Performance Prediction Model for National Level Examinations
P. Shanmugavadivu1 , P. Haritha2 , Ashish Kumar3
Section:Research Paper, Product Type: Journal Paper
Volume-06 ,
Issue-04 , Page no. 292-297, May-2018
Online published on May 31, 2018
Copyright © P. Shanmugavadivu, P. Haritha, Ashish Kumar . This is an open access article distributed under the Creative Commons Attribution License, which permits unrestricted use, distribution, and reproduction in any medium, provided the original work is properly cited.
View this paper at Google Scholar | DPI Digital Library
How to Cite this Paper
- IEEE Citation
- MLA Citation
- APA Citation
- BibTex Citation
- RIS Citation
IEEE Style Citation: P. Shanmugavadivu, P. Haritha, Ashish Kumar, “Performance Prediction Model for National Level Examinations,” International Journal of Computer Sciences and Engineering, Vol.06, Issue.04, pp.292-297, 2018.
MLA Style Citation: P. Shanmugavadivu, P. Haritha, Ashish Kumar "Performance Prediction Model for National Level Examinations." International Journal of Computer Sciences and Engineering 06.04 (2018): 292-297.
APA Style Citation: P. Shanmugavadivu, P. Haritha, Ashish Kumar, (2018). Performance Prediction Model for National Level Examinations. International Journal of Computer Sciences and Engineering, 06(04), 292-297.
BibTex Style Citation:
@article{Shanmugavadivu_2018,
author = { P. Shanmugavadivu, P. Haritha, Ashish Kumar},
title = {Performance Prediction Model for National Level Examinations},
journal = {International Journal of Computer Sciences and Engineering},
issue_date = {5 2018},
volume = {06},
Issue = {04},
month = {5},
year = {2018},
issn = {2347-2693},
pages = {292-297},
url = {https://www.ijcseonline.org/full_spl_paper_view.php?paper_id=399},
publisher = {IJCSE, Indore, INDIA},
}
RIS Style Citation:
TY - JOUR
UR - https://www.ijcseonline.org/full_spl_paper_view.php?paper_id=399
TI - Performance Prediction Model for National Level Examinations
T2 - International Journal of Computer Sciences and Engineering
AU - P. Shanmugavadivu, P. Haritha, Ashish Kumar
PY - 2018
DA - 2018/05/31
PB - IJCSE, Indore, INDIA
SP - 292-297
IS - 04
VL - 06
SN - 2347-2693
ER -
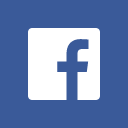
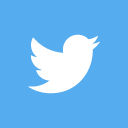
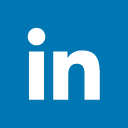
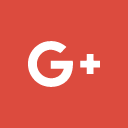
Abstract
In the recent years, usage of data mining techniques to statistically analyze the performance of candidates in academics or national level examinations is in increase. The development of predictive analytics tools and their applications are also in the rise. This paper reports on the mechanism of the proposed prediction model that predicts the performance of a candidate appearing for national level examinations. The proposed Performance Prediction Model (PPM) is designed as a framework comprising of data classification and ranking of dataset, computation of correlation coefficient that measures the dependency among the variables and prediction using linear regression. The performance of PPM is validated on UGC-NET (2016) dataset. Based on the observed correlation between Paper-II and Paper-III marks, PPM predicts the score of a candidate in Paper-III with reference to the scored marks in Paper-II. The accuracy of the predicted data is recorded as 88 per cent. The illustrative visualizations presented in this article depict the performance analysisof the candidates in Paper-I, Paper-II and Paper-III.
Key-Words / Index Term
Performance Prediction Model (PPM), Classification, Ranking, Correlation Coefficient, Linear Regression Model.
References
[1] N. Bhargava, G. Sharma, R. Bhargava, M. Mathuria, “Decision Tree Analysis on J48 Algorithm for Data Mining”. Journal of Advanced Research in Computer Science and Software Engineering, Vol.3, Issue.6, pp. 1114, 1115 June 2013.
[2] M. Gupta, N. Agarwal, “Classification Techniques Analysis”, National Conference on Computational Instrumentation, pp. 128, 129 March, 2010.
[3] S.B. Kotsiantis, “Supervised Machine Learning: A Review of Classification Techniques”, Department of Computer Science and Technology, University of Peloponnese, Greece, pp.249, July 16, 2007.
[4] G. De’ath, K. E. Fabricius, “Classification and Regression Trees: A Powerful Yet Simple Technique for Ecological Data Analysis”, Ecological Society of America, Vol. 81, No. 11, pp. 3178, 2000.
[5] T.S.Korting, "C4.5 algorithm and Multivariate Decision Trees." Image Processing Division, National Institute for Space Research – INPE SaoJose dos Campos – SP, Brazil.
[6] Y.Y. Song and Y. LU, “Decision tree methods: applications for classification and prediction”, Shanghai Archives of Psychiatry, Vol.27, No.2.
[7] I.Jenhani, N. B. Amor, Z. Elouedi, “Decision Tree as probabilistic classifiers”,In the proceedings of the International Journal of Approximate Reasoning, Vol.48, Issue.3, pp.784, August 2008.
[8] Harwati, A. Sudiya, “Application of Decision tree approach to Student Selection Model- A Case Study”. In the proceedings of the IOP Conference Series: Materials Science and Engineering, Vol.105, Conference.1, pp.1, 2016.
[9] V. Bewick, L. Cheek and J. Ball. “Statistics review 7: Correlation and Regression”, Published in Critical care, pp.451, 2003.
[10] W. Medhat, A. Hassan, H. Korashy, “Sentiment analysis algorithm and applications: A survey”, In the proceedings of Ain Shams Engineering Journal, Vol.5, Issue.4, pp.1093-1113, December 2014.
[11] F. M. B. N. Hanif, P. Saptawati, “Correlation analysis of user influence and sentiment on Twitter data” In the proceedings of the International Conference Data and Software Engineering (ICODSE), Bandung, Indonesia, 2014.
[12] V. A. Kharde, S.S. Sonawane, “Sentiment Analysis of Twitter Data: A Survey Techniques”, International Journal of Computer Applications, Vol.139, No.11, pp.5, April 2016.
[13] H.E. Brogden, “On the interpretation of the correlation coefficient as a measure of predictive efficiency”. In the proceedings of the Journal of Educational Psychology, 1946.
[14] G. Sudhamathy, “Credit Risk Analysis and Prediction Modelling of Bank Loans Using R” International Journal of Engineering and Technology, Vol. 8, No.5, pp.1954, 2016.
[15] H. Hamsa, S. Indiradevi, J. J. Kizhakkethottam, “Student academic performance prediction model using decision tree and fuzzy genetic algorithm”, In the proceedings of the Procedia Technology, Vol.25, pp.326, 2016.
[16] E. Seidal, S. Kutieleh, “Using predictive analytics to target and improve first year student attrition”, In the proceedings of Australian Journal of Education, Vol. 61, Issue.2, pp. 200, August 2017.
[17] A. S. Issac, “Statistics”, SCITech Publications,Chapter 6, 2011.