Improving Prediction Model Accuracy by Wavelet Transform Based on MRI Images of Brain Tumour Patients
Saniya Suhail1 , Savita S Dodakenchannavar2 , Shilpashree GL3 , Swetha M4 , Hemanth YK5
Section:Research Paper, Product Type: Journal Paper
Volume-07 ,
Issue-15 , Page no. 203-207, May-2019
CrossRef-DOI: https://doi.org/10.26438/ijcse/v7si15.203207
Online published on May 16, 2019
Copyright © Saniya Suhail, Savita S Dodakenchannavar, Shilpashree GL, Swetha M, Hemanth YK . This is an open access article distributed under the Creative Commons Attribution License, which permits unrestricted use, distribution, and reproduction in any medium, provided the original work is properly cited.
View this paper at Google Scholar | DPI Digital Library
How to Cite this Paper
- IEEE Citation
- MLA Citation
- APA Citation
- BibTex Citation
- RIS Citation
IEEE Style Citation: Saniya Suhail, Savita S Dodakenchannavar, Shilpashree GL, Swetha M, Hemanth YK, “Improving Prediction Model Accuracy by Wavelet Transform Based on MRI Images of Brain Tumour Patients,” International Journal of Computer Sciences and Engineering, Vol.07, Issue.15, pp.203-207, 2019.
MLA Style Citation: Saniya Suhail, Savita S Dodakenchannavar, Shilpashree GL, Swetha M, Hemanth YK "Improving Prediction Model Accuracy by Wavelet Transform Based on MRI Images of Brain Tumour Patients." International Journal of Computer Sciences and Engineering 07.15 (2019): 203-207.
APA Style Citation: Saniya Suhail, Savita S Dodakenchannavar, Shilpashree GL, Swetha M, Hemanth YK, (2019). Improving Prediction Model Accuracy by Wavelet Transform Based on MRI Images of Brain Tumour Patients. International Journal of Computer Sciences and Engineering, 07(15), 203-207.
BibTex Style Citation:
@article{Suhail_2019,
author = {Saniya Suhail, Savita S Dodakenchannavar, Shilpashree GL, Swetha M, Hemanth YK},
title = {Improving Prediction Model Accuracy by Wavelet Transform Based on MRI Images of Brain Tumour Patients},
journal = {International Journal of Computer Sciences and Engineering},
issue_date = {5 2019},
volume = {07},
Issue = {15},
month = {5},
year = {2019},
issn = {2347-2693},
pages = {203-207},
url = {https://www.ijcseonline.org/full_spl_paper_view.php?paper_id=1227},
doi = {https://doi.org/10.26438/ijcse/v7i15.203207}
publisher = {IJCSE, Indore, INDIA},
}
RIS Style Citation:
TY - JOUR
DO = {https://doi.org/10.26438/ijcse/v7i15.203207}
UR - https://www.ijcseonline.org/full_spl_paper_view.php?paper_id=1227
TI - Improving Prediction Model Accuracy by Wavelet Transform Based on MRI Images of Brain Tumour Patients
T2 - International Journal of Computer Sciences and Engineering
AU - Saniya Suhail, Savita S Dodakenchannavar, Shilpashree GL, Swetha M, Hemanth YK
PY - 2019
DA - 2019/05/16
PB - IJCSE, Indore, INDIA
SP - 203-207
IS - 15
VL - 07
SN - 2347-2693
ER -
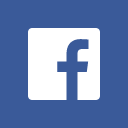
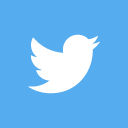
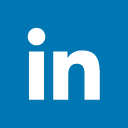
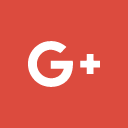
Abstract
Medical imaging provides proper diagnosis of brain tumour. Various techniques are implemented to detect the brain tumour from MRI images. One among them is the Denoising wavelet transform (DWT) method which is used to improve the accuracy of a prediction model by making use of MRI images in order to predict the overall survival time of brain tumour patients. Wavelet transform method detects the location and size of the tumour. The proposed methodology consists of image acquisition, Calculation of tissue density maps, statistical analysis. MRI provides generous information about the human soft tissue, which helps in the recognition of brain tumour. Image Segmentation categorises pixels into sections and hence defines the object regions. This paper proposes the image and feature fusion techniques to improve the accuracy of the prediction model.
Key-Words / Index Term
Machine learning, Denoising wavelet transform, MRI images, Histogram, Glioma brain tumour, Linear Regression
References
[1]. Iraky Khalifa, Aliaa Youssif and Howida Youssry,” MRI Brain Image Segmentation based on Wavelet and FCM Algorithm”.International Journal of Computer Applications 47(16):32-39, June 2012. Published by Foundation of Computer Science, New York, USA.
[2]. Y.Zhang and L.Wu “ MRI brain images classifier via Principal Component Analysis and Kernel Support Vectormachine”. Progress In Electromagnetics Research,Vol.130,369-388,2012.
[3]. Ms. Yogita K.Dubey and milind M.Mushrif,” Extraction of wavelet based features for classification of T2-weighted mri brain images”, signal & image processing : an international journal (SIPIJ) vol.3, no.1, february 2012.
[4]. G.VeeraSenthilKumar,Dr.S.Vasuki,&Dr.L.Ganesan “Segmentation of color images using 2D histogram clustering on SWT features”,2010.
[5]. K. Sikka, N. Sinha, K. Singh, and K. Mishra, "A fully automated algorithm under modified FCM framework for improved brain MR image segmentation " Magnetic Resonance Imaging, vol. 27, pp. 994-1004, 2009.
[6]. J. Rogowska, "Overview and Fundamentals of Medical Image Segmentation," Handbook of Medical Imaging: Processing and Analysis Management, I. Bankman, Ed, pp. 69-85, 2009.
[7]. K. Chuang, H. Tzeng, S. Chen, J. Wu, and J. Chen, " Fuzzy c-means clustering with spatial information for image segmentation," Computerized Medical Imaging and Graphics vol. 30, pp. 9-15, 2006.
[8]. D. Feng, "Segmentation of Soft Tissues in Medical Images," P. h. D thesis National University of Singapore, 2005.
[9]. A.F. Goldszal and D. L. Pham, "Volumetric Segmentation of Magnetic Resonance Images of the Brain," in Handbook of Medical Image processing, I. Bankman, Ed. , ed: Academic Press, 2000, pp. 185- 194.
[10]. D. Pham, C. Xu, and L. Prince, "A Survey of Current Methods in Medical Image Segmentation," Annual Review of Biomedical Engineering, vol. 2, pp. 315- 338, 1998.
[11]. Bamberg ,R.H. and Smith,M.J.T. “A filter bank for the directional decomposition of images”,IEEE Transactions on signal processing,vol.40,pp.882- 893,April 1992.
[12]. Robert l. Cannon, jitendra v. Dave, and James c. Bezdek “Efficient Implementation of the Fuzzy c-Means Clustering Algorithms” IEEE trransactions on pattern analysis and machine intelligence. Vol. Pami8, no. 2, march 1986.
[13]. E. C. Holland, “Progenitor cells and glioma formation,” J. Current. Opinion in Neurology, vol. 14, no. 6, pp. 683–688, 2001.
[14]. H. Ohgaki and P. Kleihues, “Population-based studies on incidence, survival rates, and genetic alterations inastrocytic and oligodendroglia gliomas,” J. of Neuropathology & Experimental Neurology, vol. 64, no. 6, pp. 479–489, June 2005.
[15]. Alex Lobera,” Imaging in Glioblastoma Multiforme”, Updated: Feb 10, 2017, online access 1/10/2018 https://emedicine.medscape.com/article/340870-overview#a
[16]. BH Menze et al., "The Multimodal Brain Tumor Image Segmentation Benchmark (BRATS)", J. IEEE Transactions on Medical Imaging, vol. 34, no. 10, 2015, pp. 1993-2024, doi: 10.1109/TMI.2014.2377694.
[17]. S. Bakas et al., "Advancing the Cancer Genome Atlas glioma MRI collections with expert segmentation labels and radiomic features", J. Nature Scientific Data, Sept. 5 2017, doi:10.1038/sdata.2017.117.
[18]. L. Chato and S. Latifi, “Machine Learning and Deep Learning Techniques to Predict Overall Survival of Brain Tumor Patients using MRI Images”, on Proc. 17th IEEE Int. Conf. Bio-Informatics and Bio-Engineering BIBE 2017, Washington DC, pp. 9-14, 23rd-25th Oct. 2017, 2471- 7819/17/31.00 ©2017 IEEE, doi: 10.1109/BIBE.2017.00009.
[19]. D. Drumheller, “General expressions for Rician density and distribution functions,” IEEE Trans. Aerosp. Electron. Syst., vol. 29, no. 2, pp. 580– 588, Apr. 1993.
[20]. H. Gudbjartsson and S. Patz, “The Rician distribution of noisy MRI data,” Magn. Reson. Med., vol. 34, pp. 910–914, Aug. 1995. [9] Raghuveer M. Rao., AS. Bopardikar Wavelet Transforms: Introduction To Theory And Application Published By AddisonWesly 2001 pp. l-12.