Analysis of Intrusion Detection System in Data mining
P. Mangaiyakarasi1
Section:Research Paper, Product Type: Journal Paper
Volume-07 ,
Issue-02 , Page no. 121-125, Jan-2019
Online published on Jan 31, 2019
Copyright © P. Mangaiyakarasi . This is an open access article distributed under the Creative Commons Attribution License, which permits unrestricted use, distribution, and reproduction in any medium, provided the original work is properly cited.
View this paper at Google Scholar | DPI Digital Library
How to Cite this Paper
- IEEE Citation
- MLA Citation
- APA Citation
- BibTex Citation
- RIS Citation
IEEE Style Citation: P. Mangaiyakarasi, “Analysis of Intrusion Detection System in Data mining,” International Journal of Computer Sciences and Engineering, Vol.07, Issue.02, pp.121-125, 2019.
MLA Style Citation: P. Mangaiyakarasi "Analysis of Intrusion Detection System in Data mining." International Journal of Computer Sciences and Engineering 07.02 (2019): 121-125.
APA Style Citation: P. Mangaiyakarasi, (2019). Analysis of Intrusion Detection System in Data mining. International Journal of Computer Sciences and Engineering, 07(02), 121-125.
BibTex Style Citation:
@article{Mangaiyakarasi_2019,
author = {P. Mangaiyakarasi},
title = {Analysis of Intrusion Detection System in Data mining},
journal = {International Journal of Computer Sciences and Engineering},
issue_date = {1 2019},
volume = {07},
Issue = {02},
month = {1},
year = {2019},
issn = {2347-2693},
pages = {121-125},
url = {https://www.ijcseonline.org/full_spl_paper_view.php?paper_id=660},
publisher = {IJCSE, Indore, INDIA},
}
RIS Style Citation:
TY - JOUR
UR - https://www.ijcseonline.org/full_spl_paper_view.php?paper_id=660
TI - Analysis of Intrusion Detection System in Data mining
T2 - International Journal of Computer Sciences and Engineering
AU - P. Mangaiyakarasi
PY - 2019
DA - 2019/01/31
PB - IJCSE, Indore, INDIA
SP - 121-125
IS - 02
VL - 07
SN - 2347-2693
ER -
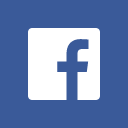
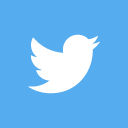
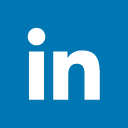
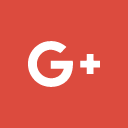
Abstract
It ends up being logically basic to separate interferences with cloud precedents to guarantee our business from digital psychological warfare dangers. This paper presents information digging advances planned therefore; SmartSifter (special case area engine), ChangeFinder, AccessTracer. All of them can learn quantifiable instances of logs adaptively and to perceive interferences as verifiable characteristics concerning the insightful precedents. We rapidly graph the measures of these engines and demonstrate their applications to sort out intrusion distinguishing proof, worm revelation, and impostor acknowledgment.
Key-Words / Index Term
Data mining, Security, IDS
References
[1] W. Lee, S.J. Stolfo, K.W. Mok, “A data mining framework for building intrusion detection models”, in: Proceedings of IEEE Symposium on Security and Privacy, 1999, pp. 120–132.
[2] W. Feng, Q. Zhng, G. Hu, J Xiangji Huang,” Mining network data for intrusion detection through combining SVMs with ant colony networks ”Future Generation Computer Systems,2013.
[3] T. Zhang, R. Ramakrishnan, M. Livny, “BIRCH: an efficient data clustering method for very large databases”, in: Proceedings of SIGMOD, ACM, 1996, pp. 103–114.
[4] L. Khan, M. Awad, B. Thuraisingham, “A new intrusion detection system using support vector machines and hierarchical clustering”, The VLDB Journal 16(2007) 507–521
[5] X. Xu, “Adaptive intrusion detection based on machine learning: feature extraction, classifier construction and sequential pattern prediction”, Information Assurance and Security 4 (2006) 237–246.
[6] J.X. Huang, J. Miao, Ben He, “High performance query expansion using adaptive co –training”, Information Processing & Management 49 (2) (2013) 441–453.
[7] Y. Li u, X. Yu, J.X. Huang, A.” An, Combining integrated sampling with SVM ensembles for learning from imbalanced datasets”, Information Processing &Management 47 (4) (2011) 617–631.
[8] V. Vapnik, “The Nature of Statistical Learning Theory”, Springer, 1999.
[9] Marcelloni, combining “supervised and unsupervised learning for data clustering”, Neural Computing & Applications 15 (3–4) (2006)289–297.
[10] C.-F. Tsai, Y.-F. Hsu, C.-Y. Lin, W.-Y. Lin, “Intrusion detection by machine learning: a review”, Expert Systems with Applications 36 (2009) 11994–12000.
[11] S.X. Wu, W. Banzhaf, “The use of computational intelligence in intrusion detection systems: a review”, Applied Soft Computing 10 (2010) 1–35.