Thyroid Disease Prediction by Machine Learning Technique From Healthcare Communities
M.Shyamala 1 , P.S.S. Akilashri2
Section:Survey Paper, Product Type: Journal Paper
Volume-06 ,
Issue-11 , Page no. 237-242, Dec-2018
Online published on Dec 31, 2018
Copyright © M.Shyamala, P.S.S. Akilashri . This is an open access article distributed under the Creative Commons Attribution License, which permits unrestricted use, distribution, and reproduction in any medium, provided the original work is properly cited.
View this paper at Google Scholar | DPI Digital Library
How to Cite this Paper
- IEEE Citation
- MLA Citation
- APA Citation
- BibTex Citation
- RIS Citation
IEEE Style Citation: M.Shyamala, P.S.S. Akilashri, “Thyroid Disease Prediction by Machine Learning Technique From Healthcare Communities,” International Journal of Computer Sciences and Engineering, Vol.06, Issue.11, pp.237-242, 2018.
MLA Style Citation: M.Shyamala, P.S.S. Akilashri "Thyroid Disease Prediction by Machine Learning Technique From Healthcare Communities." International Journal of Computer Sciences and Engineering 06.11 (2018): 237-242.
APA Style Citation: M.Shyamala, P.S.S. Akilashri, (2018). Thyroid Disease Prediction by Machine Learning Technique From Healthcare Communities. International Journal of Computer Sciences and Engineering, 06(11), 237-242.
BibTex Style Citation:
@article{Akilashri_2018,
author = {M.Shyamala, P.S.S. Akilashri},
title = {Thyroid Disease Prediction by Machine Learning Technique From Healthcare Communities},
journal = {International Journal of Computer Sciences and Engineering},
issue_date = {12 2018},
volume = {06},
Issue = {11},
month = {12},
year = {2018},
issn = {2347-2693},
pages = {237-242},
url = {https://www.ijcseonline.org/full_spl_paper_view.php?paper_id=578},
publisher = {IJCSE, Indore, INDIA},
}
RIS Style Citation:
TY - JOUR
UR - https://www.ijcseonline.org/full_spl_paper_view.php?paper_id=578
TI - Thyroid Disease Prediction by Machine Learning Technique From Healthcare Communities
T2 - International Journal of Computer Sciences and Engineering
AU - M.Shyamala, P.S.S. Akilashri
PY - 2018
DA - 2018/12/31
PB - IJCSE, Indore, INDIA
SP - 237-242
IS - 11
VL - 06
SN - 2347-2693
ER -
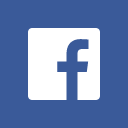
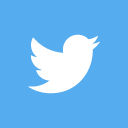
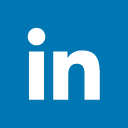
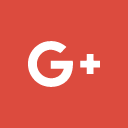
Abstract
Because of the huge information in biomedical and healthcare communities, correct study of medical data benefits early disease detection, community services and patient care. The exactness of study is reduced when the value of medical data is incomplete. Moreover, various regions exhibit unique appearances of particular regional diseases, those results in weakening the prediction of disease outbreaks. In the proposed system, it provides machine learning algorithms for effective prediction of thyroid disease occurrences in disease-frequent societies. It experiment the changed models over real-life hospital data collected. To overcome the difficulty of incomplete data, it uses a latent factor model to rebuild the missing data. It experiment on a thyroid diseases using structured and unstructured data from hospital it use FR-Growth and Decision Tree algorithm. Compared to several typical estimate algorithms, the calculation exactness of our proposed FP Growth algorithm reaches 98.8% with a convergence speed which is faster than that of the decision tree algorithm on disease risk prediction on thyroid using Weka tool.
Key-Words / Index Term
Data mining, Machine Learning, Decision Tree
References
[1] P. B. Jensen, L. J. Jensen, and S. Brunak,“Mining electronic health records: towards better research applications and clinical care.
[2] hahab Tayeb*, Matin Pirouz*, Johann Sun1, Kaylee Hall1, Andrew Chang1, Jessica Li1, Connor Song1, Apoorva Chauhan2, Michael Ferra3, Theresa Sager3, Justin Zhan*, Shahram Latifi, Toward Predicting Med-ical Conditions Using k-Nearest Neighbours, 2017 IEEE International Conference on Big Data.
[3] reekanth Rallapalli Faculty of computing Botho University Gaborone, Botswana redicting the Risk of Diabetes in Big Data Electronic Health Records by using Scalable Random Forest Classification Algorithm, 2016 IEEE.
[4] oubida Alaoui Mdaghri, Mourad El Yadari, Abdelillah Benyoussef, Ab-dellah El Kenz Faculty of Science Rabat Morocco, Rabat, Study and analysis of Data Mining for Healthcare, 2016 IEEE.
[5] hen-Ying Hung, Wei-Chen Chen, Po-Tsun Lai, Ching-Heng Lin, and Chi-Chun Lee, Comparing Deep Neural Network and Other Machine Learning Algorithms for Stroke Prediction in a Large-Scale Population-Based Electronic Medical Claims Database, 2017 IEEE.
[6] rof. Dhomse Kanchan B. Assistant Professor of IT department METS BKC IOE, Nasik Nasik, India kdhomse@gmail.com , Mr. Mahale Kishor M. Technical Assistant of IT department METS BKC IOE, Nasik, India kishu2006.kishor@gmail.com, Study of Machine Learning Algorithms for Special Disease Prediction using Principal of Component Analy-sis,2016 IEEE.
[7] P.-N. Tan, M. Steinbach, and V. Kumar, “Introduction to Data Mining,” Boston, U.S.A.: Pearson Education Inc., 2006, ch. 4, pp. 151-154.
[8] R. Polikar, “Ensemble Based Systems in Decision Making,” IEEE Circuits and Systems Magazine, vol. 6, pp. 21-45, Third Quarter, 2006.
[9] T. K. Ho, “The Random Subspace Method for Constructing Decision Forests,” IEEE Transaction on Pattern Analysis and Machine Intelligence, vol. 20, no.1, pp 832-844, August 1998
[10] Hossam M. Zawbaa, Maryam Hazman, Mona Abbass, Aboul Ella Hassanien, “Automatic fruit classification using random forest algorithm”, 14th International Conference on Hybrid Intelligent Systems,pp. 164 – 168, 2014.
[11] Jiawei Hanl, Yanheng Liu, Xin Sun, “A Scalable Random Forest Algorithm Based on MapReduce”, IEEE 4th International Conference on Software Engineering and Service Science, pp. 849 – 852, 2013.
[12] B.V.S Dheeraj Reddy, Mounika Booreddy, “Classification And Clustering Medical Datasets By Using Artificial Neural Network Models”, Publications Of Problems & Application In Engineering Research – Paper, Vol 04, Special Issue 01, 2013.
[13] Dr. G. Rasitha Banu, M.Baviya, “Predicting Thyroid Disease Using Datamining Technique”, International Journal of Modern Trends in Engineering and Research, 2014.
[14] S. Anto, Dr.S.Chandramathi, “Supervised Machine Learning Approaches for Medical Data Set Classifcation - A Review”, InternatIonal Journal of Computer Science & Technology, Vol. 2, Issue 4, Oct. - Dec. 2011
[15] Sudesh Kumar, Nancy, “Efficient K-Mean Clustering Algorithm for Large Datasets using Data Mining Standard Score Normalization”, International Journal on Recent and Innovation Trends in Computing and Communication, Volume: 2 Issue: 10, 2014.
[16] K.Saravana Kumar, Dr. R. Manicka Chezian, “Support Vector Machine And K- Nearest Neighbor Based Analysis For The Prediction Of Hypothyroid”, International Journal of Pharma and Bio Sciences, pp. 447 – 453, 2014.
[17] Noor Azah Samsudin ; Aida Mustapha ; Mohd Helmy Abd Wahab, “Ensemble classification of cyber space users tendency in blog writing using random forest”, Innovations in Information Technology (IIT), 2016 12th International Conference on 28-30 Nov. 2016