Tumorous Slices Classification from MRI Brain Volumes using Block based Features Extraction and Random Forest Classifier
P. Sriramakrishnan1 , T. Kalaiselvi2 , P. Nagaraja3 , K. Mukila4
Section:Research Paper, Product Type: Journal Paper
Volume-06 ,
Issue-04 , Page no. 191-196, May-2018
Online published on May 31, 2018
Copyright © P. Sriramakrishnan, T. Kalaiselvi, P. Nagaraja , K. Mukila . This is an open access article distributed under the Creative Commons Attribution License, which permits unrestricted use, distribution, and reproduction in any medium, provided the original work is properly cited.
View this paper at Google Scholar | DPI Digital Library
How to Cite this Paper
- IEEE Citation
- MLA Citation
- APA Citation
- BibTex Citation
- RIS Citation
IEEE Citation
IEEE Style Citation: P. Sriramakrishnan, T. Kalaiselvi, P. Nagaraja , K. Mukila, “Tumorous Slices Classification from MRI Brain Volumes using Block based Features Extraction and Random Forest Classifier,” International Journal of Computer Sciences and Engineering, Vol.06, Issue.04, pp.191-196, 2018.
MLA Citation
MLA Style Citation: P. Sriramakrishnan, T. Kalaiselvi, P. Nagaraja , K. Mukila "Tumorous Slices Classification from MRI Brain Volumes using Block based Features Extraction and Random Forest Classifier." International Journal of Computer Sciences and Engineering 06.04 (2018): 191-196.
APA Citation
APA Style Citation: P. Sriramakrishnan, T. Kalaiselvi, P. Nagaraja , K. Mukila, (2018). Tumorous Slices Classification from MRI Brain Volumes using Block based Features Extraction and Random Forest Classifier. International Journal of Computer Sciences and Engineering, 06(04), 191-196.
BibTex Citation
BibTex Style Citation:
@article{Sriramakrishnan_2018,
author = {P. Sriramakrishnan, T. Kalaiselvi, P. Nagaraja , K. Mukila},
title = {Tumorous Slices Classification from MRI Brain Volumes using Block based Features Extraction and Random Forest Classifier},
journal = {International Journal of Computer Sciences and Engineering},
issue_date = {5 2018},
volume = {06},
Issue = {04},
month = {5},
year = {2018},
issn = {2347-2693},
pages = {191-196},
url = {https://www.ijcseonline.org/full_spl_paper_view.php?paper_id=379},
publisher = {IJCSE, Indore, INDIA},
}
RIS Citation
RIS Style Citation:
TY - JOUR
UR - https://www.ijcseonline.org/full_spl_paper_view.php?paper_id=379
TI - Tumorous Slices Classification from MRI Brain Volumes using Block based Features Extraction and Random Forest Classifier
T2 - International Journal of Computer Sciences and Engineering
AU - P. Sriramakrishnan, T. Kalaiselvi, P. Nagaraja , K. Mukila
PY - 2018
DA - 2018/05/31
PB - IJCSE, Indore, INDIA
SP - 191-196
IS - 04
VL - 06
SN - 2347-2693
ER -
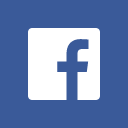
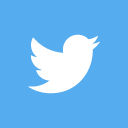
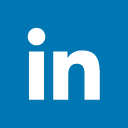
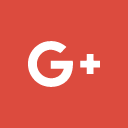
Abstract
The proposed work presents a fully automatic computer-aided diagnosis (CAD) system for magnetic resonance images (MRI) of brain tumor classification. Tumorous slices classification is one of the preprocess steps for brain tumor segmentation and visualization. The proposed work classifies each scan image of MRI volumes into normal or tumorous using block based feature extraction and random forest (RF) classifier. The given image has divided into 8 × 8 non overlapping blocks and extracted three Haralick features such as Energy, inverse difference moment (IDM) and directional moment (DM) from each block. These three extracted features of training blocks are helped to train the RF classifier. The MRI materials used are gathered from multimodal brain tumor segmentation (BraTS 2015) training dataset comprises 274 multisequence MR scans of glioma patients. The experimental results of proposed technique are validated using the measures sensitivity, specificity, accuracy, missed alarm (MA) and false alarm (FA). The average results of the proposed method reached upto 94% of sensitivity, 94% of specificity and 95% of accuracy in BraTS 2015. The error rates measures 1% of slices were missed to identify as tumor and 3% of slices spuriously detected as tumor. The performance of the proposed work was compared with eight existing methods. In summary, the results showed that the proposed method using RF classifier given effective classification for separating normal and tumorous slices from MR brain volumes.
Key-Words / Index Term
Tumor detection, Random forest, Feature extraction, Classification, BraTS dataset
References
[1] D.L. Longo, “369 Seizures and Epilepsy Harrison`s principles of internal medicine”, 18th Ed., McGraw-Hill, New York, pp. 3258, 2012.
[2] J.L. Prince, J.M. Links, “Medical Imaging Signals and Systems”, Pearson Prentice Hall, 2nd Edition, 2014.
[3] J.A. Rodger, “Discovery of medical big data analytics: improving the prediction of traumatic brain injury survival rates by data mining patient informatics processing software hybrid hadoop hive”, Informatics in Medicine Unlocked, Vol. 1, pp. 17–26, 2015.
[4] T. Kalaiselvi, P Sriramakrishnan, “Rapid brain tissue segmentation process by modified FCM algorithm with CUDA enabled GPU machine”, International Journal of Imaging Systems Technology, pp.1–12, 2018. DOI: 10.1002/ima.22267
[5] P.M. Krishnammal, SS Raja, “Automated Brain Image classification using Neural Network Approach and Abnormality Analysis”, International Journal of Engineering and Technology (IJET), Vol. 7, No.3, pp.876-886, 2015.
[6] N. Rajalakshmi, V Lakshmi Prabha, “MRI brain image classification - a hybrid approach”, International Journal of Imaging Systems Technology, Vol. 25, No.3, pp. 226–244, 2015.
[7] R.J. Ramteke, Y. Khachane Monali, “Automatic medical image classification and abnormality detection using K-Nearest Neighbour”, International Journal of Advanced Computer Research, Vol. 2, No.4, pp. 190-196, 2012.
[8] Z. Kapas, L. Lefkovits, L. Szilagyi, “Automatic Detection and Segmentation of Brain Tumor Using Random Forest Approach”, In Modeling Decisions for Artificial Intelligence, pp. 301-312. Springer, Cham, 2016.
[9] M. Rezaei, H. Yang, C. Meinel, “Brain Abnormality Detection by Deep Convolutional Neural Network”, arXiv preprint arXiv:1708.05206. 2017 Aug 17.
[10] V.P. Gladis Pushpa Rathi, S. Palani, “Brain Tumor MRI Image Classification with Feature Selection and Extraction Using Linear Discriminant Analysis”, International Journal of Information Sciences and Techniques (IJIST), Vol.2, No.4, pp.131-146, 2012.
[11] D.R. Nayak, R. Dash, B. Majhi, “Brain MR image classification using two-dimensional discrete wavelet transform and AdaBoost with random forests”, Neurocomputing, Vol. 177, pp. 188-197, 2016.
[12] T. Gupta, P, Manocha, T.K. Gandhi, R.K. Gupta, B.K. Panigrahi, “Tumor Classification and Segmentation of MR Brain Images”, arXiv preprint arXiv:1710.11309, 2017.
[13] S.A. El-Dahshan, T. Hosny, A.M. Salem, “Hybrid intelligent techniques for MRI brain images classification”, Digital Signal Processing, Vol. 20, No. 2, pp. 433-441, 2010.
[14] M. Abdallah, M. Blonski, S. Wantz-Mezieres, Y. Gaudeau, L. Taillandier, J. Moureaux, “On the relevance of two manual tumor volume estimation methods for diffuse low-grade gliomas”, Healthcare Technology Letters, pp. 1-4, 2017.
[15] M. Robert, K. Haralick, K. Shanmugam, I. Dinstein, “Texture features for Image Classification”, IEEE Transactions on Systems and Cybernetics, Vol. 3, No. 6, pp. 610 - 621 1973.
[16] T. Ho, Kam, “Random Decision Forests”,. Proceedings of the 3rd International Conference on Document Analysis and Recognition, Montreal, pp. 278–282, 1995.
[17] T. Kalaiselvi, S. Karthigai selvi, “A Novel Wavelet based Feature Selection to Classify Abnormal Images from T2-w Axial Head Scans”, Proceedings of National Conferences on New Horizons in Computational Intelligence and Information Systems (NHCIIS), India, pp. 140 -145, 2015.
[18] T. Kalaiselvi, P. Sriramakrishnan, “Brain Abnormality Detection from MRI of Human Head Scans using the Bilateral Symmetry Property and Histogram Similarity Measures”, The 20th International Computer Science and Engineering Conference, IEEE explore, Tailand, pp. 14 - 17 December 2016.
[19] R. Anitha, D. Siva Sundhara Raja, “Development of computer-aided approach for brain tumor detection using random forest classifier”, International Journal of Imaging Systems and Technology, pp.1–6, 2017. DOI: 10.1002/ima.22255