An Innovative Dashboard to Order Images with Respect to Shuffled and Smile Frequencies
Nisha Prakash1 , Niveditha G2 , Poojitha M3 , Rakshitha H D4 , Swetha N5
Section:Research Paper, Product Type: Journal Paper
Volume-07 ,
Issue-15 , Page no. 85-89, May-2019
CrossRef-DOI: https://doi.org/10.26438/ijcse/v7si15.8589
Online published on May 16, 2019
Copyright © Nisha Prakash, Niveditha G, Poojitha M, Rakshitha H D, Swetha N . This is an open access article distributed under the Creative Commons Attribution License, which permits unrestricted use, distribution, and reproduction in any medium, provided the original work is properly cited.
View this paper at Google Scholar | DPI Digital Library
How to Cite this Paper
- IEEE Citation
- MLA Citation
- APA Citation
- BibTex Citation
- RIS Citation
IEEE Style Citation: Nisha Prakash, Niveditha G, Poojitha M, Rakshitha H D, Swetha N, “An Innovative Dashboard to Order Images with Respect to Shuffled and Smile Frequencies,” International Journal of Computer Sciences and Engineering, Vol.07, Issue.15, pp.85-89, 2019.
MLA Style Citation: Nisha Prakash, Niveditha G, Poojitha M, Rakshitha H D, Swetha N "An Innovative Dashboard to Order Images with Respect to Shuffled and Smile Frequencies." International Journal of Computer Sciences and Engineering 07.15 (2019): 85-89.
APA Style Citation: Nisha Prakash, Niveditha G, Poojitha M, Rakshitha H D, Swetha N, (2019). An Innovative Dashboard to Order Images with Respect to Shuffled and Smile Frequencies. International Journal of Computer Sciences and Engineering, 07(15), 85-89.
BibTex Style Citation:
@article{Prakash_2019,
author = {Nisha Prakash, Niveditha G, Poojitha M, Rakshitha H D, Swetha N},
title = {An Innovative Dashboard to Order Images with Respect to Shuffled and Smile Frequencies},
journal = {International Journal of Computer Sciences and Engineering},
issue_date = {5 2019},
volume = {07},
Issue = {15},
month = {5},
year = {2019},
issn = {2347-2693},
pages = {85-89},
url = {https://www.ijcseonline.org/full_spl_paper_view.php?paper_id=1206},
doi = {https://doi.org/10.26438/ijcse/v7i15.8589}
publisher = {IJCSE, Indore, INDIA},
}
RIS Style Citation:
TY - JOUR
DO = {https://doi.org/10.26438/ijcse/v7i15.8589}
UR - https://www.ijcseonline.org/full_spl_paper_view.php?paper_id=1206
TI - An Innovative Dashboard to Order Images with Respect to Shuffled and Smile Frequencies
T2 - International Journal of Computer Sciences and Engineering
AU - Nisha Prakash, Niveditha G, Poojitha M, Rakshitha H D, Swetha N
PY - 2019
DA - 2019/05/16
PB - IJCSE, Indore, INDIA
SP - 85-89
IS - 15
VL - 07
SN - 2347-2693
ER -
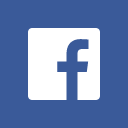
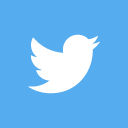
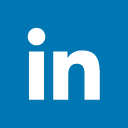
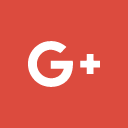
Abstract
A principled method to manage, uncover the structure of visual data by understanding significant learning task initiated by visual stage learning is presented. The target of this task is to find the phase that recovers the structure of data from revamped types of it. By virtue of standard pictures, this task comes down to recovering the primary picture from patches improved by a dark change and organised. Stage grids are discrete in this way introduces inconveniences for slant based streamlining techniques. To this end, we resort to a perpetual gauge using doubly-stochastic cross sections and define a novel bi-level streamlining issue on such systems that makes sense of how to recover the change. Such a plan prompts costly inclination calculations. We go around this issue by further proposing a computationally shoddy pattern for producing doubly stochastic frameworks dependent on PCA and DWT. The utility is exhibited on three testing PC vision issues, to be specific, relative traits learning, managed figuring out how to rank and self-directed portrayal learning. Our outcome shows condition of the craftsmanship execution on the open figure and osr benchmarks for relative qualities.
Key-Words / Index Term
visual permutation learning, PCA, DWT, CNN
References
[1] A. H. Abdulnabi, G. Wang, J. Lu, and K. Jia, “Multi-task cnn model for attribute prediction,” IEEE Transactions on Multimedia, vol. 17, no. 11, pp. 1949–1959,2015.
[2] R. P. Adams and R. S. Zemel, “Ranking via Sinkhorn propaga- tion,” arXiv preprint arXiv:1106.1925,2011.
[3] A. Agrawal, M. Dontcheva, M. Agrawala, S. Drucker, A. Col- burn, B. Curless, D. Salesin, and M. Cohen,“Interactive digital photomontage,” ACM Trans. Graph., vol. 23, no. 3,2004.
[4] Y. Bengio, Y. LeCunet al., “Scaling learning algorithms towards AI,” Large-scale kernel machines, vol. 34, no.5, pp. 1–41, 2007.
[5] G. Birkhoff, “Three observations on linear algebra,”Univ. Nac. Tucuma´n.RevistaA,vol.5,pp.147–151,1946.
[6] P. Bojanowski and A. Joulin, “Unsupervised learning bypredict- ing noise,” arXiv preprint arXiv:1704.05310,2017.
[7] S. Boyd and L. Vandenberghe, Convex Optimization. Cam- bridge University Press,2004.
[8] S. Branson, O. Beijbom, and S. Belongie, “Efficient large-scale structured learning,” in CVPR,2013.
[9] B. J. Brown, C. Toler-Franklin, D. Nehab, M. Burns, D.Dobkin, Vlachopoulos, C. Doumas, S. Rusinkiewicz, and T. Weyrich, “A system for high-volume acquisition and matching of fresco fragments: Reassembling theran wall paintings,” in ACM Trans- actions on Graphics (TOG), vol. 27, no. 3,2008.
[10] R. A. Brualdi, “Some applications of doubly stochastic matri- ces,” Linear Algebra and its Applications, vol. 107,pp.77–100,1988.