Using Adaboost AND Majority Voting Techniques For Detecting Fradulent Transcations In Credit Card
S Sharath1 , Sherwin Sampath Doddamani2 , Lakshmikantha S3
Section:Research Paper, Product Type: Journal Paper
Volume-07 ,
Issue-15 , Page no. 217-219, May-2019
CrossRef-DOI: https://doi.org/10.26438/ijcse/v7si15.217219
Online published on May 16, 2019
Copyright © S Sharath, Sherwin Sampath Doddamani, Lakshmikantha S . This is an open access article distributed under the Creative Commons Attribution License, which permits unrestricted use, distribution, and reproduction in any medium, provided the original work is properly cited.
View this paper at Google Scholar | DPI Digital Library
How to Cite this Paper
- IEEE Citation
- MLA Citation
- APA Citation
- BibTex Citation
- RIS Citation
IEEE Style Citation: S Sharath, Sherwin Sampath Doddamani, Lakshmikantha S, “Using Adaboost AND Majority Voting Techniques For Detecting Fradulent Transcations In Credit Card,” International Journal of Computer Sciences and Engineering, Vol.07, Issue.15, pp.217-219, 2019.
MLA Style Citation: S Sharath, Sherwin Sampath Doddamani, Lakshmikantha S "Using Adaboost AND Majority Voting Techniques For Detecting Fradulent Transcations In Credit Card." International Journal of Computer Sciences and Engineering 07.15 (2019): 217-219.
APA Style Citation: S Sharath, Sherwin Sampath Doddamani, Lakshmikantha S, (2019). Using Adaboost AND Majority Voting Techniques For Detecting Fradulent Transcations In Credit Card. International Journal of Computer Sciences and Engineering, 07(15), 217-219.
BibTex Style Citation:
@article{Sharath_2019,
author = {S Sharath, Sherwin Sampath Doddamani, Lakshmikantha S},
title = {Using Adaboost AND Majority Voting Techniques For Detecting Fradulent Transcations In Credit Card},
journal = {International Journal of Computer Sciences and Engineering},
issue_date = {5 2019},
volume = {07},
Issue = {15},
month = {5},
year = {2019},
issn = {2347-2693},
pages = {217-219},
url = {https://www.ijcseonline.org/full_spl_paper_view.php?paper_id=1230},
doi = {https://doi.org/10.26438/ijcse/v7i15.217219}
publisher = {IJCSE, Indore, INDIA},
}
RIS Style Citation:
TY - JOUR
DO = {https://doi.org/10.26438/ijcse/v7i15.217219}
UR - https://www.ijcseonline.org/full_spl_paper_view.php?paper_id=1230
TI - Using Adaboost AND Majority Voting Techniques For Detecting Fradulent Transcations In Credit Card
T2 - International Journal of Computer Sciences and Engineering
AU - S Sharath, Sherwin Sampath Doddamani, Lakshmikantha S
PY - 2019
DA - 2019/05/16
PB - IJCSE, Indore, INDIA
SP - 217-219
IS - 15
VL - 07
SN - 2347-2693
ER -
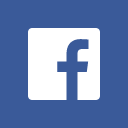
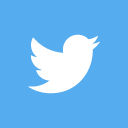
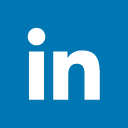
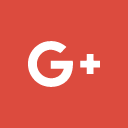
Abstract
Credit card fraud is a serious problem in financial services. Billions of dollars are lost due to credit card fraud every year. There is a lack of research studies on analyzing real-world credit card data owing to con dentiality issues. Nowadays digitalization gaining popularity because of seamless, easy and convenience use of e-commerce. It became very rampant and easy mode of payment. The experimental results positively indicate that the majority voting method achieves good accuracy rates in detecting fraud cases in credit cards. Inspired by the recent novel idea of Trerngad [1], we also quantize the released gradients to ternary levels {−B, 0, B}, where B is the bound of gradient clipping. Voting based prediction aggregation provides the final predictions. A hybrid technique of under-sampling and oversampling is carried out on the skewed data. Capsule Network (CapsNet) is adopted to further dig some deep features on the base of the expanded features, and then a fraud detection model is trained to identify if a transaction is legal or fraud.
Key-Words / Index Term
AdaBoost, classification, credit card, fraud detection, predictive modelling, voting
References
[1] N. Mahmoodi, E. Duman, “Detecting credit card fraud by Modified Fisher Discriminant Analysis”, Elsevier Expert System with Application, 2015, pp. 2510-2516.
[2] Y. Sachin, E. Duman, “Detecting Credit Card Fraud by Decision Tree and Support Vector Machine”, In Proceedings of the international multi Conference of Engineers and Computer Scientists, Hong Kong, 2011, pp. 1-6.
[3] V. Van Vlasselaer et al., “APATE : A novel approach for automated credit card transaction fraud detection using network-based extensions[J],”Decision Support Systems, 2015, 75:38-48.
[4] Mafarja M , Mirjalili S. Hybrid Whale Optimization Algorithm with simulated annealing for feature selection[J]. Neurocomputing, 2017, 260:302-312.
[5] Kecman, Vojislav; Learning and Soft Computing Support Vector Machines, Neural Networks, Fuzzy Logic Systems, The MIT Press, Cambridge, MA, 2001.
[6] Fadaei Noghani, F., and M. Moattar. ”Ensemble Classification and Extended Feature Selection for Credit Card Fraud Detection.” Journal of AI and Data Mining 5.2 (2017): 235-243.
[7] The Nilson Report. (2015). U.S. Credit & Debit Cards 2015. David Robertson.
[8] Stolfo, S., Fan, D. W., Lee, W., Prodromidis, A., & Chan, P. (1997). Credit card fraud detection using meta-learning: Issues and initial results. In AAAI-97 Workshop on Fraud Detection and Risk Management.