Feature Selection on High Dimensional Big Data of Gens Expression Using Filter Based Feature Selection Methods
A. K. Shrivas1 , Prem Kumar Chandrakar2
Section:Research Paper, Product Type: Journal Paper
Volume-07 ,
Issue-03 , Page no. 105-108, Feb-2019
Online published on Feb 15, 2019
Copyright © A. K. Shrivas, Prem Kumar Chandrakar . This is an open access article distributed under the Creative Commons Attribution License, which permits unrestricted use, distribution, and reproduction in any medium, provided the original work is properly cited.
View this paper at Google Scholar | DPI Digital Library
How to Cite this Paper
- IEEE Citation
- MLA Citation
- APA Citation
- BibTex Citation
- RIS Citation
IEEE Style Citation: A. K. Shrivas, Prem Kumar Chandrakar, “Feature Selection on High Dimensional Big Data of Gens Expression Using Filter Based Feature Selection Methods,” International Journal of Computer Sciences and Engineering, Vol.07, Issue.03, pp.105-108, 2019.
MLA Style Citation: A. K. Shrivas, Prem Kumar Chandrakar "Feature Selection on High Dimensional Big Data of Gens Expression Using Filter Based Feature Selection Methods." International Journal of Computer Sciences and Engineering 07.03 (2019): 105-108.
APA Style Citation: A. K. Shrivas, Prem Kumar Chandrakar, (2019). Feature Selection on High Dimensional Big Data of Gens Expression Using Filter Based Feature Selection Methods. International Journal of Computer Sciences and Engineering, 07(03), 105-108.
BibTex Style Citation:
@article{Shrivas_2019,
author = {A. K. Shrivas, Prem Kumar Chandrakar},
title = {Feature Selection on High Dimensional Big Data of Gens Expression Using Filter Based Feature Selection Methods},
journal = {International Journal of Computer Sciences and Engineering},
issue_date = {2 2019},
volume = {07},
Issue = {03},
month = {2},
year = {2019},
issn = {2347-2693},
pages = {105-108},
url = {https://www.ijcseonline.org/full_spl_paper_view.php?paper_id=687},
publisher = {IJCSE, Indore, INDIA},
}
RIS Style Citation:
TY - JOUR
UR - https://www.ijcseonline.org/full_spl_paper_view.php?paper_id=687
TI - Feature Selection on High Dimensional Big Data of Gens Expression Using Filter Based Feature Selection Methods
T2 - International Journal of Computer Sciences and Engineering
AU - A. K. Shrivas, Prem Kumar Chandrakar
PY - 2019
DA - 2019/02/15
PB - IJCSE, Indore, INDIA
SP - 105-108
IS - 03
VL - 07
SN - 2347-2693
ER -
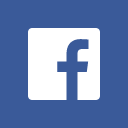
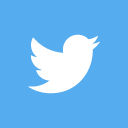
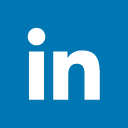
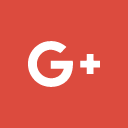
Abstract
Feature selection approach solves the dimensionality problem by removing irrelevant and redundant features. Recently, big data is widely available in information systems and data mining has pulled in a major thoughtfulness regarding analysts to transform such information into helpful learning. This implies the presence of low quality, questionable, excess and uproarious information which contrarily influence the way toward watching learning and helpful example. As follows, researchers require related big data utilizing feature selection methods. The process of feature selection is identifying the most relevant attributes and removing the redundant and irrelevant attributes. In this paper, find out the result of different feature selection methods based on a recognized dataset (i.e., gens expression dataset) and classification algorithms were used to evaluate the performance of the algorithms. In this study revealed that feature selection methods are capable to improve the performance of learning algorithms. Still, there are no any single filter based feature selection method is the best. Taken as a whole, Classifier AttEval, CorrelationAttributeEval, Principal Components, and ReliefAttEval methods performed better results than the others.
Key-Words / Index Term
Feature selection, Lung cancer, Gens expression, Classifier, Subset
References
[1] A. Tsymbal and S. Puuronen. (2010). Local feature selection with dynamic integration of classifiers. Foundations of Intelligent Systems, 363–375.
[2] Ashraf, M., Chetty, G., & Tran, D. (2013). Feature Selection Techniques on Thyroid , Hepatitis , and Breast Cancer Datasets, 3(March), 1–8.
[3] Bhattacharjee, a, Richards, W. G., Staunton, J., Li, C., Monti, S., Vasa, P., … Meyerson, M. (2001). Classification of human lung carcinomas by mRNA expression profiling reveals distinct adenocarcinoma subclasses. Proc Natl Acad Sci U S A, 98(24), 13790–5.
[4] Dimitoglou, G., Adams, J. a, & Jim, C. M. (2012). Comparison of the C4.5 and a Naive Bayes Classifier for the Prediction of Lung Cancer Survivability. Journal of Neural Computing, 4(8), 1–9.
[5] Hall, M. (1999). Correlation-based Feature Selection for Machine Learning. Methodology, 21i195-i20(April), 1–5.
[6] Holte, R. C. (1993). Very Simple Classi fi cation Rules Perform Well on Most Commonly Used Datasets. Machine Learning, 11(1), 63–91.
[7] http://www.cs.waikato.ac.nz/ml/weka. (n.d.). WEKA: Weka 3: Data Mining Software in Java.
[8] Huang, S. H., Wulsin, L. R., Li, H., & Guo, J. (2009). Dimensionality reduction for knowledge discovery in medical claims database: Application to antidepressant medication utilization study. Computer Methods and Programs in Biomedicine, 93(2), 115–123
[9] Inza, I., Larrañaga, P., Blanco, R., & Cerrolaza, A. J. (2004). Filter versus wrapper gene selection approaches in DNA microarray domains. Artificial Intelligence in Medicine, 31(2), 91–103.
[10] Jolliffe, I. T. (2002). Principal Component Analysis, Second Edition. Encyclopedia of Statistics in Behavioral Science, 30(3), 487.
[11] Kohavi, R., & John, G. H. (1997). Wrappers for feature subset selection. Artificial Intelligence, 97(1–2), 273–324.
[12] Leach, M. (2012). Parallelising feature selection algorithms. University of Manchester.
[13] Lee, I.-H., Lushington, G. H., & Visvanathan, M. (2011). A filter-based feature selection approach for identifying potential biomarkers for lung cancer. Journal of Clinical Bioinformatics, 1(1), 11.
[14] Liu, H., Setiono, R., Science, C., & Ridge, K. (1995). Chi2: Feature Selection, 388–391.
[15] Novaković, J., Strbac, P., & Bulatović, D. (2011). Toward optimal feature selection using ranking methods and classification algorithms. Yugoslav Journal of Operations Research, 21(1), 119–135.
[16] Patil, T. R. (2013). Performance Analysis of Naive Bayes and J48 Classification Algorithm for Data Classification. International Journal Of Computer Science And Applications, ISSN: 0974-1011, 6(2), 256–261.
[17] Roslina, A. H., & Noraziah, A. (2010). Prediction of hepatitis prognosis using support vector machines and wrapper method. Proceedings - 2010 7th International Conference on Fuzzy Systems and Knowledge Discovery, FSKD 2010, 5(Fskd), 2209–2211.
[18] Sathyadevi, G. (2011). Application of CART algorithm in hepatitis disease diagnosis. International Conference on Recent Trends in Information Technology, ICRTIT 2011, 1283–1287.
[19] Witten, I. H., Frank, E., & Hall, M. a. (2011). Data Mining: Practical Machine Learning Tools and Techniques (Google eBook). Complementary literature None.
[20] Yasin, H. (2011). Hepatitis-C Classification using Data Mining Techniques, 24(3), 1–6.