A Comparative Study on Weka, Orange Tool for Mushroom Data Set
M. Senthamilselvi1 , P.S.S. Akilashri2
Section:Survey Paper, Product Type: Journal Paper
Volume-06 ,
Issue-11 , Page no. 231-236, Dec-2018
Online published on Dec 31, 2018
Copyright © M. Senthamilselvi, P.S.S. Akilashri . This is an open access article distributed under the Creative Commons Attribution License, which permits unrestricted use, distribution, and reproduction in any medium, provided the original work is properly cited.
View this paper at Google Scholar | DPI Digital Library
How to Cite this Paper
- IEEE Citation
- MLA Citation
- APA Citation
- BibTex Citation
- RIS Citation
IEEE Style Citation: M. Senthamilselvi, P.S.S. Akilashri, “A Comparative Study on Weka, Orange Tool for Mushroom Data Set,” International Journal of Computer Sciences and Engineering, Vol.06, Issue.11, pp.231-236, 2018.
MLA Style Citation: M. Senthamilselvi, P.S.S. Akilashri "A Comparative Study on Weka, Orange Tool for Mushroom Data Set." International Journal of Computer Sciences and Engineering 06.11 (2018): 231-236.
APA Style Citation: M. Senthamilselvi, P.S.S. Akilashri, (2018). A Comparative Study on Weka, Orange Tool for Mushroom Data Set. International Journal of Computer Sciences and Engineering, 06(11), 231-236.
BibTex Style Citation:
@article{Senthamilselvi_2018,
author = {M. Senthamilselvi, P.S.S. Akilashri},
title = {A Comparative Study on Weka, Orange Tool for Mushroom Data Set},
journal = {International Journal of Computer Sciences and Engineering},
issue_date = {12 2018},
volume = {06},
Issue = {11},
month = {12},
year = {2018},
issn = {2347-2693},
pages = {231-236},
url = {https://www.ijcseonline.org/full_spl_paper_view.php?paper_id=577},
publisher = {IJCSE, Indore, INDIA},
}
RIS Style Citation:
TY - JOUR
UR - https://www.ijcseonline.org/full_spl_paper_view.php?paper_id=577
TI - A Comparative Study on Weka, Orange Tool for Mushroom Data Set
T2 - International Journal of Computer Sciences and Engineering
AU - M. Senthamilselvi, P.S.S. Akilashri
PY - 2018
DA - 2018/12/31
PB - IJCSE, Indore, INDIA
SP - 231-236
IS - 11
VL - 06
SN - 2347-2693
ER -
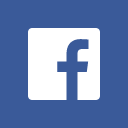
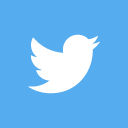
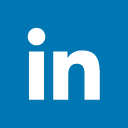
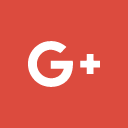
Abstract
Association rule mining (ARM) is used to advance decisions making in the application. ARM became important in an information and decision-overloaded world. They changed the way user make decisions, and helped their creators to increase revenue at the same time. Bringing ARM is essential in order to popularize them beyond the limits of scientific research and high technology entrepreneurship. This paper extracts attractive correlations frequent patterns and association among set of items in the transaction database. This paper describes the show analysis of Naïve Bayes and J48 classification algorithm based on the correct and incorrect instances of data classification. Naive Bayes is probability based and j48 algorithm is decision tree based. In this paper Comparison weka and orange by using tool to perform evaluation of classifiers NAIVE BAYES and J48 in the context of mushroom dataset in UCI repository to maximize true positive rate and minimize false positive rate of defaulters rather than achieving only higher classification accuracy using WEKA and orange tool. The experiments results reveals the true positive rate, false positive rate, classification accuracy and cost analysis. The results in the paper on mushroom dataset in UCI repository performance best in Weka than Orange tools. The efficiency and accuracy of J48 -than Naive Bayes is good
Key-Words / Index Term
Data mining, Weka Tool, Orange tool J48,Navie Bayes
References
[1] Huaifeng Zhang, Yanchang Zhao, Longbing Cao and Chengqi Zhang, “Combined Association Rule Mining”, PAKDD 2008, LNAI 5012, pp. 1069-1074, 2008 © Springer- Verlag Berlin Heidelberg 2008
[2] Pratima Gautam and K.R. Pardasani, “Algorithm for Efficient Multilevel Association Rule Mining” In (IJCSE) International Journal on Computer Science and Engineering, Volume 02, No. 05, 1700-1704, 2010.
[3] Xunwei Zhou and Hong Bao ,” Mning Double-Connective Association Rules from Multiple Tables of Relational Databases “ In IEEE,2008
[4] Raja Tlili and Yahya Slimani, “Executing Association Rule Mining Algorithm under a Gird Computing Environment” In PADTAD,July 2011.
[5] Anis Suhailis Abdul Kadir, Azuraliza Abu Bakar and Abdul Razak Hamdan, “Frequent Absence and Presence Itemset for Negative Association Rule Mining “, IEEE,2011.
[6] Guimei Liu, Haojun Zhang and Limsoon Wong, “Controlling False Positives Iin Association Rule Mining” In Proceedings of the VLDB Endowment ACM,2011.
[7] Somboon Anekritmongkol and M. L. Kulthon Kasamsan , “ The Comparative of Boolean Algebra Compress and Apriori Rule Techniques for New Theoretic Association Rule Mining Model” In IEEE,2009
[8] Salama, G.I.; Abdelhalim, M.B.; Zeid, M.A., "Experimental comparison of classifiers for breast cancer diagnosis," Computer Engineering & Systems (ICCES), 2012 Seventh International Conference on , vol., no., pp.180,185, 27-29 Nov.,2012.
[9] S. Moertini Veronica ,”Towards The Use Of C4.5 Algorithm For Classifying Banking Dataset”,Integeral Vol 8 No 2,October 2013.
[10] UM, Ashwinkumar, and Anandakumar KR. "Predicting Early Detection of Cardiac and Diabetes Symptoms using Data Mining Techniques.",IEEE,pp:161-165,2011
[11] Geetha Ramani R, Lakshmi Balasubramanian, and Shomona Gracia Jacob. "Automatic prediction of Diabetic Retinopathy and Glaucoma through retinal image analysis and data mining techniques." In Machine Vision and Image Processing (MVIP), 2012 International Conference on, pp. 149-152. IEEE, 2012
[12] Sugimoto, Masahiro, Masahiro Takada and Masakazu Toi. "Comparison of robustness against missing values of alternative decision tree and multiple logistic regression for predicting clinical data in primary breast cancer." In Engineering in Medicine and Biology Society (EMBC), 2013 35th Annual International Conference of the IEEE, pp. 3054-3057. IEEE, 2013.
[13] Hussein Asmaa S,Wail M. Omar, Xue Li, and Modafar Ati. "Efficient Chronic Disease Diagnosis prediction and recommendation system." In Biomedical Engineering and Sciences (IECBES), 2012 IEEE EMBS Conference on, pp. 209-214. IEEE, 2012.
[14] Bache, K. & Lichman, M. (2013). UCI Machine Learning Repository [http://archive.ics.uci.edu/ml]. Irvine, CA: University of California, School of Information and Computer Science.
[15] Korting, Thales Sehn. "C4. 5 algorithm and Multivariate Decision Trees." Image Processing Division, National Institute for Space Research--INPE.
[16] Guo, Yang, Guohua Bai, and Yan Hu. "Using Bayes Network for Prediction of Type-2 Diabetes." In Internet Technology And Secured Transactions, 2012 International Conferece For, pp. 471-472. IEEE, 2012.