Sentiment Analysis of Twitter Data Using Text Classification And Clustering
Kumari Ashmita Sinha1 , Maanasa VKP2 , Nikhitha SP3 , Ramya S4 , Mangala CN5
Section:Research Paper, Product Type: Journal Paper
Volume-07 ,
Issue-15 , Page no. 69-72, May-2019
CrossRef-DOI: https://doi.org/10.26438/ijcse/v7si15.6972
Online published on May 16, 2019
Copyright © Kumari Ashmita Sinha, Maanasa VKP, Nikhitha SP, Ramya S, Mangala CN . This is an open access article distributed under the Creative Commons Attribution License, which permits unrestricted use, distribution, and reproduction in any medium, provided the original work is properly cited.
View this paper at Google Scholar | DPI Digital Library
How to Cite this Paper
- IEEE Citation
- MLA Citation
- APA Citation
- BibTex Citation
- RIS Citation
IEEE Citation
IEEE Style Citation: Kumari Ashmita Sinha, Maanasa VKP, Nikhitha SP, Ramya S, Mangala CN, “Sentiment Analysis of Twitter Data Using Text Classification And Clustering,” International Journal of Computer Sciences and Engineering, Vol.07, Issue.15, pp.69-72, 2019.
MLA Citation
MLA Style Citation: Kumari Ashmita Sinha, Maanasa VKP, Nikhitha SP, Ramya S, Mangala CN "Sentiment Analysis of Twitter Data Using Text Classification And Clustering." International Journal of Computer Sciences and Engineering 07.15 (2019): 69-72.
APA Citation
APA Style Citation: Kumari Ashmita Sinha, Maanasa VKP, Nikhitha SP, Ramya S, Mangala CN, (2019). Sentiment Analysis of Twitter Data Using Text Classification And Clustering. International Journal of Computer Sciences and Engineering, 07(15), 69-72.
BibTex Citation
BibTex Style Citation:
@article{Sinha_2019,
author = {Kumari Ashmita Sinha, Maanasa VKP, Nikhitha SP, Ramya S, Mangala CN},
title = {Sentiment Analysis of Twitter Data Using Text Classification And Clustering},
journal = {International Journal of Computer Sciences and Engineering},
issue_date = {5 2019},
volume = {07},
Issue = {15},
month = {5},
year = {2019},
issn = {2347-2693},
pages = {69-72},
url = {https://www.ijcseonline.org/full_spl_paper_view.php?paper_id=1203},
doi = {https://doi.org/10.26438/ijcse/v7i15.6972}
publisher = {IJCSE, Indore, INDIA},
}
RIS Citation
RIS Style Citation:
TY - JOUR
DO = {https://doi.org/10.26438/ijcse/v7i15.6972}
UR - https://www.ijcseonline.org/full_spl_paper_view.php?paper_id=1203
TI - Sentiment Analysis of Twitter Data Using Text Classification And Clustering
T2 - International Journal of Computer Sciences and Engineering
AU - Kumari Ashmita Sinha, Maanasa VKP, Nikhitha SP, Ramya S, Mangala CN
PY - 2019
DA - 2019/05/16
PB - IJCSE, Indore, INDIA
SP - 69-72
IS - 15
VL - 07
SN - 2347-2693
ER -
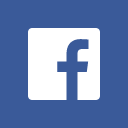
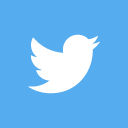
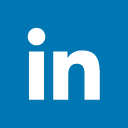
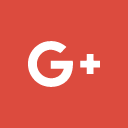
Abstract
Information and data has always been consideredas the lifeline to run any business. The stability of an organization, the growth of the business, the profit gained or the loss suffered, all these factors depend on the information gained about the market trends and also most importantly public opinion. The sentiments of the people are therefore considered as the most crucial data that the organizations use in order to take effective decisions with minimum risk.In this paper we gather data from a type of social media that is twitter. This is because the public nowadays express their opinions and their feedbacks largely to through social media. In this paper we attempt to perform sentiment analysis using text classification by Naïve Bayes and text Clustering by K-means.
Key-Words / Index Term
Twitter, Sentiment Analysis, Social Media, Naïve Bayes, K-means
References
[1] “Application of text classification and clustering of Twitter data for business analytics”, Alrence Santiago Halibas ; Abubucker Samsudeen Shaffi ; Mohamed Abdul Kader Varusai Mohamed,2018 Majan International Conference (MIC), 2018.
[2] “Text Analytics Market by Component (Software, Services), Application (Customer Experience Management, Marketing Management, Governance, Risk and Compliance Management), Deployment Model, Organization Size, Industry Vertical, Region - Global Forecast to 20,” 2017.
[3] H. S. Yaram, “Machine learning algorithms for document clustering and fraud detection,” in Proceedings of the 2016 International Conference on Data Science and Engineering, ICDSE 2016, 2017.
[4] L. P. Khobragade and V. Jethani, “Sentiment Analysis of Movie Review,”
Int. J. Adv. Res. Comput. Sci., vol. 8, no. 5, p. 19411948, 2017.
[5] R. A. Jain and P. Dandannavar, “text analytics framework using apache spark and combination of lexical and maching learning techniques,” Int.
J. Bus. Anal. Intell., vol. 5, no. 1, pp. 36–42, 2017.
[6]S.M. Allahyari et al., “A Brief Survey of Text Mining: Classification, Clustering and Extraction Techniques,” arXiv Prepr. arXiv, vol. 1707, no. 2919, pp. 1–13, 2017.
[7] V. K. S. Rawat, “Comparative Analysis of Data Mining Techniques, Tools and Maching Learning Algorithms for Efficient Data Analytics,” JOSR J. Comput. Eng., vol. 19, no. 4, pp. 56–60, 2017.
[8] P. Gonçalves and M. Araújo, “Comparing and combining sentiment analysis methods,” Proc. first ACM Conf. Online Soc. networks. ACM, pp. 27–38, 2013.
[9] W. H. Kaur and V. Mangat, “Dictionary based Sentiment Analysis of Hinglish text,” Int. J. Adv. Res. Comput. Sci., vol. 8, no. 5, pp. 816–822, 2017.
[10] C. F. N. Ribeiro, M. Araújo, P. Gonçalves, M. André Gonçalves, and F. Benevenuto, “SentiBench - a benchmark comparison of state-of-the- practice sentiment analysis methods,” EPJ Data Sci., vol. 5, no. 1, 2016.
[11] D. A. Moreno and T. Redondo, “Text Analytics: the convergence of Big Data and Artificial Intelligence,” Int. J. Interact. Multimed. Artif. Intell., vol. 3, no. 6, p. 57, 2016.
[12] E. V. A. Kharde and S. S. Sonawane, “Sentiment Analysis of Twitter Data: A Survey of Techniques,” Int. J. Comput. Appl., vol. 139, no. 11, pp. 975–8887, 2016.
[13] G. L. Ziora, “The sentiment analysis as a tool of business analytics in contemporary organizations,” Stud. Ekon., pp. 234–241, 2016.
[14] K. O. Muller, I. Junglas, S. Debortoli, and J. Von Brocke, “Using Text Analytics to Derive Customer Service Management Benefits from Unstructured Data,” MIS Q. Exec., vol. 15, no. 4, pp. 64–73, 2016.
[15] T. A. Trevino, “Introduction to K-means Clustering,” Datascience.com. 2016.
[16] X. M. H. Peetz, M. De Rijke, and R. Kaptein, “Estimating Reputation Polarity on Microblog Posts,” Inf. Process. Manag., vol. 52, no. 2, pp. 193–216, 2016.
[17] I. N. Yussupova, M. Boyko, and D. Bogdanova, “A Decision Support Approach based on Sentiment Analysis Combined with Data Mining for Customer Satisfaction Research,” Int. J. Adv. Intell. Syst., vol. 1&2, 2015.
[18] J. S. K. Markham, M. Kowolenko, and T. L. Michaelis, “Unstructured Text Analytics to Support New Product Development Decisions,” Res. Technol. Manag., vol. 58, no. 2, pp. 30–39, 2015..
[19] F. W. Medhat, A. Hassan, and H. Korashy, “Sentiment analysis algorithms and applications: A survey,” Ain Shams Eng. J., vol. 5, no. 4, pp. 1093– 1113, 2014.
[20] U. M. Hofmann; and R. Klinkenberg;, “RapidMiner: Data Mining Use Cases and Business Analytics Applications,” Zhurnal Eksp. i Teor. Fiz., 2013.