A Review on Fetal Brain Structure Extraction Techniques from Human MRI Images
S. Vijayalakshmi1 , N. Suresh Kumar2
Section:Review Paper, Product Type: Journal Paper
Volume-06 ,
Issue-04 , Page no. 239-242, May-2018
Online published on May 31, 2018
Copyright © S. Vijayalakshmi, N. Suresh Kumar . This is an open access article distributed under the Creative Commons Attribution License, which permits unrestricted use, distribution, and reproduction in any medium, provided the original work is properly cited.
View this paper at Google Scholar | DPI Digital Library
How to Cite this Paper
- IEEE Citation
- MLA Citation
- APA Citation
- BibTex Citation
- RIS Citation
IEEE Style Citation: S. Vijayalakshmi, N. Suresh Kumar, “A Review on Fetal Brain Structure Extraction Techniques from Human MRI Images,” International Journal of Computer Sciences and Engineering, Vol.06, Issue.04, pp.239-242, 2018.
MLA Style Citation: S. Vijayalakshmi, N. Suresh Kumar "A Review on Fetal Brain Structure Extraction Techniques from Human MRI Images." International Journal of Computer Sciences and Engineering 06.04 (2018): 239-242.
APA Style Citation: S. Vijayalakshmi, N. Suresh Kumar, (2018). A Review on Fetal Brain Structure Extraction Techniques from Human MRI Images. International Journal of Computer Sciences and Engineering, 06(04), 239-242.
BibTex Style Citation:
@article{Vijayalakshmi_2018,
author = {S. Vijayalakshmi, N. Suresh Kumar},
title = {A Review on Fetal Brain Structure Extraction Techniques from Human MRI Images},
journal = {International Journal of Computer Sciences and Engineering},
issue_date = {5 2018},
volume = {06},
Issue = {04},
month = {5},
year = {2018},
issn = {2347-2693},
pages = {239-242},
url = {https://www.ijcseonline.org/full_spl_paper_view.php?paper_id=389},
publisher = {IJCSE, Indore, INDIA},
}
RIS Style Citation:
TY - JOUR
UR - https://www.ijcseonline.org/full_spl_paper_view.php?paper_id=389
TI - A Review on Fetal Brain Structure Extraction Techniques from Human MRI Images
T2 - International Journal of Computer Sciences and Engineering
AU - S. Vijayalakshmi, N. Suresh Kumar
PY - 2018
DA - 2018/05/31
PB - IJCSE, Indore, INDIA
SP - 239-242
IS - 04
VL - 06
SN - 2347-2693
ER -
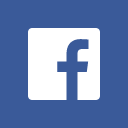
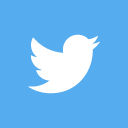
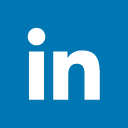
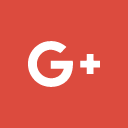
Abstract
Fetal brain magnetic resonance imaging (MRI) is an essential and trivial task to analyze and detect the growth of baby brain abnormalities and possibilities of diseases related to the brain. This Paper starts with different perception and view of different elder’s analysis and techniques such as morphological, voxel classification, Richardson LucyDeconvolution Method, diffusion-weighted and fast furious transform with Fetal Brain MRI. Finally, concluded with the development trend of automated image segmentationtechniques of fetal brain MRI imagesand their comparison.
Key-Words / Index Term
Image Segmentation, fetal brain MRI, Morphological, Voxel Classification,Richardson Lucy Deconvolution Method, Diffusion-Weighted, Fast Furious Transform
References
[1] R Barber et al.,“White matter lesions on magnetic resonance imaging indementia with Lewy bodies, Alzheimer’s disease, vascular dementia, and normal aging”, J Neurol Neurosurg Psychiatry, vol.67,pp.66-72,1999.
[2] Denise Head et al.,”Differential Vulnerability of Anterior White Matter in Nondemented Aging with Minimal Acceleration in Dementia of the Alzheimer Type: Evidence from Diffusion Tensor Imaging”,vol.14,pp.410-423,2004.
[3] Nora D. Volkow, Bruce Rosen, And Lars Farde, “Imaging the living human brain: Magnetic resonance imaging and positron emission tomography”,vol.94, pp. 2787–2788, 1997.
[4] Amit Anand et al., “Brain SPECT Imaging of Amphetamine-Induced Dopamine Release in Euthymic Bipolar Disorder Patients”, vol.157, pp.1108-1114, 2002.
[5] Milan Sonka, Vaclav Hlavac, Roger Boyle, in: “Image Processing: Analysis andMachine Vision”, second ed., Brooks/Cole Publishing Company, 1999.
[6] Brummer, M.E., Mersereau, R.M., Eisner, R.L., and Lewine, R.R.J.., “Automatic detection of brain contours in MRI data sets”, IEEE Trans.Med. Imag. vol.12, pp.153-166, 1993.
[7] Stella Atkins, and Blair T Mackiewich, ”Fully Automatic segmentation of the Brain in MRI”, IEEE transactions on Medical Imaging, vol.17, pp.98-107, 1998.
[8] Somasundaram, K., Kalaiselvi, T., “Fully Automatic Brain Extraction Algorithm for axial T2 Magnetic Resonance Images Computers in Biology and Medicine”, vol.40,pp.811-822,2010.
[9] Gousias, I. S., Edwards, A. D., Rutherford, M. A., Counsell, S. J., Hajnal, J. V., Rueckert, D., and Hammers, A. (2012). “Magnetic resonance imaging of the newborn brain: Manual segmentation of labelled atlases in term-born and preterm infants”, NeuroImage, 62(3):1499-1509.
[10] Habas, P. A., Kim, K., Rousseau, F., Glenn, O. A., Barkovich, A. J., and Studholme, C. (2010b). “Atlas-based segmentation of developing tissues in the human brain with quantitative validation in young fetuses”, Human Brain Mapping, 31(9):1348-1358.
[11] Peterson, B. S., Anderson, A. W., Ehrenkranz, R., Staib, L. H., Tageldin, M., Colson, E., Gore, J. C., Duncan, C. C., Makuch, R., and Ment, L. R. (2003). “Regional brain volumes and their later neurodevelopmental correlates in term and preterm infants”, Pediatrics, 111(5):939-948.
[12] Jones, S. E., Buchbinder, B. R., and Aharon, I. (2000). “Three-dimensional mapping of cortical thickness using Laplace`s Equation. Human Brain Mapping”, 11(1):12-32.
[13] Md. Shakowat Zaman Sarker, Tan Wooi Haw and Rajasvaran Logeswaran,“Morphological based technique for image segmentation”, International Journal of Information Technology, Vol. 14 No. 1
[14] K. Haris and Efstratiadis, “Hybrid Image Segmentation Using Watershed and Fast Region Merging,” IEEE Trans. Image Process., 1998, vol. 7, no. 12, pp. 1684-1699.
[15] D. F. Shen and M. T. Huang, “A Watershed-Based Image Segmentation Using JND Property,” Speech and Signal Process., 2003, pp. 377-380.
[16] Bernhard Kainz, Kevin Keraudren, Vanessa Kyriakopoulou, Mary Rutherford, Joseph V. Hajnal, Daniel Rueckert,”fast fully automatic brain detection in fetal mri Using dense rotation invariant image descriptors”,978-1-4673-1961-4/14/$31.00 ©2014 IEEE
[17] Y. Li and S. Osher, “A new median formula with applications to PDE based denoising,” Commun. Math. Sci.,vol. 7, no. 3, pp. 741–753, 2009.
[18] NVIDIA Corporation, NVIDIA CUDA Compute Unified Device Architecture Programming Guide, NVIDIACorporation, 2012.
[19] K Somasundaram, P A Kalaividya, “Brain Portion Segmentation From Magnetic Resonance Images(MRI) Of Human Head Scan Using Richardson Lucy Deconvolution And Intensity Thresholding”, 978-1-5090-4420-7/16/$31.00 ©2016 IEEE
[20] Y. Xiao, T. Zeng, J. Yu, and M. K. Ng, “Restoration of images corrupted by mixed gaussian-impulse noise via l1-l0 minimization” Pattern Recognition, vol. 44, no. 8, pp. 1708 – 1720, 2011.
[21] Z. Ningbo, W. Gang, Y. Gaobo, and D. Weiming, “A fast 2d otsu thresholding algorithm based on improved histogram” in Pattern Recognition, 2009,CCPR 2009, Chinese Conference on, pp. 1–5, 2009.
[22] Rosita Shishegar, Anand A. Joshi, Mary Tolcos, David W. Walker, Leigh A. Johnston, “Automatic segmentation of fetal brain using diffusion-weighted imaging cues”, 978-1-5090-1172-8/17/$31.00 ©2017 IEEE.
[23] J Tournier, Fernando Calamante, Alan Connelly, et al.,“MRtrix: diffusion tractography in crossing fiber regions”,International Journal of Imaging Systems andTechnology, vol. 22, no. 1, pp. 53–66, 2012.
[24] Jose Dolz, Ismail Ben Ayed, Jing Yuany Christian Desrosiers, “Isointense infant brain segmentation with a hyper-dense connectedConvolutional neural network”.