Sentiment Analysis Methods on Web Predication
S.Karthikeyan 1 , P.Srivaramangai 2
Section:Review Paper, Product Type: Journal Paper
Volume-06 ,
Issue-02 , Page no. 130-134, Mar-2018
Online published on Mar 31, 2018
Copyright © S.Karthikeyan, P.Srivaramangai . This is an open access article distributed under the Creative Commons Attribution License, which permits unrestricted use, distribution, and reproduction in any medium, provided the original work is properly cited.
View this paper at Google Scholar | DPI Digital Library
How to Cite this Paper
- IEEE Citation
- MLA Citation
- APA Citation
- BibTex Citation
- RIS Citation
IEEE Style Citation: S.Karthikeyan, P.Srivaramangai, “Sentiment Analysis Methods on Web Predication,” International Journal of Computer Sciences and Engineering, Vol.06, Issue.02, pp.130-134, 2018.
MLA Style Citation: S.Karthikeyan, P.Srivaramangai "Sentiment Analysis Methods on Web Predication." International Journal of Computer Sciences and Engineering 06.02 (2018): 130-134.
APA Style Citation: S.Karthikeyan, P.Srivaramangai, (2018). Sentiment Analysis Methods on Web Predication. International Journal of Computer Sciences and Engineering, 06(02), 130-134.
BibTex Style Citation:
@article{_2018,
author = {S.Karthikeyan, P.Srivaramangai},
title = {Sentiment Analysis Methods on Web Predication},
journal = {International Journal of Computer Sciences and Engineering},
issue_date = {3 2018},
volume = {06},
Issue = {02},
month = {3},
year = {2018},
issn = {2347-2693},
pages = {130-134},
url = {https://www.ijcseonline.org/full_spl_paper_view.php?paper_id=219},
publisher = {IJCSE, Indore, INDIA},
}
RIS Style Citation:
TY - JOUR
UR - https://www.ijcseonline.org/full_spl_paper_view.php?paper_id=219
TI - Sentiment Analysis Methods on Web Predication
T2 - International Journal of Computer Sciences and Engineering
AU - S.Karthikeyan, P.Srivaramangai
PY - 2018
DA - 2018/03/31
PB - IJCSE, Indore, INDIA
SP - 130-134
IS - 02
VL - 06
SN - 2347-2693
ER -
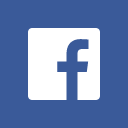
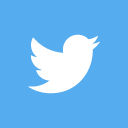
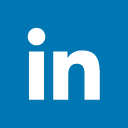
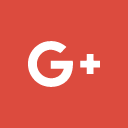
Abstract
World Wide Web is a huge warehouse of web pages and links. It offers large quantity of data for the Internet users. The growth of web is incredible as around one million pages are added per day. Users’ accesses are recorded in weblogs. Web usage mining is a variety of mining technique in logs. Because of the outstanding usage, the log files are growing at a faster rate and the size is suitable very large. This leads to the complexity for mining the practice log according to the needs. This provides a vast field for the researchers to supply their proposal to develop a better mining technique. In this paper, we analyse and study Markov model and allKth Markov model in Web prediction. We propose a new customized Markov model to ease the issue of scalability in the number of paths. In adding, we there a new two-tier prediction structure that creates an example classifier EC, based on the training examples and the generated classifiers. We show that such framework can advance the prediction time without compromising prediction accuracy. We have used standard benchmark data sets to analyze, compare, and demonstrate the effectiveness of our techniques using variations of Markov models and relationship rule mining. Our experiments demonstrate the effectiveness of our modified Markov model in reducing the number of paths without compromising accuracy. Additionally, the results support our investigation conclusions that accuracy improves with higher orders of all-Kth model
Key-Words / Index Term
Big Data Sentiment Analysis
References
[1] Dembczynski, K., Kotłowski, W., Sydow, M.: Effective Prediction of Web User Behaviour with User-Level Models, 2007.
[2] M. Awad, L. Khan, and B. Thuraisingham, “Predicting WWW surfing using multiple evidence combination,” VLDB J., vol. 17, no. 3, pp. 401–417, May 2008.
[3] Marcelo Maia, Jussara Almeida, and Virg ılioAlmeida . Identifying user behavior in online social networks. In Proceedings of the 1st Workshop on Social Network Systems ,Social Nets’08,pages1–6, NewYork, NY, USA, 2008. ACM.
[4] M. T. Hassan, K. N. Junejo, and A. Karim, “Learning and predicting key Web navigation patterns using Bayesian models,” in Proc. Int. Conf. Comput. Sci. Appl. II, Seoul, Korea, 2009, pp. 877–887.
[5] HAUGER, D., PARAMYTHIS, A., AND WEIBELZAHL, S. 2011. Using browser interaction data to determine page reading behavior. In Proceedings of the 19th International Conference on User Modelling, adaption, and Personalization (UMAP).147–158
[6] LEIVA, L. A. 2011. Mining the browsing context: Discovering interaction profiles via behavioral clustering. In Adjunct Proceedings of the 19th Conference on User Modeling, Adaptation, and Personalization (UMAP) . 31–33
[7] GUO,Q. AND A GICHTEIN , E. 2012. Beyond dwell time: Estimating document relevance from cursor movements and other post-click searcher behaviour. In Proceedings of the 21st International Conference on World Wide Web (WWW). 569–578.