A Framework for Detection of Accuracy of Spam in Twitter
Pooja Naik1 , Monisha S2 , Supritha Shetty3 , Pooja NR4 , Anoop N Prasad5
Section:Research Paper, Product Type: Journal Paper
Volume-07 ,
Issue-15 , Page no. 105-110, May-2019
CrossRef-DOI: https://doi.org/10.26438/ijcse/v7si15.105110
Online published on May 16, 2019
Copyright © Pooja Naik, Monisha S, Supritha Shetty, Pooja NR, Anoop N Prasad . This is an open access article distributed under the Creative Commons Attribution License, which permits unrestricted use, distribution, and reproduction in any medium, provided the original work is properly cited.
View this paper at Google Scholar | DPI Digital Library
How to Cite this Paper
- IEEE Citation
- MLA Citation
- APA Citation
- BibTex Citation
- RIS Citation
IEEE Style Citation: Pooja Naik, Monisha S, Supritha Shetty, Pooja NR, Anoop N Prasad, “A Framework for Detection of Accuracy of Spam in Twitter,” International Journal of Computer Sciences and Engineering, Vol.07, Issue.15, pp.105-110, 2019.
MLA Style Citation: Pooja Naik, Monisha S, Supritha Shetty, Pooja NR, Anoop N Prasad "A Framework for Detection of Accuracy of Spam in Twitter." International Journal of Computer Sciences and Engineering 07.15 (2019): 105-110.
APA Style Citation: Pooja Naik, Monisha S, Supritha Shetty, Pooja NR, Anoop N Prasad, (2019). A Framework for Detection of Accuracy of Spam in Twitter. International Journal of Computer Sciences and Engineering, 07(15), 105-110.
BibTex Style Citation:
@article{Naik_2019,
author = {Pooja Naik, Monisha S, Supritha Shetty, Pooja NR, Anoop N Prasad},
title = {A Framework for Detection of Accuracy of Spam in Twitter},
journal = {International Journal of Computer Sciences and Engineering},
issue_date = {5 2019},
volume = {07},
Issue = {15},
month = {5},
year = {2019},
issn = {2347-2693},
pages = {105-110},
url = {https://www.ijcseonline.org/full_spl_paper_view.php?paper_id=1209},
doi = {https://doi.org/10.26438/ijcse/v7i15.105110}
publisher = {IJCSE, Indore, INDIA},
}
RIS Style Citation:
TY - JOUR
DO = {https://doi.org/10.26438/ijcse/v7i15.105110}
UR - https://www.ijcseonline.org/full_spl_paper_view.php?paper_id=1209
TI - A Framework for Detection of Accuracy of Spam in Twitter
T2 - International Journal of Computer Sciences and Engineering
AU - Pooja Naik, Monisha S, Supritha Shetty, Pooja NR, Anoop N Prasad
PY - 2019
DA - 2019/05/16
PB - IJCSE, Indore, INDIA
SP - 105-110
IS - 15
VL - 07
SN - 2347-2693
ER -
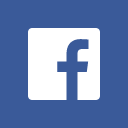
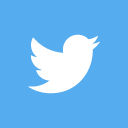
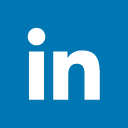
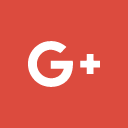
Abstract
With millions of users tweeting around the world, real time search systems and different types of mining tools are emerging to allow people tracking the repercussion of events and news on Twitter. Trending topics, the most talked about items on Twitter at a given point in time, have been seen as an opportunity to generate traffic and revenue. Spammers post tweets containing typical words of a trending topic and URLs, usually obfuscated by URL shortness, that lead users to completely unrelated websites. This kind of spam can contribute to de-value real time search services unless mechanisms to fight and stop spammers can be found. To solve this issue, we propose to take tweet text features along with user-based features. We have evaluated our approach with natural language processing and the naïve-Bayes machine learning algorithm.
Key-Words / Index Term
Twitter, tweets, spam, navie bayes, natural lanugage processing
References
[1] C. P.-Y. Chin, N. Evans, and K.-K. R. Choo, “Exploring factors influencingthe use of enterprise social networks in multinational professionalservice firms,” J. Organizat. Comput. Electron. Commerce, vol. 25, no. 3, pp. 289–315, 2017.
[2] H. T sukayama, “Twitter turns 7: Users send over 400 million tweets per day,” Washingon Post, Mar. 2017.
[3] T. Wu, S. Liu, J. Zhang, and Y. Xiang, “Twitter spam detection based on deep learning,” in Proceedings of the Australasian Computer Science Week Multiconference, ser. ACSW ’17. New York, NY,USA.
[4] C. Chen, J. Zhang, X. Chen, Y. Xiang, and W. Zhou, “6 million spam tweets: A large ground truth for timely twitter spam detection,” in 2016 IEEE International Conference on Communications (ICC), June 2015, pp. 7065–7070.
[5] F. Benevenuto, G. Magno, T. Rodrigues, and V. Almeida, “Detecting spammer on Twitter,” presented at the 7th Annu. Collab. Electron. Messaging Anti-Abuse Spam Conf., Redmond, WA, USA, Jul. 2017.
[6] L. Timson, “ Electoral commission Twitter account hacked, voters asked not to click,” Sydney Morning Herald, Aug. 2013 [Online]. Available: http://www.s mh.com.au/it-pro/security- it/electoral-commission-twitteraccount- hacked-voters-asked- not-to-click-20130807-hv1b 5.html
[7] Z. Miller, B. Dickinson, W. Deitrick, W. Hu, and A. H. Wang, “Twitter spammer detection using data stream clustering,” Inf. Sci., vol. 260, pp. 64– 73.
[8] K. Thomas, C. Grie r, D. Song, and V. Pa xson, Suspended accounts in retrospect: An analysis of Twitter spam,” in Proc. ACM SIGCOMM Conf. Internet Meas., pp. 243– 258.
[9] K. Thomas, C. Grie r, D. Song, and V. Pa xson, Suspended accounts in retrospect: An analysis of Twitter spam,” in Proc. ACM SIGCOMM Conf. Internet Meas.,pp. 243– 258.
[10] F. Benevenuto, G. Magno, T. Rodrigues, and V. Almeida, “Detecting spammers on twitter,” in In Collaboration, Electronic messaging, Anti- Abuse and Spam Conference.
[11] C. Pash., “The lure of Naked Hollywood Star Photos Sent the Internet into Meltdown in New Zealand, Bus. Insider, accessed on Aug. 1, 2015,” https://tinyurl.com/yc93ssj4,2017.
[12] “BotMaker,” blog.twitter,us/a/2014fighting-spam-with-botmaker.
[13] Witten and E. Frank. Data Mining: Practical machine learning tools and techniques. Morgan Kaufmann, 2016.
[14] C. Wu, K. Cheng, Q. Zhu, and Y. Wu. Using visual features for anti-spam filtering. In IEEE Int’l Conference on Image Processing (ICIP), 2017.
[15] Y. Xie, F. Yu, K. Achan, R. Panigrahy, G. Hulten, and I. Osipkov. Spamming botnets: Signatures and characteristics. In ACM SIGCOMM,.
[16] D. Fetterly, M. Manasse, and M. Najork. Spam, damn spam, and statistics: Using statistical analysis to locate spam web pages. In Int’l Workshop on the Web and Databases (WebDB),.
[17] S. Garriss, M. Kaminsky, M. Freedman, B. Karp,
[18] D. Mazi`eres, and H. Yu. Re: Reliable email. In USENIX Conference on Networked Systems Design & Implementation (NSDI), 2016.
[19] Z. Gy¨ongyi, H. Garcia-Molina, and J. Pedersen. Combating web spam with trustrank. In Int’l. Conference on Very Large Data Bases (VLDB), 2017.
[20] P. Heymann, G. Koutrika, and H. Garcia-Molina. Fighting spam on social web sites: A survey of approaches and future challenges. IEEE Internet Computing, 11, 2017.
[21] A Framework for Real-Time Spam Detection in Twitter
Himank Gupta, Mohd. Saalim Jamal, Sreekanth Madisetty and Maunendra Sankar Desarkar Department of Computer Science and Engineering,Indian Institute of Technology Hyderabad, India,2018.