Detecting Density Changes of Mangrove Forest in India using Remotely Sensed Images
S. Chakraborty1 , S. Nandi2 , S. Ahmed3 , N. Adhikari4 , M. Sultana5 , S. Bhattacharya6
Section:Research Paper, Product Type: Journal Paper
Volume-11 ,
Issue-01 , Page no. 249-256, Nov-2023
Online published on Nov 30, 2023
Copyright © S. Chakraborty, S. Nandi, S. Ahmed, N. Adhikari, M. Sultana, S. Bhattacharya . This is an open access article distributed under the Creative Commons Attribution License, which permits unrestricted use, distribution, and reproduction in any medium, provided the original work is properly cited.
View this paper at Google Scholar | DPI Digital Library
How to Cite this Paper
- IEEE Citation
- MLA Citation
- APA Citation
- BibTex Citation
- RIS Citation
IEEE Citation
IEEE Style Citation: S. Chakraborty, S. Nandi, S. Ahmed, N. Adhikari, M. Sultana, S. Bhattacharya, “Detecting Density Changes of Mangrove Forest in India using Remotely Sensed Images,” International Journal of Computer Sciences and Engineering, Vol.11, Issue.01, pp.249-256, 2023.
MLA Citation
MLA Style Citation: S. Chakraborty, S. Nandi, S. Ahmed, N. Adhikari, M. Sultana, S. Bhattacharya "Detecting Density Changes of Mangrove Forest in India using Remotely Sensed Images." International Journal of Computer Sciences and Engineering 11.01 (2023): 249-256.
APA Citation
APA Style Citation: S. Chakraborty, S. Nandi, S. Ahmed, N. Adhikari, M. Sultana, S. Bhattacharya, (2023). Detecting Density Changes of Mangrove Forest in India using Remotely Sensed Images. International Journal of Computer Sciences and Engineering, 11(01), 249-256.
BibTex Citation
BibTex Style Citation:
@article{Chakraborty_2023,
author = {S. Chakraborty, S. Nandi, S. Ahmed, N. Adhikari, M. Sultana, S. Bhattacharya},
title = {Detecting Density Changes of Mangrove Forest in India using Remotely Sensed Images},
journal = {International Journal of Computer Sciences and Engineering},
issue_date = {11 2023},
volume = {11},
Issue = {01},
month = {11},
year = {2023},
issn = {2347-2693},
pages = {249-256},
url = {https://www.ijcseonline.org/full_spl_paper_view.php?paper_id=1441},
publisher = {IJCSE, Indore, INDIA},
}
RIS Citation
RIS Style Citation:
TY - JOUR
UR - https://www.ijcseonline.org/full_spl_paper_view.php?paper_id=1441
TI - Detecting Density Changes of Mangrove Forest in India using Remotely Sensed Images
T2 - International Journal of Computer Sciences and Engineering
AU - S. Chakraborty, S. Nandi, S. Ahmed, N. Adhikari, M. Sultana, S. Bhattacharya
PY - 2023
DA - 2023/11/30
PB - IJCSE, Indore, INDIA
SP - 249-256
IS - 01
VL - 11
SN - 2347-2693
ER -
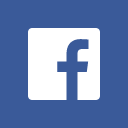
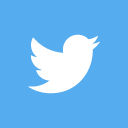
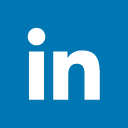
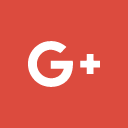
Abstract
The use of deep learning technology in the domain of biodiversity has been expanding over the past few years, with applications in wildlife and vegetation monitoring. The Convolutional (CNN) is a powerful tool that has enabled new feature extraction methods in computer vision. Remote sensing techniques, such as satellite and drone-assisted images, have also contributed to the development of vegetation cover assessment. This study focuses on detecting changes in vegetation cover in the Sundarbans mangrove forest, which is the world`s largest mangrove and a heritage site that supports over 4.37 million people and reduces 45 million tons of CO2. The study Neural Network used a deep learning model to analyze time-series data and achieved an accuracy score of 99.85% and a value of 1 for the other three metrics - precision, recall, and F1-Score. The study also includes a review of previous work in the field and proposes a novel model for vegetation cover assessment. This study emphasizes the importance of sustaining the ecology of the Sundarbans and provides valuable insights for future research in this field.
Key-Words / Index Term
Mangrove Forest; Deep Learning; Convolutional Neural Network; Remote Sensing Images.
References
[1] October 2021. [Online]. Available: http://www.wildbengal.com/sundarban.php.
[2] R. S. DeFries, R. A. Houghton, M. C. Hansen, C. B. Field, D. Skole and J. Townshend, “Carbon emissions from tropical deforestation and regrowth based on satellite observations for the 1980s and 1990s,” in Proceedings of the National Academy of Sciences, 2002.
[3] M. Emch and M. Peterson, “Mangrove forest cover change in the Bangladesh Sundarbans from 1989-2000: A remote sensing approach.,” Geocarto International, vol. 21, no. 1, pp. 5-12, 2006.
[4] M. I. Brown, T. Pearce, J. Leon, R. Sidle and R. Wilson, “Using remote sensing and traditional ecological knowledge (TEK) to understand mangrove change on the Maroochy River, Queensland, Australia.,” Applied Geography, vol. 94, pp. 71-83, 2018.
[5] P. Kumar, M. Rani, P. C. Pandey, A. Majumdar and M. S. Nathawat, “Monitoring of deforestation and forest degradation using remote sensing and GIS: A case study of Ranchi in Jharkhand (India),” Report and opinion, vol. 2, no. 4, pp. 14-20, 2010.
[6] S. N. M. Hoque, M. M. M. Ahmed and M. I. 5. 9.-1. Bhuiyan, “Investigating rapid deforestation and carbon dioxide release in Bangladesh using geospatial information from remote sensing data.,” Ecocycles, vol. 5, no. 2, pp. 97-105, 2019.
[7] P. P. De Bem, O. A. de Carvalho Junior, R. Fontes Guimarães and R. A. Trancoso Gomes, “Change detection of deforestation in the Brazilian Amazon using landsat data and convolutional neural networks,” Remote Sensing, vol. 12, no. 6, p. 901, 2020.
[8] D. Chamberlain, S. Phinn and H. Possingham, “Remote sensing of mangroves and estuarine communities in central Queensland, Australia.,” Remote Sensing, vol. 12, no. 1, p. 197, 2020.
[9] J. V. d. Oliveira, “Differences in precipitation and evapotranspiration between forested and deforested areas in the Amazon rainforest using remote sensing data.,” Environmental earth sciences, vol. 77, no. 6, pp. 1-14, 2018.
[10] M. Mortoja and T. Yigitcanlar, “Local drivers of anthropogenic climate change: Quantifying the impact through a remote sensing approach in Brisbane.,” Remote Sensing, vol. 12, no. 14, p. 2271, 2020.
[11] H. He, M. Chen, T. Chen and D. Li, “Matching of remote sensing images with complex background variations via Siamese convolutional neural network.,” Remote Sensing, vol. 10, no. 2, p. 355, 2018.
[12] J. S. Babu and T. Sudha, “Analysis and Detection of Deforestation Using Novel Remote-Sensing Technologies with Satellite Images,” in 2018 IDAS International Conference on Computing, Communications & Data Engineering (CCODE), 2018.
[13] M. D. Behera, P. Tripathi, P. Das, S. K. Srivastava, P. S. Roy, C. Joshi and Y. V. N. Krishnamurthy, “Remote sensing based deforestation analysis in Mahanadi and Brahmaputra river basin in India since 1985.,” Journal of environmental management, vol. 206, pp. 1192-1203, 2018.
[14] M. Salvaris, D. Dean and W. H. Tok, “Generative adversarial networks.,” in In Deep Learning with Azure, Berkeley, CA., 2018.
[15] J. Feng, H. Yu, L. Wang, X. Cao, X. Zhang and L. Jiao, “Classification of hyperspectral images based on multiclass spatial–spectral generative adversarial networks.,” IEEE Transactions on Geoscience and Remote Sensing, vol. 57, no. 8, pp. 5329-5343, 2019.
[16] P. Yan, F. He, Y. Yang and F. Hu, “Semi-supervised representation learning for remote sensing image classification based on generative adversarial networks.,” in IEEE Access, 2020.
[17] J. M. Haut, M. E. Paoletti, J. Plaza, A. Plaza and J. Li, “Hyperspectral image classification using random occlusion data augmentation.,” in IEEE Geoscience and Remote Sensing Letters, 2019.
[18] Y. Bazi, L. Bashmal, M. M. A. Rahhal, R. A. Dayil and N. A. Ajlan, “Vision transformers for remote sensing image classification,” in Remote Sensing, 2021.
[19] J. Song, S. Gao, Y. Zhu and C. Ma, “A survey of remote sensing image classification based on CNNs.,” Big earth data, vol. 3, no. 3, pp. 232-254, 2019.
[20] C. Wang, L. Zhang, W. Wei and Y. Zhang, “Hyperspectral image classification with data augmentation and classifier fusion.,” in IEEE Geoscience and Remote Sensing Letters, 17(8), 1420-1424, 2019.
[21] R. Stivaktakis, G. Tsagkatakis and P. Tsakalides, “Deep learning for multilabel land cover scene categorization using data augmentation.,” in IEEE Geoscience and Remote Sensing Letters, 2019.
[22] A. N. Abbasi and M. He, “Convolutional neural network with PCA and batch normalization for hyperspectral image classification,” in IEEE International Geoscience and Remote Sensing Symposium, 2019.
[23] R. Vaddi and P. Manoharan, “Hyperspectral image classification using CNN with spectral and spatial features integration.,” Infrared Physics & Technology, vol. 107, no. 103296, 2020.
[24] J. Li and B. Y. Liang, “A hybrid neural network for hyperspectral image classification.,” in Remote Sensing Letters, 2020.