Ensemble Forecasting Through Learning Process
K.Amudha 1 , S.Padmapriya 2 , D.Saravanan 3
Section:Research Paper, Product Type: Journal Paper
Volume-06 ,
Issue-02 , Page no. 332-335, Mar-2018
Online published on Mar 31, 2018
Copyright © K.Amudha, S.Padmapriya, D.Saravanan . This is an open access article distributed under the Creative Commons Attribution License, which permits unrestricted use, distribution, and reproduction in any medium, provided the original work is properly cited.
View this paper at Google Scholar | DPI Digital Library
How to Cite this Paper
- IEEE Citation
- MLA Citation
- APA Citation
- BibTex Citation
- RIS Citation
IEEE Style Citation: K.Amudha, S.Padmapriya, D.Saravanan, “Ensemble Forecasting Through Learning Process,” International Journal of Computer Sciences and Engineering, Vol.06, Issue.02, pp.332-335, 2018.
MLA Style Citation: K.Amudha, S.Padmapriya, D.Saravanan "Ensemble Forecasting Through Learning Process." International Journal of Computer Sciences and Engineering 06.02 (2018): 332-335.
APA Style Citation: K.Amudha, S.Padmapriya, D.Saravanan, (2018). Ensemble Forecasting Through Learning Process. International Journal of Computer Sciences and Engineering, 06(02), 332-335.
BibTex Style Citation:
@article{_2018,
author = {K.Amudha, S.Padmapriya, D.Saravanan},
title = {Ensemble Forecasting Through Learning Process},
journal = {International Journal of Computer Sciences and Engineering},
issue_date = {3 2018},
volume = {06},
Issue = {02},
month = {3},
year = {2018},
issn = {2347-2693},
pages = {332-335},
url = {https://www.ijcseonline.org/full_spl_paper_view.php?paper_id=261},
publisher = {IJCSE, Indore, INDIA},
}
RIS Style Citation:
TY - JOUR
UR - https://www.ijcseonline.org/full_spl_paper_view.php?paper_id=261
TI - Ensemble Forecasting Through Learning Process
T2 - International Journal of Computer Sciences and Engineering
AU - K.Amudha, S.Padmapriya, D.Saravanan
PY - 2018
DA - 2018/03/31
PB - IJCSE, Indore, INDIA
SP - 332-335
IS - 02
VL - 06
SN - 2347-2693
ER -
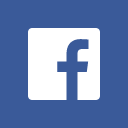
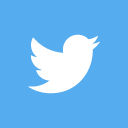
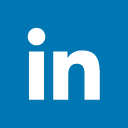
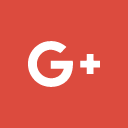
Abstract
Ensemble forecasting is a widely-used numerical prediction method for modeling the progression of nonlinear dynamic systems. To calculate the future state of such systems, a set of ensemble member forecasts is generated from several runs of computer models, where each run is obtained by disquieting the starting condition or using a different model representation of the system. The ensemble mean or median is typically chosen as a point approximation for the ensemble member forecasts. These approaches are limited in that they assume each ensemble member is equally skillful and may not conserve the temporal autocorrelation of the predicted time series. To overcome these confines, new scheme present an online multi-task learning formation called ORION to estimate the optimal weights for combining the ensemble portion forecasts. Unlike other existing formulations, the proposed framework is original in that its learning algorithm must backtrack and modify its previous forecasts before making future predictions if the earlier forecasts were incorrect when verified against new observation data. We termed this strategy as online learning with restart. Our proposed framework employs a graph Laplacian regularize to ensure consistency of the predicted time series. It can also accommodate unusual types of loss functions, including insensitive and loss functions, the latter of which is particularly useful for extreme value prediction. A theoretical proof representative the convergence of our algorithm is also given. Tentative results on seasonal soil moisture forecasts from major river basins in North America exhibit the authority of ORION compared to other baseline algorithms.
Key-Words / Index Term
Online learning, multi-task learning, ensemble forecasting
References
[1] C. Tebaldi and R. Knutti, “The use of the multi-model ensemble in probabilistic climate projections,” Philosoph. Trans. Roy. Soc. London A: Math. Phys. Eng. Sci., vol. 365, no. 1857, pp. 2053–2075, 2007.
[2] P. Cantelaube and J.-M. Terres, “Seasonal weather forecasts for crop yield modeling in Europe,” Tellus A, vol. 57, no. 3, pp. 476– 487, 2005.
[3] M. B. Araujo and M. New, “Ensemble forecasting of species distributions,” Trends Ecology Evol., vol. 22, no. 1, pp. 42–47, 2007.
[4] L. Luo and E. F. Wood, “Use of Bayesian merging techniques in a multimodel seasonal hydrologic ensemble prediction system for the Eastern United States,” J. Hydrometeorology, vol. 9, pp. 866– 884, 2008.
[5] M. Leutbecher and T. Palmer, “Ensemble forecasting,” J. Comput. Phys., vol. 227, pp. 3515–3539, 2008.
[6] X.-T. Yuan and S. Yan, “Visual classification with multi-task joint sparse representation,” in Proc. IEEE Comput. Soc. Conf. Comput. Vis. Pattern Recognit., 2010, pp. 3493–3500.
[7] X. Wang, C. Zhang, and Z. Zhang, “Boosted multi-task learning for face verification with applications to Web image and video search,” in Proc. IEEE Conf. Comput. Vis. Pattern Recognit., 2009, pp. 142–149.
[8] J. Zhou, L. Yuan, J. Liu, and J. Ye, “A multi-task learning formulation for predicting disease progression,” in Proc. 17th ACM SIGKDD Int. Conf. Knowl. Discovery Data Mining, 2011, pp. 814– 822.
[9] S. Bickel, J. Bogojeska, T. Lengauer, and T. Scheffer, “Multi-task learning for HIV therapy screening,” in Proc. 25th Int. Conf. Mach. Learn., 2008, pp. 56–63.
[10] X. Ning and G. Karypis, “Multi-task learning for recommender system,” in Proc. 2nd Asian Conf. Mach. Learn., 2010, pp. 269–284.
[11] J. Wang, S. C. Hoi, P. Zhao, and Z.-Y. Liu, “Online multi-task collaborative filtering for on-the-fly recommender systems,” in Proc. 7th ACM Conf. Recommender Syst., 2013, pp. 237–244. [12] R. K. Ando and T. Zhang, “A framework for learning predictive structures from multiple tasks and unlabeled data,” J. Mach. Learm. Res., vol. 6, pp. 1817–1853, Dec. 2005.
[13] G. Obozinski, B. Taskar, and M. Jordan, “Joint covariate selection and joint subspace selection for multiple classification problems,” Statist. Comput., vol. 20, no. 2, pp. 231–252, 2010.
[14] J. Xu, P.-N. Tan, and L. Luo, “ORION: Online regularized multItask regressiON and its application to ensemble forecasting,” in Proc. IEEE Int. Conf. Data Mining, 2014, pp. 1061–1066.
[15] H. Cheng, P.-N. Tan, G. Jing, and J. Scripps, “Multi-step ahead time series prediction,” in Proc. 10th Pacific-Asia Conf. Advances Knowl. Discovery Data Mining, 2006, pp. 765–774.