Classification of Chronic Kidney Disease using Combination Feature Selection Techniques and Classifiers
A. K. Shrivas1 , Sanat Kumar Sahu2
Section:Research Paper, Product Type: Journal Paper
Volume-07 ,
Issue-03 , Page no. 114-117, Feb-2019
Online published on Feb 15, 2019
Copyright © A. K. Shrivas, Sanat Kumar Sahu . This is an open access article distributed under the Creative Commons Attribution License, which permits unrestricted use, distribution, and reproduction in any medium, provided the original work is properly cited.
View this paper at Google Scholar | DPI Digital Library
How to Cite this Paper
- IEEE Citation
- MLA Citation
- APA Citation
- BibTex Citation
- RIS Citation
IEEE Style Citation: A. K. Shrivas, Sanat Kumar Sahu, “Classification of Chronic Kidney Disease using Combination Feature Selection Techniques and Classifiers,” International Journal of Computer Sciences and Engineering, Vol.07, Issue.03, pp.114-117, 2019.
MLA Style Citation: A. K. Shrivas, Sanat Kumar Sahu "Classification of Chronic Kidney Disease using Combination Feature Selection Techniques and Classifiers." International Journal of Computer Sciences and Engineering 07.03 (2019): 114-117.
APA Style Citation: A. K. Shrivas, Sanat Kumar Sahu, (2019). Classification of Chronic Kidney Disease using Combination Feature Selection Techniques and Classifiers. International Journal of Computer Sciences and Engineering, 07(03), 114-117.
BibTex Style Citation:
@article{Shrivas_2019,
author = {A. K. Shrivas, Sanat Kumar Sahu},
title = {Classification of Chronic Kidney Disease using Combination Feature Selection Techniques and Classifiers},
journal = {International Journal of Computer Sciences and Engineering},
issue_date = {2 2019},
volume = {07},
Issue = {03},
month = {2},
year = {2019},
issn = {2347-2693},
pages = {114-117},
url = {https://www.ijcseonline.org/full_spl_paper_view.php?paper_id=689},
publisher = {IJCSE, Indore, INDIA},
}
RIS Style Citation:
TY - JOUR
UR - https://www.ijcseonline.org/full_spl_paper_view.php?paper_id=689
TI - Classification of Chronic Kidney Disease using Combination Feature Selection Techniques and Classifiers
T2 - International Journal of Computer Sciences and Engineering
AU - A. K. Shrivas, Sanat Kumar Sahu
PY - 2019
DA - 2019/02/15
PB - IJCSE, Indore, INDIA
SP - 114-117
IS - 03
VL - 07
SN - 2347-2693
ER -
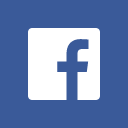
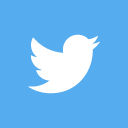
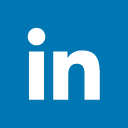
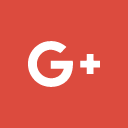
Abstract
The aim of the study is to predict significant features from dataset of Chronic Kidney Disease features. It represents the data in a tabular and graphical manner to form its clear understanding. This investigation helps in the crucial role of features and experimental features in the CKD dataset and their associations, their dependability for coming up with any classification system. It also shows that how CKD can be diagnosis by exploiting data mining techniques. The Data Mining algorithm is an inspirational force in detecting abnormalities in various data sets and with a good success utilized in various classification and feature selection task. The different kinds of Decision Tree-based classifiers like RF (Random Forest), J48 (C4.5), C5.0, and CART (Classification and Regression Tree) and their ensemble model have experimentally validated CKD dataset and our result is evaluated. Our result representation that the ensemble models classifier reached the most favourable performances on the identification of CKD dataset before and after the feature selection.
Key-Words / Index Term
Chronic Kidney Disease, Feature Selection Techniques, Classification, Random Forest, J48(C4.5), C5.0, CART, Ensemble model, Genetic Search, Greedy Stepwise
References
[1] M. P. Webster A.C., Nagler E.V., R.L., “Chronic Kideny disease,” 2016. [Online]. Available: http://www.worldkidneyday.org/faqs/chronic-kidney-disease/. [Accessed: 28-Feb-2018].
[2] M. S. McManus and S. Wynter-Minott, “Guidelines for Chronic Kidney Disease: Defining, Staging, and Managing in Primary Care,” J. Nurse Pract., vol. 13, no. 6, pp. 400–410, 2017.
[3] J. Han, M. Kamber, and J. Pei, Data mining: concepts and techniques, Third. Elsevier, 2012.
[4] I. H. Witten, E. Frank, and M. A. Hall, Data mining. 2011.
[5] A. Pujari, Data mining techniques, Third. University press, 2013.
[6] I. Guyon and A. Elisseeff, “An Introduction to Variable and Feature Selection,” J. Mach. Learn. Res., vol. 3, no. 3, pp. 1157–1182, 2003.
[7] A. Bhalla and R. K. Agrawal, “Microarray gene-expression data classification using less gene expressions by combining feature selection methods and classifiers,” Int. J. Inf. Eng. Electron. Bus., vol. 5, no. 5, pp. 42–48, 2013.
[8] A. K. Shrivas, S. K. Sahu, and H. S. Hota, “Classification of Chronic Kidney Disease with proposed Union Based Feature Selection Technique,” no. 2007, pp. 503–507, 2018.
[9] A. Subasi, E. Alickovic, and J. Kevric, “Diagnosis of Chronic Kidney Disease by Using Random Forest,” C. 2017 Proc. Int. Conf. Med. Biol. Eng. 2017, vol. 7, no. 1, pp. 589–594, 2017.
[10] B. Boukenze, A. Haqiq, and H. Mousannif, “Predicting Chronic Kidney Failure Disease Using Data Mining Techniques,” vol. 397, 2017.
[11] A. Charleonnan, T. Fufaung, T. Niyomwong, W. Chokchueypattanakit, S. Suwannawach, and N. Ninchawee, “Predictive analytics for chronic kidney disease using machine learning techniques,” 2016 Manag. Innov. Technol. Int. Conf., p. MIT-80-MIT-83, 2016.
[12] D. N. R. S.Ramya, “Diagnosis of Chronic Kidney Disease Using Machine Learning Algorithms,” Int. J. Innov. Res. Comput. Commun. Eng., vol. 4, no. 1, pp. 812–820, 2016.
[13] M. Arora and E. A. Sharma, “Chronic Kidney Disease Detection by Analyzing Medical Datasets in Weka,” Int. J. Comput. Appl., vol. 6, no. 4, pp. 20–26, 2016.
[14] M. Kumar, “Prediction of Chronic Kidney Disease Using Random Forest Machine Learning Algorithm,” Int. J. Comput. Sci. Mob. Comput., vol. 5, no. 2, pp. 24–33, 2016.
[15] R. Nagalla, P. Pothuganti, and D. S. Pawar, “Analyzing Gap Acceptance Behavior at Unsignalized Intersections Using Support Vector Machines, Decision Tree and Random Forests,” Procedia Comput. Sci., vol. 109, no. 2016, pp. 474–481, 2017.
[16] R. Parimala and R. Nallaswamy, “A Study of Spam E-mail classification using Feature Selection package,” Glob. J. Comput. Sci. Technol., vol. 11, no. 7, pp. 45–54, 2011.
[17] A. K. Shrivas, S. K. Sahu, and S. K. Singhai, “Decision support system for classification of chronic kidney disease with principle component analysis,” vol. 14, no. 2, pp. 105–110, 2017.
[18] Sivanandam and Deepa, Principles of Soft Computing, Second. wiley, 2014.
[19] S. Haykin, Neural Networks and Learning Machines, vol. 3. 2008.
[20] C. Arun Kumar, M. P. Sooraj, and S. Ramakrishnan, “A Comparative Performance Evaluation of Supervised Feature Selection Algorithms on Microarray Datasets,” Procedia Comput. Sci., vol. 115, pp. 209–217, 2017.
[21] D. E. Goldberg, “Genetic Algorithms in Search Optimization & Machine Learning.” p. 412, 1989.
[22] M. Hall, “Correlation-based Feature Selection for Machine Learning,” Methodology, vol. 21i195-i20, no. April, pp. 1–5, 1999.
[23] “UCI Machine Learning Repository of machine learning databases,” 2015. [Online]. Available: https://archive.ics.uci.edu/ml/datasets/Chronic_Kidney_Disease. [Accessed: 01-Jan-2016].