Performance Analysis of wavelet Thresholding for Denoising EEG Signal
Dipali Sinha1 , Thangavel K.2
Section:Research Paper, Product Type: Journal Paper
Volume-06 ,
Issue-04 , Page no. 214-218, May-2018
Online published on May 31, 2018
Copyright © Dipali Sinha, Thangavel K. . This is an open access article distributed under the Creative Commons Attribution License, which permits unrestricted use, distribution, and reproduction in any medium, provided the original work is properly cited.
View this paper at Google Scholar | DPI Digital Library
How to Cite this Paper
- IEEE Citation
- MLA Citation
- APA Citation
- BibTex Citation
- RIS Citation
IEEE Style Citation: Dipali Sinha, Thangavel K., “Performance Analysis of wavelet Thresholding for Denoising EEG Signal,” International Journal of Computer Sciences and Engineering, Vol.06, Issue.04, pp.214-218, 2018.
MLA Style Citation: Dipali Sinha, Thangavel K. "Performance Analysis of wavelet Thresholding for Denoising EEG Signal." International Journal of Computer Sciences and Engineering 06.04 (2018): 214-218.
APA Style Citation: Dipali Sinha, Thangavel K., (2018). Performance Analysis of wavelet Thresholding for Denoising EEG Signal. International Journal of Computer Sciences and Engineering, 06(04), 214-218.
BibTex Style Citation:
@article{Sinha_2018,
author = {Dipali Sinha, Thangavel K.},
title = {Performance Analysis of wavelet Thresholding for Denoising EEG Signal},
journal = {International Journal of Computer Sciences and Engineering},
issue_date = {5 2018},
volume = {06},
Issue = {04},
month = {5},
year = {2018},
issn = {2347-2693},
pages = {214-218},
url = {https://www.ijcseonline.org/full_spl_paper_view.php?paper_id=384},
publisher = {IJCSE, Indore, INDIA},
}
RIS Style Citation:
TY - JOUR
UR - https://www.ijcseonline.org/full_spl_paper_view.php?paper_id=384
TI - Performance Analysis of wavelet Thresholding for Denoising EEG Signal
T2 - International Journal of Computer Sciences and Engineering
AU - Dipali Sinha, Thangavel K.
PY - 2018
DA - 2018/05/31
PB - IJCSE, Indore, INDIA
SP - 214-218
IS - 04
VL - 06
SN - 2347-2693
ER -
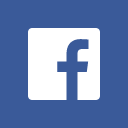
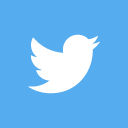
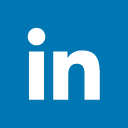
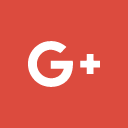
Abstract
Electroencephalogram (EEG) is used for detecting problems in the electrical activity of the brain associated with brain disorders. During acquisition of EEG signals various noises like electrocardiogram (ECG),electromyogram(EMG),electrooculogram(EOG)and power line interference etc. contaminates the signal, which makes the proper analysis of the signal difficult. Therefore, noise removal is an integral part of preprocessing step before signal analysis. In this paper, wavelet transform using different kind of filters like db2, db4, coif2, coif4, sym2 and sym4 is used to decompose the signal into low and high frequency components. Then, high frequency components have been thresholded at each level of decomposition. The denoised signal is reconstructed using the thresholded coefficients and the approximation coefficients. Thresholding methods such as minimaxi, Sure (Heuristic and rigorous) and Square-Root-Log are investigated to compute the threshold value. The coiflet filter at level 4 with minimax thresholding method performed better than other wavelet filters and thresholding methods in terms of Peak Signal-to-Noise Ratio (PSNR) value.
Key-Words / Index Term
Electroencephalogram, Wavelet Transform, Threshold, Denoising, Peak Signal-to-Noise Ratio
References
[1] V. Singh, R. Sharma,“Performance Comparison of Denoising Methods of Electroencephalogram”International Journal of Engineering Research & Technology, Vol.3 Issue.9,2014
[2] M. Mamun, M. Al-Kadi, M. Marufuzzaman,“Effectiveness of Wavelet Denoising on Electroencephalogram Signals” Journal of Applied Research and Technology, Vol.11, pp.156-160, 2013
[3] S. Sudha, G. R. Suresh, R. Sukanesh,“Wavelet based image denoising using adaptive thresholding” In the proceedings of the 2007 International Conference on Computational Intelligence and Multimedia Applications (ICCIMA), pp.296–300, 2007
[4] A. K. Verma, N. Verma,“Performance analysis of wavelet thresholding methods in denoising of audio signals of some indian musical instruments” International Journal of Engineering Science Technology, vol.4, No.5, pp.2047–2052, 2012
[5] V. Krishnaveni, S. Jayaraman, S. Aravind, V. Hariharasudhan, K. Ramadoss,“Automatic identification and removal of ocular artifacts from EEG using wavelet transform”Measurement Science Review, Vol.6, No.4,pp.45-57,2006
[6] P. SenthilKumar, R. Arumuganathan, K. Sivakumar, C. Vimal,“Removal of ocular artifacts in the EEG through wavelet transform without using an EOG reference channel”Int. J. Open Problems Compt. Math., Vol.1, No.3,pp.188-200,2008
[7] N. K. Al-Qazzaz, S. Ali, S. A. Ahmad, M. S. Islam, and M. I. Ariff,“Selection of mother wavelets thresholding methods in denoising multi-channel EEG signals during working memory task”In the proceedings of 2014 IEEE Conference on Biomedical Engineering and Sciences, pp. 214–219, 2014
[8] TP. Jung, S. Makeig, C. Humpheries, T. Lee, M.J. Mckeown, V. Iragui, T.J. Sejnowski,“Removing electroencephalographic artifacts by blind source separation”Psychophysiology 37, pp.163–1782000
[9] S. Lahmiri, M. Boukadoum,“A Weighted Bio-signal Denoising Approach Using Empirical Mode Decomposition”The Korean Society of Medical & Biological Engineering and Springer, Vol.5, Issue.2, pp.131-139,2015
[10] K. Thangavel, K. Sasirekha,“Denoising Iris Image Using a Novel Wavelet Based Threshold” In the proceedings of Digital Connectivity – Social Impact. CSI pp. 57-69, 2016