Detection of Unusual Activites at ATM Using Machine Learning
Tejas D1 , Varshini K2 , Sushmitha U3 , Sunandha V.K4
Section:Research Paper, Product Type: Journal Paper
Volume-07 ,
Issue-15 , Page no. 213-216, May-2019
CrossRef-DOI: https://doi.org/10.26438/ijcse/v7si15.213216
Online published on May 16, 2019
Copyright © Tejas D, Varshini K, Sushmitha U, Sunandha V.K . This is an open access article distributed under the Creative Commons Attribution License, which permits unrestricted use, distribution, and reproduction in any medium, provided the original work is properly cited.
View this paper at Google Scholar | DPI Digital Library
How to Cite this Paper
- IEEE Citation
- MLA Citation
- APA Citation
- BibTex Citation
- RIS Citation
IEEE Style Citation: Tejas D, Varshini K, Sushmitha U, Sunandha V.K, “Detection of Unusual Activites at ATM Using Machine Learning,” International Journal of Computer Sciences and Engineering, Vol.07, Issue.15, pp.213-216, 2019.
MLA Style Citation: Tejas D, Varshini K, Sushmitha U, Sunandha V.K "Detection of Unusual Activites at ATM Using Machine Learning." International Journal of Computer Sciences and Engineering 07.15 (2019): 213-216.
APA Style Citation: Tejas D, Varshini K, Sushmitha U, Sunandha V.K, (2019). Detection of Unusual Activites at ATM Using Machine Learning. International Journal of Computer Sciences and Engineering, 07(15), 213-216.
BibTex Style Citation:
@article{D_2019,
author = {Tejas D, Varshini K, Sushmitha U, Sunandha V.K},
title = {Detection of Unusual Activites at ATM Using Machine Learning},
journal = {International Journal of Computer Sciences and Engineering},
issue_date = {5 2019},
volume = {07},
Issue = {15},
month = {5},
year = {2019},
issn = {2347-2693},
pages = {213-216},
url = {https://www.ijcseonline.org/full_spl_paper_view.php?paper_id=1229},
doi = {https://doi.org/10.26438/ijcse/v7i15.213216}
publisher = {IJCSE, Indore, INDIA},
}
RIS Style Citation:
TY - JOUR
DO = {https://doi.org/10.26438/ijcse/v7i15.213216}
UR - https://www.ijcseonline.org/full_spl_paper_view.php?paper_id=1229
TI - Detection of Unusual Activites at ATM Using Machine Learning
T2 - International Journal of Computer Sciences and Engineering
AU - Tejas D, Varshini K, Sushmitha U, Sunandha V.K
PY - 2019
DA - 2019/05/16
PB - IJCSE, Indore, INDIA
SP - 213-216
IS - 15
VL - 07
SN - 2347-2693
ER -
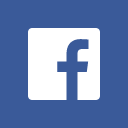
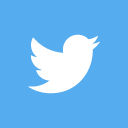
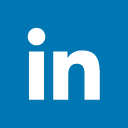
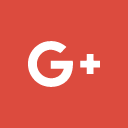
Abstract
The idea of designing and implementation of security against ATM theft is born with the observation of our real life incidents happening around us. This project deals with prevention of ATM crimes and hence overcome the drawback found in existing technology in our society. This paper uses machine learning to enhance the security in ATM. When any suspicious activities such as a man holding a gun in his hand is detected using ORB algorithm, a person attempting to close the camera at ATM, more than two persons entering into ATM, fighting scenes happening at ATM will be detected as an unusual activity and alarm is raised at ATM and a message is passed to nearest police station. Parallelly an email consisting a snap of unusual activity will be sent to the registered police official e-mail id, this helps the police officers to analyze the situation and overcome the fake alarms.
Key-Words / Index Term
Unusual Activity, Machine Learning, ATM security
References
[1] Sorina Smeureanu, Radu Tudor Ionescu, “Deep Appearance Features for Abnormal Behavior Detection in Video”, Springer ICIAP, 2017
[2] A.V. Kulakarni, J.S. Jagtap, V.K. Harpale, “Object recognition with ORB and its Implementation on FPGA”, IJCSE,2013
[3] Letian Li,Lin Wu,Yongcun Gao. “Improved image matching method based on ORB”. 2016 17th IEEE/ACIS International Conference on Software Engineering, Artificial Intelligence, Networking and Parallel/Distributed Computing (SNPD).
[4] Rublee E, Rabaud V, Konolige K, et al, “ORB: an efficient alternative to SIFT or SURF,” Computer Vision (ICCV), 2011 IEEE International Conference on. IEEE,pp.2564-2571,2011.
[5] Mohan, A. S., & Resmi, R. “Video image processing for moving object detection and segmentation using background subtraction.”2014 First International Conference on Computational Systems and Communications (ICCSC).
[6] Jakubovic, A., & Velagic, J. “Image Feature Matching and Object Detection Using Brute-Force Matchers.” 2018 International Symposium ELMAR.
[7] GuoQing Yin, & Bruckner, D. (2009). “Gaussian models and fast learning algorithm for persistence analysis of tracked video objects”. 2009 2nd Conference on Human System Interactions.
[8] Saini, D. K., Ahir, D., & Ganatra, A. “Techniques and Challenges in Building Intelligent Systems: Anomaly Detection in Camera Surveillance. Smart Innovation, Systems and Technologies”,Springer ICICT,2016.