Comparative Analysis of Roughness with Maximum Dependency Attribute
M.Jancy Rani1 , A. Pethalakshmi2
Section:Review Paper, Product Type: Journal Paper
Volume-06 ,
Issue-04 , Page no. 145-149, May-2018
Online published on May 31, 2018
Copyright © M.Jancy Rani, A. Pethalakshmi . This is an open access article distributed under the Creative Commons Attribution License, which permits unrestricted use, distribution, and reproduction in any medium, provided the original work is properly cited.
View this paper at Google Scholar | DPI Digital Library
How to Cite this Paper
- IEEE Citation
- MLA Citation
- APA Citation
- BibTex Citation
- RIS Citation
IEEE Citation
IEEE Style Citation: M.Jancy Rani, A. Pethalakshmi, “Comparative Analysis of Roughness with Maximum Dependency Attribute,” International Journal of Computer Sciences and Engineering, Vol.06, Issue.04, pp.145-149, 2018.
MLA Citation
MLA Style Citation: M.Jancy Rani, A. Pethalakshmi "Comparative Analysis of Roughness with Maximum Dependency Attribute." International Journal of Computer Sciences and Engineering 06.04 (2018): 145-149.
APA Citation
APA Style Citation: M.Jancy Rani, A. Pethalakshmi, (2018). Comparative Analysis of Roughness with Maximum Dependency Attribute. International Journal of Computer Sciences and Engineering, 06(04), 145-149.
BibTex Citation
BibTex Style Citation:
@article{Rani_2018,
author = {M.Jancy Rani, A. Pethalakshmi},
title = {Comparative Analysis of Roughness with Maximum Dependency Attribute},
journal = {International Journal of Computer Sciences and Engineering},
issue_date = {5 2018},
volume = {06},
Issue = {04},
month = {5},
year = {2018},
issn = {2347-2693},
pages = {145-149},
url = {https://www.ijcseonline.org/full_spl_paper_view.php?paper_id=371},
publisher = {IJCSE, Indore, INDIA},
}
RIS Citation
RIS Style Citation:
TY - JOUR
UR - https://www.ijcseonline.org/full_spl_paper_view.php?paper_id=371
TI - Comparative Analysis of Roughness with Maximum Dependency Attribute
T2 - International Journal of Computer Sciences and Engineering
AU - M.Jancy Rani, A. Pethalakshmi
PY - 2018
DA - 2018/05/31
PB - IJCSE, Indore, INDIA
SP - 145-149
IS - 04
VL - 06
SN - 2347-2693
ER -
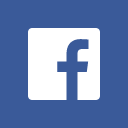
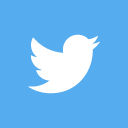
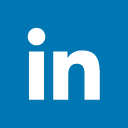
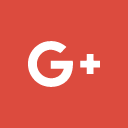
Abstract
Rough set theory is a powerful mathematical tool that has been applied widely to extract knowledge from many databases. It deals with inexact and incomplete data. Cluster analysis means finding similarities between data according to the characteristics found in the data and grouping similar data objects into clusters that are meaningful, useful, or both. Data clustering techniques are valuable tools for researchers working with large databases of multivariate data. Cluster analysis is used in various applications viz., Pattern Recognition, Data Analysis, Image Processing and so on. This paper analyses Roughness and Maximum Dependency Attribute clustering algorithms that minimizes the need for subjective human intervention and compare the purity analysis between these two methods. Purity analysis percentage is calculated from the result of final clusters. Six datasets are used in this research work for comparing the roughness and maximum dependency attribute algorithm to describe the cluster solution by using the purity analysis (PA).
Key-Words / Index Term
Rough Clustering, Equivalence Classes, Roughness, Maximum Dependency Attribute, Purity Analysis
References
[1] Arun K Pujari, “Data Mining Techniques”, Universities Press (India) Private Limited, 2010.
[2] C. L. Blake and C.J. Merz, “UCI Repository of Machine Learning Databases”, Irvin, University of California, http://www.ics.uci.eduction."/~mlearn/, 1998.
[3] Chandranath Adak, “Rough Clustering Based Unsupervised Image Change Detection”.
[4] J.Han and M.Kamber, “Datamining: Concepts and Techniques”, Morgan Kaufmann Publishers, 1992.
[5] Jun-Hao Zhang, Ming-Hu Ha, Jing Wu, “Implementation of Rough K-Means Clustering Algorithm in MATLAB”, 9th International Conference on Machine Learning and Cybernetics, pp.2084-2087, July 2010.
[6] Manish Joshi, Yiyu Yao, Pawan Lingras, Virendrakumar C.Bhavsar, “Rough, Fuzzy, Interval Clustering For Web Usage Mining”, 10th International Conference on Intelligent Systems Design and Applications, pp.397- 402, 2010.
[7] Pawan Lingras, Rui Yan, Chad West, “Comparison of Conventional And Rough K-Means Clustering”, LNAI 2639, Springer – Verlag Berlin Heidelberg,pp.130-137,2003.
[8] Z. Pawlak, “Rough sets”, International Journal of Computer and Information Sciences, vol. 11, pp. 341–356, 1982.
[9] Pethalakshmi.A, A.Banumathi, “Refinement of K-Means And Fuzzy C-Means”, International Journal of Computer Applications, Volume 39, Paper Number: 17, Feb 2012.
[10] Pethalakshmi.A, A.Banumathi, “A Novel Approach in Clustering via Rough set”, IJSR, vol. 2, Issue 7, July 2013.
[11] Tien-Chin Wang, Lisa Y.Chen, Hsien-Da Lee, “Fuzzy Entropy-Based
Rough Set Approach for Extracting Decision Rules”, pp.5636-5639, IEEE 2007.