An Efficient Survey on Predictive Analytics in Big Data
S. Chitra1 , P. Srivaramanga2
Section:Review Paper, Product Type: Journal Paper
Volume-06 ,
Issue-02 , Page no. 112-117, Mar-2018
Online published on Mar 31, 2018
Copyright © S. Chitra, P. Srivaramanga . This is an open access article distributed under the Creative Commons Attribution License, which permits unrestricted use, distribution, and reproduction in any medium, provided the original work is properly cited.
View this paper at Google Scholar | DPI Digital Library
How to Cite this Paper
- IEEE Citation
- MLA Citation
- APA Citation
- BibTex Citation
- RIS Citation
IEEE Style Citation: S. Chitra, P. Srivaramanga, “An Efficient Survey on Predictive Analytics in Big Data,” International Journal of Computer Sciences and Engineering, Vol.06, Issue.02, pp.112-117, 2018.
MLA Style Citation: S. Chitra, P. Srivaramanga "An Efficient Survey on Predictive Analytics in Big Data." International Journal of Computer Sciences and Engineering 06.02 (2018): 112-117.
APA Style Citation: S. Chitra, P. Srivaramanga, (2018). An Efficient Survey on Predictive Analytics in Big Data. International Journal of Computer Sciences and Engineering, 06(02), 112-117.
BibTex Style Citation:
@article{Chitra_2018,
author = {S. Chitra, P. Srivaramanga},
title = {An Efficient Survey on Predictive Analytics in Big Data},
journal = {International Journal of Computer Sciences and Engineering},
issue_date = {3 2018},
volume = {06},
Issue = {02},
month = {3},
year = {2018},
issn = {2347-2693},
pages = {112-117},
url = {https://www.ijcseonline.org/full_spl_paper_view.php?paper_id=215},
publisher = {IJCSE, Indore, INDIA},
}
RIS Style Citation:
TY - JOUR
UR - https://www.ijcseonline.org/full_spl_paper_view.php?paper_id=215
TI - An Efficient Survey on Predictive Analytics in Big Data
T2 - International Journal of Computer Sciences and Engineering
AU - S. Chitra, P. Srivaramanga
PY - 2018
DA - 2018/03/31
PB - IJCSE, Indore, INDIA
SP - 112-117
IS - 02
VL - 06
SN - 2347-2693
ER -
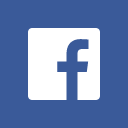
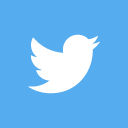
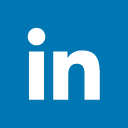
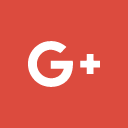
Abstract
Big Data has gained much attention from the academia and the IT industry. In the digital and computing world, information is generated and collected at a rate that rapidly exceeds the boundary range. Currently, over 2 billion people worldwide are connected to the Internet, and over 5 billion individuals own mobile phones. By 2020, 50 billion devices are expected to be connected to the Internet. In the recent times the amount of data are generated and stored by various industries are rapidly increasing on the internet thus data scientists are facing a lot of challenges for maintaining a huge amount of data as the fast growing industries require the significant information for enhancing the business and for predictive analysis of the information. This paper focuses on the various states of art studies towards Big Data Analytics techniques.
Key-Words / Index Term
Big Data Analytics, Hadoop, Map Reduce, Data Center, Hadoop Distributed File System (HDFS)
References
[1] S.Vikram Phaneendra & E.Madhusudhan Reddy “Big Data- solutions for RDBMS problems- A survey” In 12th IEEE/IFIP Network Operations & Management Symposium (NOMS 2010) (Osaka, Japan, Apr 19{23 2013).
[2] Kiran kumara Reddi & Dnvsl Indira “Different Technique to Transfer Big Data : survey” IEEE Transactions on 52(8) (Aug.2013) 2348- 2355.
[3] T. J. Hacker and K. Mahadik. Flexible resource allocation for reliable virtual cluster computing systems. In Proc. ACM Conf. High Perf. Comp., Networking, Storage and Analysis, page 48, 2011.
[4] Jimmy Lin “MapReduce Is Good Enough?” The control project. IEEE Computer 32 (2013).
[5] M. Durairaj, T.S. Poornappriya, “A Review on Big Data Analytics Tools for Telecommunication Industry”, International Journal of Control Theory and Applications, Volume 9, Issue 27, pp.185-193, 2016.
[6] M. A. Salehi, P. Radha Krishna, K. S. Deepak, and R. Buyya. Preemption-aware energy management in virtualized data centers. In Proc. of the 5th IEEE Intl. Conf. on Cloud Computing, pages 844{851, 2012}.
[7] Umasri.M.L, Shyamalagowri.D ,Suresh Kumar.S “Mining Big Data:- Current status and forecast to the future” Volume 4, Issue 1, January 2014 ISSN: 2277 128X.
[8] J. Ekanayake, H. Li, B. Zhang, T. Gunarathne, S.-H. Bae, J. Qiu, and G. Fox. Twister: a runtime for iterative mapreduce. In Proc. 19th ACM Int`l Symp. High Performance Distr. Comp., pages 810-818, 2010.
[9] Sameer Agarwal, Barzan Mozafari, Aurojit Panda, Henry Milner, Samuel Madden, Ion Stoica “BlinkDB: Queries with Bounded Errors and Bounded Response Times on Very Large Data” Copyright © 2013ì ACM 978-1-4503-1994 2/13/04.
[10] Y. Song, Y. Sun, and W. Shi. A two-tiered on-demand resource allocation mechanism for vm-based data centers. IEEE Transactions on Services Computing, 6(1):116{129, 2013.
[11] M. Zaharia, A. Konwinski, A. D. Joseph, R. Katz, and I. Stoica. Improving mapreduce performance in heterogeneous environments. In Proc. of the 8th USENIX Conf. on Operating systems design and implementation, pages 29{42, 2008.
[12] Anitya Kumar Gupta, Srishti Gupta, "Security Issues in Big Data with Cloud Computing", International Journal of Scientific Research in Computer Science and Engineering, Vol.5, Issue.6, pp.27-32, 2017
[13] S. Sathyamoorthy, "Data Mining and Information Security in Big Data", International Journal of Scientific Research in Computer Science and Engineering, Vol.5, Issue.3, pp.86-91, 2017
[14] Z. Ren, X. Xu, M. Zhou, J. Wan, and W. Shi. Workload analysis, implications and optimization on a production hadoop cluster: A case study on taobao. IEEE Transactions on Services Computing, 7(2):307{321, 2014
[15] Albert Bifet “Mining Big Data In Real Time” Informatica 37 (2013) 15–20 DEC 2012.
[16] F. Tian and K. Chen. Towards optimal resource provisioning for running mapreduce programs in public clouds. In Proc. IEEE Int`l Conf. on Cloud Computing, pages 155-162, 2011.
[17] V.K. Gujare, P. Malviya, "Big Data Clustering Using Data Mining Technique", International Journal of Scientific Research in Computer Science and Engineering, Vol.5, Issue.2, pp.9-13, 2017
[18] J. Zhan, L. Wang, X. Li, W. Shi, C. Weng, W. Zhang, and X. Zang. Cost-aware cooperative resource provisioning for heterogeneous workloads in data centers. IEEE Transactions on Computers, 62(11):2155-2168, 2013.