Diagnose Anxiety and Depression in young children using Machine Learning
Rushmitha K1 , Sravani V2 , Jyothsana. R3 , Harsha. A.C4
Section:Research Paper, Product Type: Journal Paper
Volume-07 ,
Issue-15 , Page no. 165-170, May-2019
CrossRef-DOI: https://doi.org/10.26438/ijcse/v7si15.165170
Online published on May 16, 2019
Copyright © Rushmitha K, Sravani V, Jyothsana. R, Harsha. A.C . This is an open access article distributed under the Creative Commons Attribution License, which permits unrestricted use, distribution, and reproduction in any medium, provided the original work is properly cited.
View this paper at Google Scholar | DPI Digital Library
How to Cite this Paper
- IEEE Citation
- MLA Citation
- APA Citation
- BibTex Citation
- RIS Citation
IEEE Style Citation: Rushmitha K, Sravani V, Jyothsana. R, Harsha. A.C, “Diagnose Anxiety and Depression in young children using Machine Learning,” International Journal of Computer Sciences and Engineering, Vol.07, Issue.15, pp.165-170, 2019.
MLA Style Citation: Rushmitha K, Sravani V, Jyothsana. R, Harsha. A.C "Diagnose Anxiety and Depression in young children using Machine Learning." International Journal of Computer Sciences and Engineering 07.15 (2019): 165-170.
APA Style Citation: Rushmitha K, Sravani V, Jyothsana. R, Harsha. A.C, (2019). Diagnose Anxiety and Depression in young children using Machine Learning. International Journal of Computer Sciences and Engineering, 07(15), 165-170.
BibTex Style Citation:
@article{K_2019,
author = {Rushmitha K, Sravani V, Jyothsana. R, Harsha. A.C},
title = {Diagnose Anxiety and Depression in young children using Machine Learning},
journal = {International Journal of Computer Sciences and Engineering},
issue_date = {5 2019},
volume = {07},
Issue = {15},
month = {5},
year = {2019},
issn = {2347-2693},
pages = {165-170},
url = {https://www.ijcseonline.org/full_spl_paper_view.php?paper_id=1220},
doi = {https://doi.org/10.26438/ijcse/v7i15.165170}
publisher = {IJCSE, Indore, INDIA},
}
RIS Style Citation:
TY - JOUR
DO = {https://doi.org/10.26438/ijcse/v7i15.165170}
UR - https://www.ijcseonline.org/full_spl_paper_view.php?paper_id=1220
TI - Diagnose Anxiety and Depression in young children using Machine Learning
T2 - International Journal of Computer Sciences and Engineering
AU - Rushmitha K, Sravani V, Jyothsana. R, Harsha. A.C
PY - 2019
DA - 2019/05/16
PB - IJCSE, Indore, INDIA
SP - 165-170
IS - 15
VL - 07
SN - 2347-2693
ER -
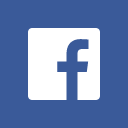
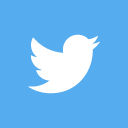
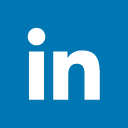
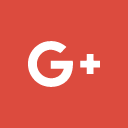
Abstract
Suicide is the second leading cause of death among young adults but the challenges of preventing suicide are significant because the signs often seem invisible. Research has shown that clinicians are not able to reliably predict when someone is at greatest risk.. Machine learning and data extracted from one 20-second phase of the task are used to predict diagnosis in a large sample of children with and without an internalizing diagnosis. Nevertheless, the proposed approach provides a rapid, objective, and accurate means for diagnosing internalizing disorders in young children. This new approach reduces the time required for diagnosis while also limiting the need for highly trained personnel – each of which can help to reduce the length of waitlists for child mental health services. While these results can likely be improved and extended, this is an important first step in reducing the barriers associated with assessing young children for internalizing disorders.
Key-Words / Index Term
ML, KNN, LAN
References
[1] H. L. Egger and A. Angold, “Common emotional and behavioural disorders in preschool children: presentation, nosology, and epidemiology,” J Child Psychol Psychiatry, vol. 47, no. 3–4, pp. 313– 337, Apr. 2006.
[2] S. J. Bufferd, L. R. Dougherty, G. A. Carlson, and D. N. Klein, “Parent-Reported Mental Health in Preschoolers: Findings Using a Diagnostic Interview,” Compr Psychiatry, vol. 52, no. 4, pp. 359–369, 2011.
[3] M. Tandon, E. Cardeli, and J. Luby, “Internalizing Disorders in Early Childhood: A Review of Depressive and Anxiety Disorders,” Childand Adolescent Psychiatric Clinics of North America, vol. 18, no. 3, pp. 593–610, Jul. 2009.
[4] J. L. Luby, A. C. Belden, J. Pautsch, X. Si, and E. Spitznagel, “The clinical significance of preschool depression: Impairment in functioning and clinical markers of the disorder,” Journal of AffectiveDisorders, vol. 112, no. 1–3, pp. 111–119, Jan. 2009.
[5] D. J. Whalen, C. M. Sylvester, and J. L. Luby, “Depression and
Anxiety in Preschoolers: A Review of the Past 7 Years,” Child and Adolescent Psychiatric Clinics of North America, vol. 26, no. 3, pp.503–522, Jul. 2017.
[6] H.-U. Wittchen and H. Sonntag, “Nicotine consumption in mental disorders: a clinical epidemiological perspective,” EuropeanNeuropsychopharmacology, vol. 10, p. 119, Sep. 2000.
[7] A. Bittner, H. L. Egger, A. Erkanli, E. Jane Costello, D. L. Foley, and A. Angold, “What do childhood anxiety disorders predict?,” J ChildPsychol Psychiatry, vol. 48, no. 12, pp. 1174–1183, Dec. 2007.
[8] M. S. GOULD et al., “Psychopathology Associated With SuicidalIdeation and Attempts Among Children and Adolescents,” Journal ofthe American Academy of Child & Adolescent Psychiatry, vol. 37, no. 9, pp. 915–923, Sep. 1998.
[9] M. G. Craske and M. B. Stein, “Anxiety,” The Lancet, vol. 388, no. 10063, pp. 3048 3059, Dec. 2016.
[10] A. Konnopka, F. Leichsenring, E. Leibing, and H.-H. König, “Costof- illness studies and cost-effectiveness analyses in anxiety disorders:
A systematic review,” Journal of Affective Disorders, vol. 114, no. 1,
pp. 14–31, Apr. 2009.
[11] J. L. Luby, “Preschool Depression: The Importance of Identification of Depression Early in Development,” Current Directions inPsychological Science, vol. 19, no. 2, pp. 91–95, May 2010.
[12] J. L. Luby et al., “The clinical picture of depression in preschool children,” Journal of the American Academy of Child & AdolescentPsychiatry, vol. 42, no. 3, pp. 340–348, Mar. 2003.
[13] J. Garber and K. M. Kaminski, “Laboratory and performance-based measures of depression in children and adolescents,” Journal ofclinical child psychology, vol. 29, no. 4, pp. 509–525, 2000.
[14] T. E. Chansky and P. C. Kendall, “Social expectancies and selfperceptions
in anxiety-disordered children,” J Anxiety Disord, vol. 11, no. 4, pp. 347–363, Aug. 1997.
[15] D. J. Kolko and A. E. Kazdin, “Emotional/behavioral problems in clinic and nonclinic children: Correspondence among child, parent and teacher reports,” Journal of Child Psychology and Psychiatry, vol. 34, no. 6, pp. 991–1006, 1993.
[16] A. G. Renouf and M. Kovacs, “Concordance between mothers’ reports and children’s self-reports of depressive symptoms: Alongitudinal study,” Journal of the American Academy of Child &Adolescent Psychiatry, vol. 33, no. 2, pp. 208–216, Feb. 1994.
[17] D. Cawthorpe et al., “Priority-Setting for Children’s Mental Health: Clinical Usefulness and Validity of the Priority Criteria Score,” J CanAcad Child Adolesc Psychiatry, vol. 16, no. 1, pp. 18–26, Feb. 2007.
[18] C. Lord et al., “The autism diagnostic observation schedule-generic: a standard measure of social and communication deficits associated with the spectrum of autism,” J Autism Dev Disord, vol. 30, no. 3, pp. 205–223, Jun. 2000.
[19] E. J. Mash and S. L. Foster, “Exporting analogue behavioural observation from research to clinical practice: useful or costdefective?,” Psychol Assess, vol. 13, no. 1, pp. 86–98, Mar. 2001.
[20] E. McGinnis et al., “Movements indicate threat response phases in children at-risk for anxiety,” IEEE Journal of Biomedical and HealthInformatics, vol. 21, no. 5, pp. 1460–1465, 2017.
[21] N. L. Lopez-Duran, N. J. Hajal, S. L. Olson, B. T. Felt, and D. M. Vazquez, “Individual differences in cortisol responses to fear and frustration during middle childhood,” J Exp Child Psychol, vol. 103, no. 3, pp. 285–295, Jul. 2009.
[22] J. J. Gross, “Emotion regulation: Affective, cognitive, and social consequences,” Psychophysiology, vol. 39, no. 3, pp. 281–291, May 2002.
[23] R. S. McGinnis, S. M. Cain, S. P. Davidson, R. V. Vitali, S. G. McLean, and N. C. Perkins, “Validation of Complementary Filter Based IMU Data Fusion for Tracking Torso Angle and Rifle Orientation,” presented at the ASME 2014 International Mechanical
Engineering Congress and Exposition, Montreal, QC, 2014, p.V003T03A052.
[24] R. McGinnis, “GitHub repository,” GitHub. [Online]. Available: https://github.com/ryan-mcginnis. [Accessed: 03-Nov-2017].
[25] B. Birmaher et al., “Schedule for Affective Disorders and Schizophrenia for School-Age Children (K-SADS-PL) for the Assessment of Preschool Children- A Preliminary Psychometric Study,” J Psychiatr Res, vol. 43, no. 7, pp. 680–686, Apr. 2009.