the hyperspectral data can yield higher classification accuracies and more detailed class taxonomies. In this approach, we are using deep learning and neural networks to train a model for classifying land cover using data analytics in hyperspectral imaging.
Key-Words / Index Term
Hyperspectral imaging, Land cover classification, Deep learning, Tensor flow
References
[1]. “Hyperspectral Image Analysis using Deep Learning”- a Review Henrik Petersson, David Gustafsson and David Bergström Swedish Defence Research Agency (FOI)Division of C4ISR
[2]. Yushi Chen, Zhouhan Lin, Xing Zhao, Gang Wang, and Yanfeng Gu. “Deep learning-based classification of hyperspectral data”. Selected Topics in Applied Earth Observations and Remote Sensing, IEEE Journal of, 7(6):2094–2107, June 2014
[3]. “Random N-Finder (N-FINDR) Endmember Extraction Algorithms for Hyperspectral Imagery” Chein-I Chang, Fellow, IEEE, Chao-Cheng Wu, Member, IEEE, and Ching-Tsorng Tsai
[4]. N. Keshava and J. Mustard, “Spectral unmixing,” IEEE Signal Process. Mag., vol. 19, no. 1, pp. 44–57, Jan. 2002
[5]. M.Winter, “Fast autonomous spectral end-member determination in hyperspectral data,” in Proc. 13th Int. Conf. on Applied Geologic Remote Sensing, vol. 2, Vancouver, pp. 337–344, Apr. 1999.
[6]. J. M. Nascimento and J. M. Bioucas-Dias, “Vertex component analysis: A fast algorithm to unmix hyperspectral data,” IEEE Trans. Geosci. Remote Sens., vol. 43, no. 4, pp. 898–910, Apr. 2005.
[7]. N. Dobigeon, S. Moussaoui, M. Coulon, J. Y. Tourneret and A. O. Hero, “Joint Bayesian endmember extraction and linear unmixing for hyperspectral imagery,” IEEE Trans. Signal Process., vol. 57, no. 11, pp. 4355–4368, Nov. 2009.
[8]. Makantasis, K.; Doulamis, A.; Doulamis, N.; Nikitakis, “A. Tensor-based classification models for hyperspectral data analysis”. IEEE Trans. Geosci. Remote Sens. 2018, 56, 1–15. [CrossRef]
[9]. Zhang, M.; Li, W.; Du, Q. “Diverse region-based CNN for hyperspectral image classification”. IEEE Trans. Image Process. 2018, 27, 2623–2634. [CrossRef]
[10]. http://www.escience.cn/people/feiyunZHU/Dataset_GT.html the website for dataset and ground truth.
[11]. “TensorFlow: A System for Large-Scale Machine Learning” Martín Abadi, Paul Barham, Jianmin Chen, Zhifeng Chen, Andy Davis, Jeffrey Dean,Matthieu Devin, Sanjay Ghemawat, Geoffrey Irving, Michael Isard, Manjunath Kudlur,Josh Levenberg, Rajat Monga, Sherry Moore, Derek G. Murray, Benoit Steiner, Paul Tucker,Vijay Vasudevan, Pete Warden, Martin Wicke, Yuan Yu, and Xiaoqiang Zheng, Google Brain