An Analysis on Methods to Predict On Demand Based Online Agricultural Commodity of Selling Buying Using Social Computational Driven Models
Nithya Ganapathi Subramanian1 , P.S.S. Akhilashri2
Section:Survey Paper, Product Type: Journal Paper
Volume-06 ,
Issue-11 , Page no. 206-209, Dec-2018
Online published on Dec 31, 2018
Copyright © Nithya Ganapathi Subramanian, P.S.S. Akhilashri . This is an open access article distributed under the Creative Commons Attribution License, which permits unrestricted use, distribution, and reproduction in any medium, provided the original work is properly cited.
View this paper at Google Scholar | DPI Digital Library
How to Cite this Paper
- IEEE Citation
- MLA Citation
- APA Citation
- BibTex Citation
- RIS Citation
IEEE Citation
IEEE Style Citation: Nithya Ganapathi Subramanian, P.S.S. Akhilashri, “An Analysis on Methods to Predict On Demand Based Online Agricultural Commodity of Selling Buying Using Social Computational Driven Models,” International Journal of Computer Sciences and Engineering, Vol.06, Issue.11, pp.206-209, 2018.
MLA Citation
MLA Style Citation: Nithya Ganapathi Subramanian, P.S.S. Akhilashri "An Analysis on Methods to Predict On Demand Based Online Agricultural Commodity of Selling Buying Using Social Computational Driven Models." International Journal of Computer Sciences and Engineering 06.11 (2018): 206-209.
APA Citation
APA Style Citation: Nithya Ganapathi Subramanian, P.S.S. Akhilashri, (2018). An Analysis on Methods to Predict On Demand Based Online Agricultural Commodity of Selling Buying Using Social Computational Driven Models. International Journal of Computer Sciences and Engineering, 06(11), 206-209.
BibTex Citation
BibTex Style Citation:
@article{Subramanian_2018,
author = {Nithya Ganapathi Subramanian, P.S.S. Akhilashri},
title = {An Analysis on Methods to Predict On Demand Based Online Agricultural Commodity of Selling Buying Using Social Computational Driven Models},
journal = {International Journal of Computer Sciences and Engineering},
issue_date = {12 2018},
volume = {06},
Issue = {11},
month = {12},
year = {2018},
issn = {2347-2693},
pages = {206-209},
url = {https://www.ijcseonline.org/full_spl_paper_view.php?paper_id=572},
publisher = {IJCSE, Indore, INDIA},
}
RIS Citation
RIS Style Citation:
TY - JOUR
UR - https://www.ijcseonline.org/full_spl_paper_view.php?paper_id=572
TI - An Analysis on Methods to Predict On Demand Based Online Agricultural Commodity of Selling Buying Using Social Computational Driven Models
T2 - International Journal of Computer Sciences and Engineering
AU - Nithya Ganapathi Subramanian, P.S.S. Akhilashri
PY - 2018
DA - 2018/12/31
PB - IJCSE, Indore, INDIA
SP - 206-209
IS - 11
VL - 06
SN - 2347-2693
ER -
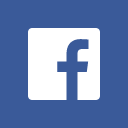
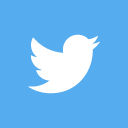
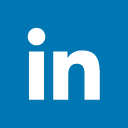
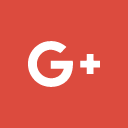
Abstract
Consumption and demand for agricultural produce is always on high variable ends. Consumption of an agricultural produce increases the demand among consumers and also vice versa. The need for a system to predict on demand for commodity is always felt among farming commodity such that the demand for agricultural commodity can be predicted earlier and hence supported earlier. This research works on application of social computing models over understanding the trends of consumer for prediction of demand. This research works on base of online marketing along with commodity utilization demand is discussed. Subsequently, ways to improve online marketing using the concepts of social computing are proposed and implemented. Analysis predicts on application of multiple soft computational models employed over traditional online marketing methods to suggest of effective / accurate prediction.
Key-Words / Index Term
Social Computing, Agricultural Commodity, Agricultural Demand / Supply
References
[1] Arunkumar Thangavelu, N Manoharan, Design and Analysis of an Effective Channel Distribution Approach for Agricultural Commodities using MongoDB, Indian Journal of Science and Technology, Volume 9, Issue 47, 2018
[2] Chandra, R., Iyer, R. S., & Raman, R. (2015). “Enabling organizations to implement smarter, customized social computing platforms by leveraging knowledge flow patterns” In Journal of Knowledge Management, 19(1), 95-107
[3] Evans, N.D. (2010), “Application modernization and outsourcing-the adoption of social computing in the enterprise” In UNISYS, Tech Republic
[4] Chandra, R., Iyer, R.S. and Raman, R. (2013), “Social computing platforms leveraging knowledge flow patterns” In ICKM conference proceedings, pp. 29-39.
[5] W. Mason, J. W. Vaughan, and H. Wallach. (2013). “Computational social science and social computing” In Journal of Machine Learning, pages 1–4.
[6] Fei-Yue Wang, Xiaochen Li, Wenji Mao, Tao Wang. (2012). “Social Computing: Methods and Applications” In Zhejiang University Press, Hangzhou
[7] X Wang, L Li, Y Yuan, P Ye, FY Wang. (2016). “ACP based social computing and parallel intelligence” In CAAI Transactions on Intelligence Technology pp 377-393
[8] Steven J. Jackson, Tarleton Gillespie, and Sandy Payette. (2014). “The policy knot: Re-integrating policy, practice and design in CSCW studies of social computing” In Proceedings of the 17th ACM conference on Computer supported cooperative work & social computing (CSCW `14). ACM, New York, NY, USA, pp. 588–602.
[9] Gunasundari Anantharaj, Arunkumar Thangavelu, A Predictive analytical approach towards improving the crop growth yield using fuzzy cognitive maps – CROYAN, IIOAB, Vol. 6,No-4, pgs: 120–130, 2015
[10]Shilton K., Koepfler Jes A., Fleischmann Kenneth R. (2014). “How to see values in social computing: methods for studying values dimensions” In Proceedings of the 17th ACM conference on Computer supported cooperative work & social computing, February 15-19 2014, Baltimore, Maryland, USA
[11] Koch M, Schwabe G, Briggs R O. (2015). “CSCW and Social Computing” In Business and Information Systems Engineering Vol 57 Issue 3 pp 149-153
[12] Jin R., Zhang H., Zhang Y. (2018). “The Uncertainty problem in Social Computing and its Solution Method” In 2018 International Conference on Robots and Intelligent Systems (ICRIS) pp 517-521
[13] Sakthikumar Subramanian, Arunkumar Thangavelu, FACT-An adaptive customer churn rate prediction method using fuzzy muti-criteria classification approach for decision making, Asian Journal of Science and Technology, Volume-4, Issue-11, pg: 227-233, 2011
[14] Tang M., Zhu H., Mao X. (2015). “A Lightweight social computing approach to emergency management policy selection” In IEEE Transactions on Systems, Man and Cybernetics 2016 Vol 46 Issue 8
[15] Yu R., Prakash S. (2017). “Optimized online marketing and scheduling systems and methods that are based on demand driven results” In US Patent US9741021B2
[16] Padmanabhan N. (2018). “Online Marketing- Present Scenario” In International Journal of Scientific Research Vol 7 No 4(2018)
[17] Susanne Schwarzl and Monika Grabowska. (2015), “Online Marketing Strategies: the future is here” In Journal of international studies, Volume :8, No.2, pp.187 – 196.
[18] Wu, J., N. Wen, W. Dou, and J. Chen. (2015). “Exploring the Effectiveness of Consumer Creativity in Online Marketing Communications” In European Journal of Marketing 49 (1/2): 262–276.
[19] G Roy, B Datta, R Basu. (2017). “Trends and future directions in online marketing research” In Journal of Internet Commerce Vol 16 Issue 1
[20] Bowie D., Paraskevas A., Mariussen A. (2014). “Technology driven online marketing- Lessons from Affiliate Marketing” International Journal of Online Marketing 4(4)
[21] Stoliartchouk A., Jordan F., Box B. (2017). “System and method for dynamic management of affiliate links for online marketing” US Patent US9779425B2
[22] Ting T., Davis J., Pettit F. (2014). “Online marketing research utilizing sentiment analysis and tunable demographics analysis” US Patent US8694357B2
[23] Mitran A., Negricea C., Edu T. (2014). “Modelling the Influence of Online Marketing Communication on Behavioural Intentions”, Network Intelligence Studies Volume II, 2 (4), 245-253.
[24] Gurau C. (2008). “Integrated online marketing communication: implementation and management” Journal of communication management Vol 12 Issue 2