Classification of Negation in Sentiment Analysis using Twitter Data
A. Dian1 , D. Saha2
Section:Research Paper, Product Type: Journal Paper
Volume-07 ,
Issue-01 , Page no. 94-99, Jan-2019
Online published on Jan 20, 2019
Copyright © A. Dian, D. Saha . This is an open access article distributed under the Creative Commons Attribution License, which permits unrestricted use, distribution, and reproduction in any medium, provided the original work is properly cited.
View this paper at Google Scholar | DPI Digital Library
How to Cite this Paper
- IEEE Citation
- MLA Citation
- APA Citation
- BibTex Citation
- RIS Citation
IEEE Citation
IEEE Style Citation: A. Dian, D. Saha, “Classification of Negation in Sentiment Analysis using Twitter Data,” International Journal of Computer Sciences and Engineering, Vol.07, Issue.01, pp.94-99, 2019.
MLA Citation
MLA Style Citation: A. Dian, D. Saha "Classification of Negation in Sentiment Analysis using Twitter Data." International Journal of Computer Sciences and Engineering 07.01 (2019): 94-99.
APA Citation
APA Style Citation: A. Dian, D. Saha, (2019). Classification of Negation in Sentiment Analysis using Twitter Data. International Journal of Computer Sciences and Engineering, 07(01), 94-99.
BibTex Citation
BibTex Style Citation:
@article{Dian_2019,
author = {A. Dian, D. Saha},
title = {Classification of Negation in Sentiment Analysis using Twitter Data},
journal = {International Journal of Computer Sciences and Engineering},
issue_date = {1 2019},
volume = {07},
Issue = {01},
month = {1},
year = {2019},
issn = {2347-2693},
pages = {94-99},
url = {https://www.ijcseonline.org/full_spl_paper_view.php?paper_id=600},
publisher = {IJCSE, Indore, INDIA},
}
RIS Citation
RIS Style Citation:
TY - JOUR
UR - https://www.ijcseonline.org/full_spl_paper_view.php?paper_id=600
TI - Classification of Negation in Sentiment Analysis using Twitter Data
T2 - International Journal of Computer Sciences and Engineering
AU - A. Dian, D. Saha
PY - 2019
DA - 2019/01/20
PB - IJCSE, Indore, INDIA
SP - 94-99
IS - 01
VL - 07
SN - 2347-2693
ER -
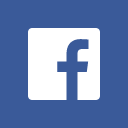
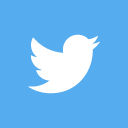
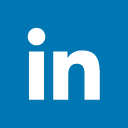
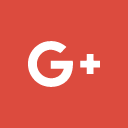
Abstract
This paper provides a brief overview of a paradigm that has been used to identify, classify and cluster the negations consist in the Tweets. Usually unambiguous short text messages, collected from the famous microblogging service Twitter, are called Tweets. It has a maximum character limit of 280 characters. People usually express their standpoints or perspectives about a situation or fact through Tweets. In this collected dataset of Tweets, some negations may be overlapped or/and misclassified. So, our objective is to improve the accuracy using fine classification and increase the sharpness by reducing the overlap or/and misclassification. Here, we have used two different techniques of Sentiment Analysis, such as Lexicon Based Approach and Supervised Learning Approach to train our model. This proposed system has also analyzed Tweets and Emoticons into three categories- Positive, Negative and Neutral. In this analysis, we have used a data set of 2000 Tweets and found 88.14 percentage of accuracy.
Key-Words / Index Term
sentiment, negation, polarity, emoticons
References
[1] L. Jia, C. Yu, W. Meng, “The effect of negation on sentiment analysis and retrieval Effectiveness”, In the Proceeding of the 18th ACM conference (CIKM `09), Hong Kong, China, pp.1827-1830, 2009.
[2] A. Hogenboom, P. V. Iterson, B. Heerschop, F. Frasincar, U. Kaymak, “Determining negation scope and strength in sentiment analysis”, In the Proceedings of 2011 IEEE International Conference on Systems, Man, and Cybernetics, Alaska, USA, pp. 2589-2594, 2011.
[3] B. Pang, L. Lee, S. Vaithyanathan, “Thumbs Up?Sentiment Classification Using Machine Learning Techniques”, In the Proceedings of the 7th conference on Empirical methods in Natural Language Processing (EMNLP `02), Philadelphia, USA, pp.79-86, 2002.
[4] R. Amalia, M. A. Bijaksana, D. Darmantoro, “Negation handling in sentiment classification using rule-based adapted from Indonesian language syntactic for Indonesian text in Twitter”, Journal of Physics: Conference Series, Vol.971, Issue.1, 2018.
[5] F. Zwarts, “Negatief polaire uitdrukkingen I”, Journal of GLOT, Vol.4, pp.35-132, 2002.
[6] T. Givón, “English grammar: A function-based introduction”, John Benjamins Publishing Company, Amsterdam, Netherlands, pp.1-318, 1993.
[7] M. Wiegand, A. Balahur, B. Roth, D. Klakow and A. Montoyo, “A Survey on the Role of Negation in Sentiment Analysis”, In the Proceedings of the Workshop on Negation and Speculation in Natural Language Processing, Uppsala, Sweden, pp.60-68, 2010.
[8] O. Bojar, Franky, K. Veselovská, “Resources for Indonesian Sentiment Analysis”, Journal of The Prague Bulletin of Mathematical Linguistics, Vol.103, Issue.1, pp.21-41, 2015.
[9] S. R. Das, M. Y. Chen, “Yahoo! for Amazon: Sentiment Extraction from Small Talk on the Web”, Journal of Management Science, INFORMS, pp.1375-1388, Vol.53, No.9, 2007.
[10] H. Wang, A. J. Castanon, “Sentiment analysis via emoticons using twitter data”, In the Proceedings of 2015 IEEE International Conference on Big Data, Santa Clara, CA, USA, pp.2404-2408, 2015.
[11] M. Dadvar, C. Hauff, F. D. Jong, “Scope of Negation Detection in Sentiment Analysis”, In the Proceedings of the 11th Dutch-Belgian Information Retrieval Workshop (DIR 2011), Amsterdam, Netherlands, pp.16-20, 2011.
[12] U. Farooq, H. Mansoor, A. Nongaillard, Y. Ouzrout, M. A. Qadir, “Negation Handling in Sentiment Analysis at Sentence Level”, Journal of Computers, Vol.12, Issue.5, pp.470-478, 2017.