Domestic water demand predicting by factors analysis of planned colony in Ajmer, Rajasthan (India)
Ganpat Singh1 , Arun Goel2 , Mahender Choudhary3
Section:Research Paper, Product Type: Journal Paper
Volume-05 ,
Issue-02 , Page no. 32-40, Dec-2017
CrossRef-DOI: https://doi.org/10.26438/ijcse/v5si2.3240
Online published on Dec 31, 2017
Copyright © Ganpat Singh, Arun Goel , Mahender Choudhary . This is an open access article distributed under the Creative Commons Attribution License, which permits unrestricted use, distribution, and reproduction in any medium, provided the original work is properly cited.
View this paper at Google Scholar | DPI Digital Library
How to Cite this Paper
- IEEE Citation
- MLA Citation
- APA Citation
- BibTex Citation
- RIS Citation
IEEE Style Citation: Ganpat Singh, Arun Goel , Mahender Choudhary, “Domestic water demand predicting by factors analysis of planned colony in Ajmer, Rajasthan (India),” International Journal of Computer Sciences and Engineering, Vol.05, Issue.02, pp.32-40, 2017.
MLA Style Citation: Ganpat Singh, Arun Goel , Mahender Choudhary "Domestic water demand predicting by factors analysis of planned colony in Ajmer, Rajasthan (India)." International Journal of Computer Sciences and Engineering 05.02 (2017): 32-40.
APA Style Citation: Ganpat Singh, Arun Goel , Mahender Choudhary, (2017). Domestic water demand predicting by factors analysis of planned colony in Ajmer, Rajasthan (India). International Journal of Computer Sciences and Engineering, 05(02), 32-40.
BibTex Style Citation:
@article{Singh_2017,
author = {Ganpat Singh, Arun Goel , Mahender Choudhary},
title = {Domestic water demand predicting by factors analysis of planned colony in Ajmer, Rajasthan (India)},
journal = {International Journal of Computer Sciences and Engineering},
issue_date = {12 2017},
volume = {05},
Issue = {02},
month = {12},
year = {2017},
issn = {2347-2693},
pages = {32-40},
url = {https://www.ijcseonline.org/full_spl_paper_view.php?paper_id=296},
doi = {https://doi.org/10.26438/ijcse/v5i2.3240}
publisher = {IJCSE, Indore, INDIA},
}
RIS Style Citation:
TY - JOUR
DO = {https://doi.org/10.26438/ijcse/v5i2.3240}
UR - https://www.ijcseonline.org/full_spl_paper_view.php?paper_id=296
TI - Domestic water demand predicting by factors analysis of planned colony in Ajmer, Rajasthan (India)
T2 - International Journal of Computer Sciences and Engineering
AU - Ganpat Singh, Arun Goel , Mahender Choudhary
PY - 2017
DA - 2017/12/31
PB - IJCSE, Indore, INDIA
SP - 32-40
IS - 02
VL - 05
SN - 2347-2693
ER -
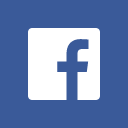
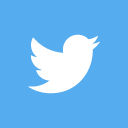
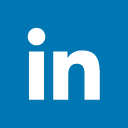
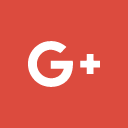
Abstract
In this paper, contribution of variables of domestic urban water demand considered for the purpose of prediction of urban water supply in planned colonies of the City of Ajmer. The data for these 15 variables are entered in the factor analysis under principal component, generalised least square and maximum likelihood method, and five factors/variables are extracted, comprising combinations of these 15 variables. Based on these five principal components (PCs) variables a multi linear regression (PCR) coefficient of 0.76, 0.64 and 0.62 are obtained by the principal component, generalised least square and maximum likelihood model respectively. These five significant principal components are further fed into a multilayer perceptron neural network (NN) model for water demand forecasting. The linear regression coefficient of MLP NN (PCs) is 0.76, very close to the principal component multiple linear regression (stepwise) coefficient of 0.76, and verifying the dependence of water demand on these five principal components. The better result is showing by factor analysis under PCR compare to the factor analysis under Maximum Likelihood and Generalised Least Square methods. The lowest average percentage difference of predicted value is -2.64 PCs MLP (NN) and -2.08 were giving by PCR (PC) respectively. The outcome of the study suggests that the extracted variables are significant for estimation of water demand for planned colonies of Ajmer city.
Key-Words / Index Term
Factor analysis, (PCA), generalised least square (GLS), maximum likelihood (MLH), principal component regression (PCR), neural network (NN), domestic water demand
References
[1]. Adamowski, J. and Karapataki, C. 2010. Comparison of multivariate regression and artificial neural networks for peak urban water demand forecasting: Evaluation of different ANN learning algorithms. J Hydrolo Eng, 15(10), 729–743.
[2]. Adamowski, J., Adamowski, K. and Prokoph, A. 2013. A spectral analysis based methodology to detect climatologically influences on daily urban water demand. Mathematical Geosciences, 45(1), 49–68.
[3]. Antonio, M. and Mario, N. 2011. Long-run water demand estimation: habits, adjustment dynamics, and structural breaks. Applied Economics, 43(17), 2111–2127.
[4]. Arbues, F., Garcia-Valinas, M. and Martinez-Espineira, R. 2003. Estimation of residential water demand: A state-of-the-art review. J Soc Econ, 32(1), 81–102.
[5]. Babel, M. S. and Shinde, V. R. 2011. Identifying prominent explanatory variables for water demand prediction using artificial neural networks: a case study of Bangkok. Water Resour Manage, 25, 1653–1676.
[6]. Billings, B. and Jones, C. 2008. Forecasting urban water demands (2nd edn). American Water Works Association, Washington, DC.
[7]. Choi, T., Kwon, O. and Koo, J. 2010. Water demand forecasting by characteristics of city using principal component and cluster analyses. Environ Eng Res, 15(3), 135–140.
[8]. Choudhary, M., Sharma, R. and Sudhir, K. 2012. Development of residential water demand model for a densely populated area of Jaipur city, India. J Water, Sanitation, and Hygiene for Development, 2(1), 10–18.
[9]. Donkor, E. A., Thomas, A. M., Soyer, R. and Roberson, J. A. 2014. Urban water demand forecasting: A review of methods and models. J Water Resour Plan Manage, 140(2), 146–159.
[10]. Field, C. B., Barros, V., Stocker, T. F., and Qin, D. 2012. Managing the risks of extreme events and disasters to advance climate change adaptation. A special report of working groups I and II of the Intergovernmental Panel on Climate Change (IPCC). Cambridge University Press, Cambridge, UK, and New York.
[11]. Ghiassi, M., Zimbra, D. K. and Saidane, H. 2008. Urban water demand forecasting with a dynamic artificial neural network model. J Water Resour Plan Manage, 134(2), 138–146.
[12]. Ghimire, M., Tracy, A. B., Chanjin, C. and Justin, Q. M. 2015. Estimation of residential water demand under uniform volumetric water pricing. J Water Resour Plan Manage, 142: 1–6. DOI:10.1061/(ASCE)WR.1943-5452.0000580,.
[13]. Herrera M., Torgo L., Izquierdo J. & Perez-garcia R. 2011 Predictive models for forecasting hourly urban water demand. J Hydrol, 387(1–2), 141–150.
[14]. Hinton, P. R., Brownlow, C., McMurray, I. and Cozens, B. 2012. SPSS Explained. Routledge Taylor & Francis Group, London, UK, and New York.
[15]. IBM, (2011) “SPSS Neural Networks 20.” Copyright IBM Corporation 1989, 2011.
[16]. Jain, A., Varshney, A. and Joshi, U. 2001. Short-term water demand forecast modeling at IIT, Kanpur using artificial neural networks. Water Resour Manage, 15(5), 299–321.
[17]. Khatri, K. and Vairavamoorthy, K. 2009. Water demand forecasting for the city of the future against the uncertainties and the global change pressure: Case of Birmingham, World Environmental and Water Resources Congress 2009, May 17-21, Kansas City, MO, USA. DOI: 10.1061/41036(342)523.
[18]. Odan, F. K. and Reisl, F. R. 2012. Hybrid water demand forecasting model associating artificial neural network with Fourier series. J Water Resour Plan Manage, 138(3), 245–256.
[19]. Singh, G., Goel, A. and Choudhary, M. 2015. An inventory of methods and models for domestic water demand forecasting: a review. J of Indian Water Resources Society, 35(3), 34–45.
[20]. Singh, G., Goel, A. and Choudhary, M. 2017. Analysis of domestic water demand variables of a residential colony in Ajmer, Rajasthan (India). Journal of Water Sanitation and Hygiene for Development, DOI: 10.2166/washdev.2017.020.
[21]. Wu, H., Mckay, J. and Hemphill, E. 2010. Roles of levies for sustainable domestic water consumption, Water Resour Manage, 25(3), 929–940.
[22]. Yurdusev, M. A. and Kumanhoglu, A. A. 2007. Survey-based estimation of domestic water saving potential in the case of Manisa city. Water Resour Manage, 22, 291–305.