MRI Image Brain Tumor Detection and Segmentation
T. Chithambaram1
Section:Research Paper, Product Type: Journal Paper
Volume-06 ,
Issue-02 , Page no. 336-346, Mar-2018
Online published on Mar 31, 2018
Copyright © T. Chithambaram . This is an open access article distributed under the Creative Commons Attribution License, which permits unrestricted use, distribution, and reproduction in any medium, provided the original work is properly cited.
View this paper at Google Scholar | DPI Digital Library
How to Cite this Paper
- IEEE Citation
- MLA Citation
- APA Citation
- BibTex Citation
- RIS Citation
IEEE Style Citation: T. Chithambaram, “MRI Image Brain Tumor Detection and Segmentation,” International Journal of Computer Sciences and Engineering, Vol.06, Issue.02, pp.336-346, 2018.
MLA Style Citation: T. Chithambaram "MRI Image Brain Tumor Detection and Segmentation." International Journal of Computer Sciences and Engineering 06.02 (2018): 336-346.
APA Style Citation: T. Chithambaram, (2018). MRI Image Brain Tumor Detection and Segmentation. International Journal of Computer Sciences and Engineering, 06(02), 336-346.
BibTex Style Citation:
@article{Chithambaram_2018,
author = {T. Chithambaram},
title = {MRI Image Brain Tumor Detection and Segmentation},
journal = {International Journal of Computer Sciences and Engineering},
issue_date = {3 2018},
volume = {06},
Issue = {02},
month = {3},
year = {2018},
issn = {2347-2693},
pages = {336-346},
url = {https://www.ijcseonline.org/full_spl_paper_view.php?paper_id=262},
publisher = {IJCSE, Indore, INDIA},
}
RIS Style Citation:
TY - JOUR
UR - https://www.ijcseonline.org/full_spl_paper_view.php?paper_id=262
TI - MRI Image Brain Tumor Detection and Segmentation
T2 - International Journal of Computer Sciences and Engineering
AU - T. Chithambaram
PY - 2018
DA - 2018/03/31
PB - IJCSE, Indore, INDIA
SP - 336-346
IS - 02
VL - 06
SN - 2347-2693
ER -
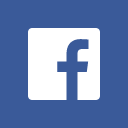
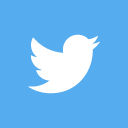
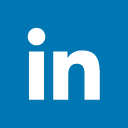
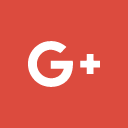
Abstract
Image segmentation is used to extract the abnormal tumor portion in brain. Brain tumor is an abnormal mass of tissue in which cells grow and multiply uncontrollably, apparently unregulated by mechanisms that control cells. Segmentation of brain tissue in the magnetic resonance image (MRI) is very important for detecting and existence of outlines the brain tumor. In this research an algorithm for segmentation based on the symmetry character of brain image is presented. Our goal is to detect the position and edge of tumors automatically. Restorative picture dissection What`s more preparing need extraordinary importance in the field about medicine, particularly clinched alongside non-invasive medicine and clinical study. It aides those doctors to visualize Furthermore examine the picture to see abnormalities previously, internal structures. This suggested system comprises for four phases. Over 1st phase MRI picture is procured toward utilizing MATLAB. In the second stage pre-processing need been done. This pre-processed MRI cerebrum picture is normalized also improved to attain computational consistency. In the third stage high back commotion parts are evacuated toward suitableness filters. For fourth stage the tumor a piece need been fragmented utilizing successful hereditary calculation and the execution Investigation need been made.
Key-Words / Index Term
MRI, Segmentation, Genetic Algorithm, Morphological operations.
References
[1] Anithadevi D, Perumal K. Brain tumor extraction based on segmentation. IJRITCC. 2014; 2(9):2682–9.
[2] Anithadevi D, Perumal K. Generic evaluation of automat¬ic segmentation with seed selection. National Conference
Contemporary Developments in Information and Commu¬nication Technologies; Madurai. 2014. p. 14 24.
[3] Anithadevi D, Perumal K. Novel approach for noise remov¬al of brain tumor MRI images. JBEMi. 2015; 2(3):1–14.
[5] Chang-Tsun Li and Randy Chiao (2003), ‘Multiresolution genetic clustering algorithm for texture segmentation’, Image and
Vision Computing, Vol. 21, pp. 955 - 966.
[6]. Kim E.Y., Park S.H. and Kim H.J. (2000), ‘A genetic algorithm-based segmentation of Markov random field modeled
images’, Signal Processing Letters, IEEE, Digital Object Identifier10.1109/ 97.873564. Vol. 7, No. 11, pp. 301- 315.
[7]. Minglun Gong and Yee-Hong Yang (2001), ‘Genetic-based multiresolution color image segmentation’, Vision Interface, pp. 141–148.
[8]. Nordin P. and Banzhaf W. (1996), ‘Programmatic compression of images and sound’, In Genetic Programming 1996,Proceedings of the First
Annual Conference, Koza J. R. Editors, MIT Press, pp. 345-350.
[9] Ou Y., Cheng W. and Han Ferng-ching (2004), ‘Based on genetic algorithm fuzzy c- means clustering algorithm’, Journal of Chongqing
University, Vol. 27 No. 6, pp. 89 - 926 . Philippe Andrey (1999), ‘Genetic algorithms applied to image segmentation’ Image and Vision Computing, Vol. 17, pp.175- 187.
[10] M. S. Yang, Y. J. Hu, Karen, C. R. Lin and C. C. Lin, ―Segmentation techniques for tissue differentiation in MRI of Ophthalmology using fuzzy clustering algorithms,‖ Magnetic Resonance Imaging, vol. 20, issue 2, pp. 173-179, 2002.
[11] G. C. Karmakar and L. S. Dooley, ―A generic fuzzy rule based image segmentation algorithm,‖ Pattern Recognition Letters, vol. 23, issue 10, pp. 1215-1227, 2002.
[12] D. L. Pham and J. L. Prince, ―An adaptive fuzzy C-means algorithm for image segmentation in the presence of intensity inhomogeneities,‖ Pattern Recognition Letters, vol. 20, issue 1, pp. 57–68, 1999.
[13] Y. A. Tolias and S. M. Panas, ―On applying spatial constraints in fuzzy image clustering using a fuzzy rule-based system,‖ IEEE Signal Processing Letters, vol. 5, issue 10, pp. 245–247, 1998.
[14] Y. A. Tolias and S. M. Panas, ―Image segmentation by a fuzzy clustering algorithm using adaptive spatially constrained membership functions,‖ IEEE Transactions on Systems, Man, and Cybernetics - Part A: Systems and Humans, vol. 28, issue 3, pp. 359–369, 1998.
[15] A. W. C. Liew, S. H. Leung, and W. H. Lau, ―Fuzzy image clustering incorporating spatial continuity,‖ IEE Proceedings - Vision, Image and Signal Processing, vol. 147, issue 2, pp. 185–192, 2000.
[16] D. L. Pham, ―Fuzzy clustering with spatial constraints,‖ in IEEE Proceedings International Conference on Image Processing, New York, II-65–II-68, 2002.
[17] M. N. Ahmed, S. M. Yamany, N. Mohamed, A. A. Farag, T. Moriarty, ―A modified fuzzy C-means algorithm for bias field estimation and segmentation of MRI data,‖ IEEE Transactions on Medical Imaging, vol. 21, issue 3, pp. 193–199, 2002.
[18] S. C. Chen and D. Q. Zhang, ―Robust image segmentation using FCM with spatial constraints based on new kernel-induced distance measure,‖ IEEE Transactions on Systems, Man, and Cybernetics, Part B (Cybernetics), vol. 34, issue 4, pp. 1907-1916, 2004.
[19] L. Szilágyi, Z. Benyó, S. M. Szilágyii, H. S. Adam, and M. R. Brain ―Image segmentation using an enhanced fuzzy C-means algorithm,‖ in 25th Annual International Conference of IEEE EMBS, pp. 17-21, 2003.
[20] M. N. Ahmed, S. M. Yamany, N. A. Mohamed, A. A. Farag, and T. Moriarty, ―Bias field estimation and adaptive segmentation of MRI data using modified fuzzy C-means algorithm,‖ IEEE Computer Society Conference on Computer Vision and Pattern Recognition, vol. 1, pp. 250–255, 1999.