Prediction of Heart Disease with Claims Data using Machine Learning Method
avanya L1 , Megha V2 , Nagashree H3 , Pavithra S4 , Anusha K L5
Section:Research Paper, Product Type: Journal Paper
Volume-07 ,
Issue-15 , Page no. 65-68, May-2019
CrossRef-DOI: https://doi.org/10.26438/ijcse/v7si15.6568
Online published on May 16, 2019
Copyright © Lavanya L, Megha V, Nagashree H, Pavithra S, Anusha K L . This is an open access article distributed under the Creative Commons Attribution License, which permits unrestricted use, distribution, and reproduction in any medium, provided the original work is properly cited.
View this paper at Google Scholar | DPI Digital Library
How to Cite this Paper
- IEEE Citation
- MLA Citation
- APA Citation
- BibTex Citation
- RIS Citation
IEEE Citation
IEEE Style Citation: Lavanya L, Megha V, Nagashree H, Pavithra S, Anusha K L, “Prediction of Heart Disease with Claims Data using Machine Learning Method,” International Journal of Computer Sciences and Engineering, Vol.07, Issue.15, pp.65-68, 2019.
MLA Citation
MLA Style Citation: Lavanya L, Megha V, Nagashree H, Pavithra S, Anusha K L "Prediction of Heart Disease with Claims Data using Machine Learning Method." International Journal of Computer Sciences and Engineering 07.15 (2019): 65-68.
APA Citation
APA Style Citation: Lavanya L, Megha V, Nagashree H, Pavithra S, Anusha K L, (2019). Prediction of Heart Disease with Claims Data using Machine Learning Method. International Journal of Computer Sciences and Engineering, 07(15), 65-68.
BibTex Citation
BibTex Style Citation:
@article{L_2019,
author = {Lavanya L, Megha V, Nagashree H, Pavithra S, Anusha K L},
title = {Prediction of Heart Disease with Claims Data using Machine Learning Method},
journal = {International Journal of Computer Sciences and Engineering},
issue_date = {5 2019},
volume = {07},
Issue = {15},
month = {5},
year = {2019},
issn = {2347-2693},
pages = {65-68},
url = {https://www.ijcseonline.org/full_spl_paper_view.php?paper_id=1202},
doi = {https://doi.org/10.26438/ijcse/v7i15.6568}
publisher = {IJCSE, Indore, INDIA},
}
RIS Citation
RIS Style Citation:
TY - JOUR
DO = {https://doi.org/10.26438/ijcse/v7i15.6568}
UR - https://www.ijcseonline.org/full_spl_paper_view.php?paper_id=1202
TI - Prediction of Heart Disease with Claims Data using Machine Learning Method
T2 - International Journal of Computer Sciences and Engineering
AU - Lavanya L, Megha V, Nagashree H, Pavithra S, Anusha K L
PY - 2019
DA - 2019/05/16
PB - IJCSE, Indore, INDIA
SP - 65-68
IS - 15
VL - 07
SN - 2347-2693
ER -
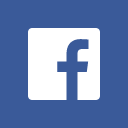
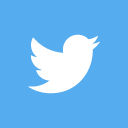
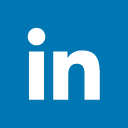
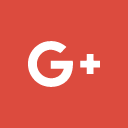
Abstract
Machine learning can be referred as discovery of relationships in larger datasets and in some cases it is used for predicting relationships based on the results discovered. Nowadays machine learning is achieving widespread in various fields such as healthcare industry, scientific and engineering. In healthcare industry, machine learning is mainly used for disease prediction. The main objective of our work is to predict heart disease using Naïve Bayes classifier. Naïve Bayes are the probabilistic classifiers used to classify the data using attributes. It retrieves the trained data and compares the attribute values with test data sets and predicts the result.
Key-Words / Index Term
Machine learning, prediction, healthcare industry, Naïve Bayes Classifier, Heartdisease
References
[1] E. Choi, M.T.Bahadori, L.Song, W.F. Stewart, and J.Sun, “Gram: Graph- based attention model for health acre representation learning,” in proceedings of the 23rd ACMSIGKDD International Conference on Knowledge Discovery and Data Mining, ser. KDD ’17. New York,NY, USA:ACM, pp 787-795.
[2] D. Kartchner, T. Chirstensen, J. Humphreys, and S.Wade, “Code2vec: Embedding and clustering medical diagosis data,” in 2017 IEEE International Conference on Healthcare Informatics (ICHI), Aug 2017, pp. 386-390.
[3] R. Miotto, L. Li, B. A. Kidd, and J.Dudley, “Deep patient: An unsupervised representation to predict the future of patients from the electronic health records,” in Scientific reports, 2016
[4] Z. Che, Y. Cheng, S. Zhai, Z.Sun and Y.Liu, “Boosting deep learning risk prediction with generative adversarial netwoks for electronic health records, ” in 2017 IEEE International Conference on Data Mining (ICDM) , Nov 2017, pp. 787-792.
[5]. R.Snyderman, “Personalized health care: from theory to practice,” Biotechnology Journal, vol. 7, no. 8, pp. 973-979, Aug. 2012
[6] N.Razavian and D. Sontag, “Temporal convolution neural networks for diagnosis from lab tests,” CoRR, vol. abs/1511.07938, 2015.
[7] A. Perotte, R. Ranganath, J.S. Hirsch, D. Blei, and N.Elthadad, “Risk prediction forchronic kidney disease progression using heterogenous electronic helath record data and tie series analysis,” Journal of the American Medical Informatics Association: JAMLA, vol. 22, no. 4, pp. 872-880, Jul. 2015.
[8] N. Tangri, L.A. Stevens, J, Griffith, H. Tghiouart, O.Djurdjev, D. Naimark, A. Levin, and A.S levey, “A Predictive model for progression of chronic kidney disease to kidney failure,” Jama, vol.305, no.15, pp. 1553-1559, 2011.
[9] E. Choi, M.T. Bahadori, A Schuetz , W. F. Stewart, and J. Sun “Doctor ai: Predicting clinical events via recurrent neural networks ,” in Proceedings of the !st Machine Learning for Healthcare Conferences ser. Proceedings of Machine Learning Research, F. Doshi-Velez, J. Fackler, D.Kale, B. Wallace, and J.Wiens, Eds., vol.56. Children’s Hospital LA, Los Angeles, CA, USA: PMLR, 18-1, Aug 2016, pp.301-318
[10] M.Khalilia, S. Chakraborty, and M. Popescu, “Predicting disease risks from highly imbalanced data using random forest,” BMC medical informatics and decision making, vol.11, no. 1, p. 1,2011.
[11] X.Wang, D. Sontag, and F.Wang, “Unspervised learning of disease progression models,” in Proceedings of the 20th ACM SIGKDD International Conference on knowledge discovery and data mining. ACM, 2014, pp. 85-94.
[12] Z.C. Lipton, D.C. Kale, C.Elkan, and R. Wetzell, “Learning to diagnose with lstm recurrent neural networks,” arXiv preprint arXiv: 1511. 03677, 2015
[13]N.Barakat, A.P.Bradley, and M.N.H. Barakat, “Intelligible support vector Machine for Diagnosis of Diabetes Mellitus,” IEEE Transactions on Information Technology in Biomedicine, vol. 14, no, pp.1114- 1120, Jul. 2010.
[14] “Prediction Modelling Using HER data: Challenges, Strategies Medical Carehhtp://journals.lww.com/lwwmedcalcare/Fulltext/2010/06001/Prediction Modeling Using EHR data Challenges,.17.aspx.
[15] W. Yu, T. Liu, R. Valdez, M. Gwinn, and M.J. Khoury, “Application of support vector machine modelling for prediction of common disease: the case of diabetes and pre-diabetes,” BMc Medical Informatics and Decision Making, vol. 10, p. 16, 2010.
[16] A. V. Lebedev, E, Westman, G.J. P. Van Western, M.G. Kramberger, A. Lundervold, D, Aarsland, H. Soininen, I. Kloszewska, P. Mecocci, M. Tsolaki, B. Vellas, S. Lovestone, And A. Simmons, “Random Forest ensembles for detection and prediction of Alzheimer’s disease with good between- cohort robustness,” NeuroImage: Clinical, vol.6, pp. 115-125, 2014