Intelligent Product Retrieval System
Manisha Sharma1 , Pavani S2 , Pooja R3 , Varshitha U4 , Sunanda V K5
Section:Research Paper, Product Type: Journal Paper
Volume-07 ,
Issue-15 , Page no. 128-132, May-2019
CrossRef-DOI: https://doi.org/10.26438/ijcse/v7si15.128132
Online published on May 16, 2019
Copyright © Manisha Sharma, Pavani S, Pooja R, Varshitha U, Sunanda V K . This is an open access article distributed under the Creative Commons Attribution License, which permits unrestricted use, distribution, and reproduction in any medium, provided the original work is properly cited.
View this paper at Google Scholar | DPI Digital Library
How to Cite this Paper
- IEEE Citation
- MLA Citation
- APA Citation
- BibTex Citation
- RIS Citation
IEEE Style Citation: Manisha Sharma, Pavani S, Pooja R, Varshitha U, Sunanda V K, “Intelligent Product Retrieval System,” International Journal of Computer Sciences and Engineering, Vol.07, Issue.15, pp.128-132, 2019.
MLA Style Citation: Manisha Sharma, Pavani S, Pooja R, Varshitha U, Sunanda V K "Intelligent Product Retrieval System." International Journal of Computer Sciences and Engineering 07.15 (2019): 128-132.
APA Style Citation: Manisha Sharma, Pavani S, Pooja R, Varshitha U, Sunanda V K, (2019). Intelligent Product Retrieval System. International Journal of Computer Sciences and Engineering, 07(15), 128-132.
BibTex Style Citation:
@article{Sharma_2019,
author = {Manisha Sharma, Pavani S, Pooja R, Varshitha U, Sunanda V K},
title = {Intelligent Product Retrieval System},
journal = {International Journal of Computer Sciences and Engineering},
issue_date = {5 2019},
volume = {07},
Issue = {15},
month = {5},
year = {2019},
issn = {2347-2693},
pages = {128-132},
url = {https://www.ijcseonline.org/full_spl_paper_view.php?paper_id=1213},
doi = {https://doi.org/10.26438/ijcse/v7i15.128132}
publisher = {IJCSE, Indore, INDIA},
}
RIS Style Citation:
TY - JOUR
DO = {https://doi.org/10.26438/ijcse/v7i15.128132}
UR - https://www.ijcseonline.org/full_spl_paper_view.php?paper_id=1213
TI - Intelligent Product Retrieval System
T2 - International Journal of Computer Sciences and Engineering
AU - Manisha Sharma, Pavani S, Pooja R, Varshitha U, Sunanda V K
PY - 2019
DA - 2019/05/16
PB - IJCSE, Indore, INDIA
SP - 128-132
IS - 15
VL - 07
SN - 2347-2693
ER -
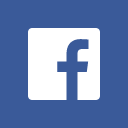
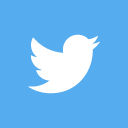
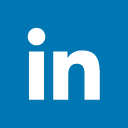
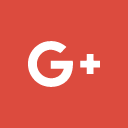
Abstract
It is desired (especially for young people) to shop for the same or similar products shown in the multimedia contents (such as online TV programs). This indicates an urgent demand for improving the experience of TV-to-Online (T2O). In this paper, a transfer learning approach as well as a prototype system for effortless T2O experience is developed. In this paper, a novel manifold regularized heterogeneous multitask metric learning framework is proposed, in which each domain is treated equally. The proposed approach allows us to simultaneously exploit the information from other domains and the unlabelled. In the system, a key component is high-precision product search, which is to fulfil exact matching between a query item and the database ones. The matching performance primarily relies on distance estimation, but the data characteristics cannot be well modelled and exploited by a simple Euclidean distance.
Key-Words / Index Term
TV-to-Online, distance metric learning, transfer learning, heterogeneous domains, manifold regularization, ranking-based loss.
References
[1] I. Gonzalez-Diaz, M. Birinci, F. Diaz-de Maria, and E. J. Delp, “Neighborhood matching for image retrieval,” IEEE Trans. Multimedia, vol. 19, no. 3, pp. 544–558, Mar. 2017.
[2] S. Karaoglu, R. Tao, T. Gevers, and A. W. Smeulders, “Words matter: Scene text for image classification and retrieval,” IEEE Trans.Multimedia, vol. 19, no. 5, pp. 1063–1076, May 2017.
[3] J.-E. Lee, R. Jin, and A. K. Jain, “Rank-based distance metric learning: An application to image retrieval,” in Proc. IEEE Conf. Comput. Vision Pattern Recognit., 2008, pp. 1–8. 2124 IEEE TRANSACTIONS ON MULTIMEDIA, VOL. 20, NO. 8, AUGUST 2018
[4] E. P. Xing, M. I. Jordan, S. Russell, and A. Ng, “Distance metric learning with application to clustering with side-information,” in Proc. Int. Conf. Neural Inf. Process. Syst., 2002, pp. 505–512.
[5] Y. Luo, Y. Wen, and D. Tao, “Heterogeneous multitask metric learning across multiple domains,” IEEE Trans. Neural Netw. Learn. Syst., Oct. 4, 2017, doi: 10.1109/TNNLS.2017.2750321.
[6] Y. Zhang and D.-Y. Yeung, “Multi-task learning in heterogeneous feature spaces,” in Proc. AAAI Conf. Artif. Intell., 2011, pp. 574–579.
[7] P. Xie and E. P. Xing, “Multi-modal distance metric learning,” in Proc.Int. Joint Conf. Artif. Intell., 2013, pp. 1806–1812.
[8] K. Aas and L. Eikvil, “Text categorization:Asurvey,”Norwegian Comput. Center, Oslo, Norway, Tech. Rep., 1999.
[9] R. Suzuki, D. Sakamoto, and T. Igarashi, “AnnoTone: Record-time audio watermarking for context-aware video editing,” in Proc. ACM Conf. Human Factors Comput. Syst., 2015, pp. 57–66.
[10] Y. Luo et al., “Multiview vector-valued manifold regularization for multilabel image classification,” IEEE Trans. Neural Netw. Learn. Syst., vol. 24, no. 5, pp. 709–722, May 2013.